AUC Score :
Short-term Tactic1 :
Dominant Strategy :
Time series to forecast n:
ML Model Testing : Transfer Learning (ML)
Hypothesis Testing : Spearman Correlation
Surveillance : Major exchange and OTC
1Short-term revised.
2Time series is updated based on short-term trends.
Key Points
The SMI index is projected to experience moderate volatility in the near term. The prevailing trend suggests a consolidation phase, with potential for minor fluctuations. The market sentiment appears cautiously optimistic, although economic uncertainties could trigger corrections. Further, geopolitical events represent significant risk, potentially leading to increased downside pressure. Overall, the index is likely to trade within a defined range, with the possibility of breaking out on either side dependent on evolving circumstances.About SMI Index
The Swiss Market Index (SMI) serves as the benchmark for the Swiss stock market, reflecting the performance of the 20 largest and most liquid companies listed on the SIX Swiss Exchange. It's a capitalization-weighted index, meaning the companies with larger market capitalizations have a greater influence on the index's value. This weighting methodology provides a good representation of the overall health and direction of the Swiss equity market, making it a key indicator for investors seeking to understand the Swiss economy and investment landscape.
The SMI's composition is reviewed periodically to ensure its continued relevance and representativeness. This involves assessing the size and liquidity of the companies and adjusting the weighting as necessary. The index's focus on large, established companies contributes to a degree of stability, while also offering exposure to a range of industries prevalent in the Swiss economy, including pharmaceuticals, financial services, and luxury goods. As such, the SMI is a widely followed and important instrument for investors in both Switzerland and globally.
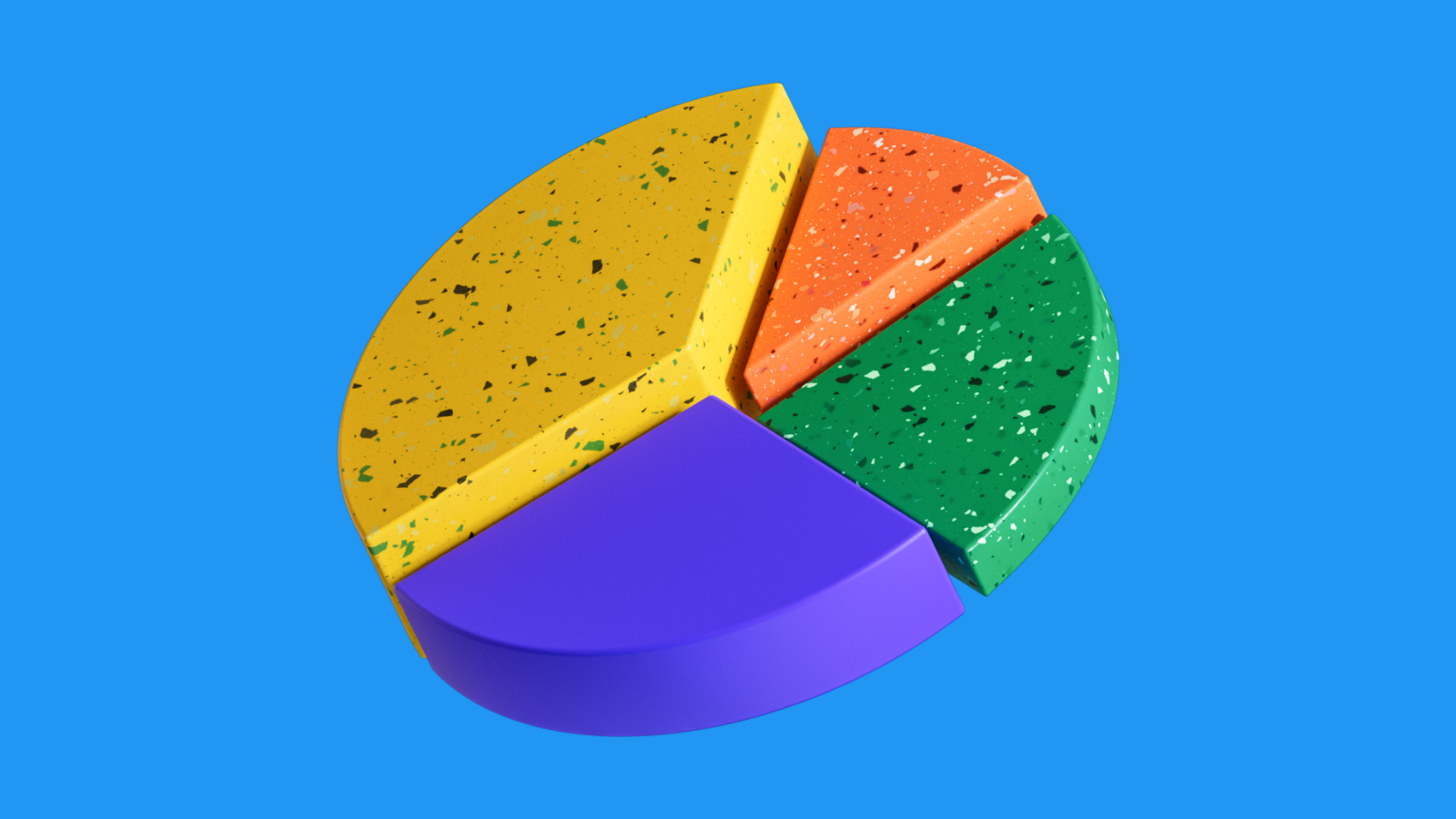
SMI Index Forecasting Model
Our team of data scientists and economists has developed a comprehensive machine learning model to forecast the Swiss Market Index (SMI). The core of our approach involves a blend of time series analysis and feature engineering to capture both the historical patterns and external influences on the index. We will leverage a diverse set of predictors, encompassing macroeconomic indicators such as GDP growth, inflation rates (CPI), and interest rate differentials, alongside market-specific variables like trading volume, volatility measures (VIX), and the performance of related European indices. Furthermore, the model will incorporate sentiment analysis derived from financial news articles and social media data to gauge investor sentiment, a critical factor in short-term market fluctuations. The aim is to create a robust and reliable forecasting tool by integrating diverse data sources and employing advanced techniques.
For the model's architecture, we've selected a combination of algorithms. Initially, we'll employ Recurrent Neural Networks (RNNs), specifically Long Short-Term Memory (LSTM) networks, due to their ability to effectively handle sequential data inherent in time series analysis. We will also integrate Gradient Boosting Machines (GBM) and Random Forest algorithms to capture non-linear relationships and interactions between features, increasing model accuracy. Feature selection will be conducted using techniques like correlation analysis, and statistical significance testing to identify the most informative predictors and prevent overfitting. The training process will involve splitting the historical data into training, validation, and testing sets. We'll conduct hyperparameter tuning using techniques like grid search and cross-validation to optimize model performance and generalization capabilities.
The model's performance will be meticulously evaluated using several metrics, including Mean Absolute Error (MAE), Root Mean Squared Error (RMSE), and the Mean Absolute Percentage Error (MAPE) to quantify the forecasting accuracy. We will also calculate the Directional Accuracy, measuring the proportion of times the model correctly predicts the direction of price movement. Our team will continuously monitor the model's performance, retraining and re-evaluating it regularly as new data become available to maintain its predictive capabilities. The model's output, including forecasted values and confidence intervals, will be regularly assessed to provide valuable insight to the decision-making process.
ML Model Testing
n:Time series to forecast
p:Price signals of SMI index
j:Nash equilibria (Neural Network)
k:Dominated move of SMI index holders
a:Best response for SMI target price
For further technical information as per how our model work we invite you to visit the article below:
How do KappaSignal algorithms actually work?
SMI Index Forecast Strategic Interaction Table
Strategic Interaction Table Legend:
X axis: *Likelihood% (The higher the percentage value, the more likely the event will occur.)
Y axis: *Potential Impact% (The higher the percentage value, the more likely the price will deviate.)
Z axis (Grey to Black): *Technical Analysis%
SMI Index Financial Outlook and Forecast
The Swiss Market Index (SMI), representing the performance of the 20 largest and most liquid companies listed on the SIX Swiss Exchange, exhibits a generally positive outlook, particularly in the medium term. The Swiss economy's inherent stability, fueled by a robust financial sector, pharmaceutical giants, and strong international trade relationships, forms a solid foundation. Furthermore, the SMI benefits from a currency that is widely considered a safe haven, attracting investment during periods of global economic uncertainty. This, in turn, can contribute to relative outperformance compared to other global indices. The index's composition, heavily weighted towards healthcare and financials, provides a degree of diversification and resilience, given the consistent demand for healthcare products and services and the generally conservative approach of Swiss financial institutions. This diversification and the underlying strength of the Swiss economy support a constructive view of the SMI's long-term prospects.
Several factors suggest continued positive momentum for the SMI. The strong performance of multinational corporations listed on the index, such as Roche, Novartis, and Nestlé, is a significant driver. Their global reach and ability to generate revenue in diverse markets, combined with their focus on innovation and research and development, provide significant growth potential. Additionally, the financial sector, with key players like UBS and Credit Suisse (now part of UBS Group) contributing substantially to the index's value, demonstrates resilience and the ability to adapt to evolving market conditions. While the sector faces its own challenges, the Swiss financial institutions' focus on wealth management and global financial services, along with robust capital buffers, offers protection. Further aiding the index's positive outlook are the Swiss franc's inherent stability and the attractive dividend yields offered by many of the SMI components, providing investors with a stream of income and potential capital appreciation.
However, the SMI's performance is not without its challenges. The index's concentration, with a significant weighting in healthcare and financial sectors, creates a vulnerability to any downturn in these areas. Changes in global regulations impacting pharmaceutical pricing or adverse developments affecting the financial sector can weigh on the index. The strength of the Swiss franc, while a safe haven asset, can also negatively affect the earnings of Swiss companies with significant foreign operations, as their revenues translate to fewer Swiss francs. Furthermore, the Swiss economy's reliance on international trade exposes it to global economic slowdowns or protectionist measures. Inflationary pressures, although less severe than in other regions, and any resulting monetary policy adjustments by the Swiss National Bank could also impact the cost of capital and overall economic activity, influencing the performance of the SMI.
Considering the current environment, the SMI is expected to demonstrate moderate gains over the next year. The strengths of the underlying economy, the diversification benefits within the index, and the attractive dividend yields of many constituent companies are the key factors behind this projection. The primary risk to this positive outlook is a sharper-than-expected global economic slowdown, leading to a decline in demand for Swiss exports and negatively impacting the profitability of SMI-listed companies. Geopolitical instability, rising interest rates, or any substantial disruption to the financial sector also pose threats. However, the robust fundamentals of the Swiss economy and the conservative nature of Swiss businesses mitigate some of these risks, leading to a relatively optimistic forecast for the index, albeit with inherent uncertainties.
Rating | Short-Term | Long-Term Senior |
---|---|---|
Outlook | B2 | B1 |
Income Statement | Caa2 | Caa2 |
Balance Sheet | Baa2 | Baa2 |
Leverage Ratios | C | Caa2 |
Cash Flow | Caa2 | Baa2 |
Rates of Return and Profitability | B1 | B2 |
*An aggregate rating for an index summarizes the overall sentiment towards the companies it includes. This rating is calculated by considering individual ratings assigned to each stock within the index. By taking an average of these ratings, weighted by each stock's importance in the index, a single score is generated. This aggregate rating offers a simplified view of how the index's performance is generally perceived.
How does neural network examine financial reports and understand financial state of the company?
References
- Bierens HJ. 1987. Kernel estimators of regression functions. In Advances in Econometrics: Fifth World Congress, Vol. 1, ed. TF Bewley, pp. 99–144. Cambridge, UK: Cambridge Univ. Press
- D. Bertsekas. Nonlinear programming. Athena Scientific, 1999.
- Jacobs B, Donkers B, Fok D. 2014. Product Recommendations Based on Latent Purchase Motivations. Rotterdam, Neth.: ERIM
- D. S. Bernstein, S. Zilberstein, and N. Immerman. The complexity of decentralized control of Markov Decision Processes. In UAI '00: Proceedings of the 16th Conference in Uncertainty in Artificial Intelligence, Stanford University, Stanford, California, USA, June 30 - July 3, 2000, pages 32–37, 2000.
- Li L, Chen S, Kleban J, Gupta A. 2014. Counterfactual estimation and optimization of click metrics for search engines: a case study. In Proceedings of the 24th International Conference on the World Wide Web, pp. 929–34. New York: ACM
- Kitagawa T, Tetenov A. 2015. Who should be treated? Empirical welfare maximization methods for treatment choice. Tech. Rep., Cent. Microdata Methods Pract., Inst. Fiscal Stud., London
- Mazumder R, Hastie T, Tibshirani R. 2010. Spectral regularization algorithms for learning large incomplete matrices. J. Mach. Learn. Res. 11:2287–322