AUC Score :
Short-term Tactic1 :
Dominant Strategy :
Time series to forecast n:
ML Model Testing : Active Learning (ML)
Hypothesis Testing : Pearson Correlation
Surveillance : Major exchange and OTC
1Short-term revised.
2Time series is updated based on short-term trends.
Key Points
The Dow Jones U.S. Semiconductors index is anticipated to experience moderate growth, fueled by ongoing demand in areas like artificial intelligence, automotive electronics, and cloud computing. This growth will likely be tempered by potential headwinds, including global economic uncertainties, supply chain disruptions, and rising interest rates, which could increase borrowing costs for companies and impact consumer spending on technology products. Further, intense competition within the industry, alongside regulatory scrutiny related to antitrust concerns, pose additional risks that could hinder growth. However, technological advancements and innovation in chip design are expected to provide some offset to the downward pressures, ensuring continued expansion in this sector.About Dow Jones U.S. Semiconductors Index
The Dow Jones U.S. Semiconductors Index tracks the performance of U.S.-listed companies involved in the design, manufacture, and sale of semiconductors and related equipment. This sector-specific index serves as a benchmark for investors seeking exposure to the semiconductor industry, reflecting its growth and technological advancements. It typically includes a range of companies, from chipmakers to manufacturers of semiconductor equipment, providing a broad representation of the entire value chain. This index is often used by analysts, fund managers, and investors as a tool for evaluating the sector's overall health, identifying investment opportunities, and comparing individual company performances.
The index's composition and weighting methodologies may vary, but generally, it employs market capitalization to determine the influence each constituent has on the overall index movement. Rebalancing occurs periodically, adjusting the index to reflect changes in market dynamics, corporate events, and the evolving landscape of the semiconductor industry. Because of its focus, investors often use it as a gauge of technological innovation and its financial effects, offering insight into the industry's cyclical nature influenced by global demand and technological disruptions.
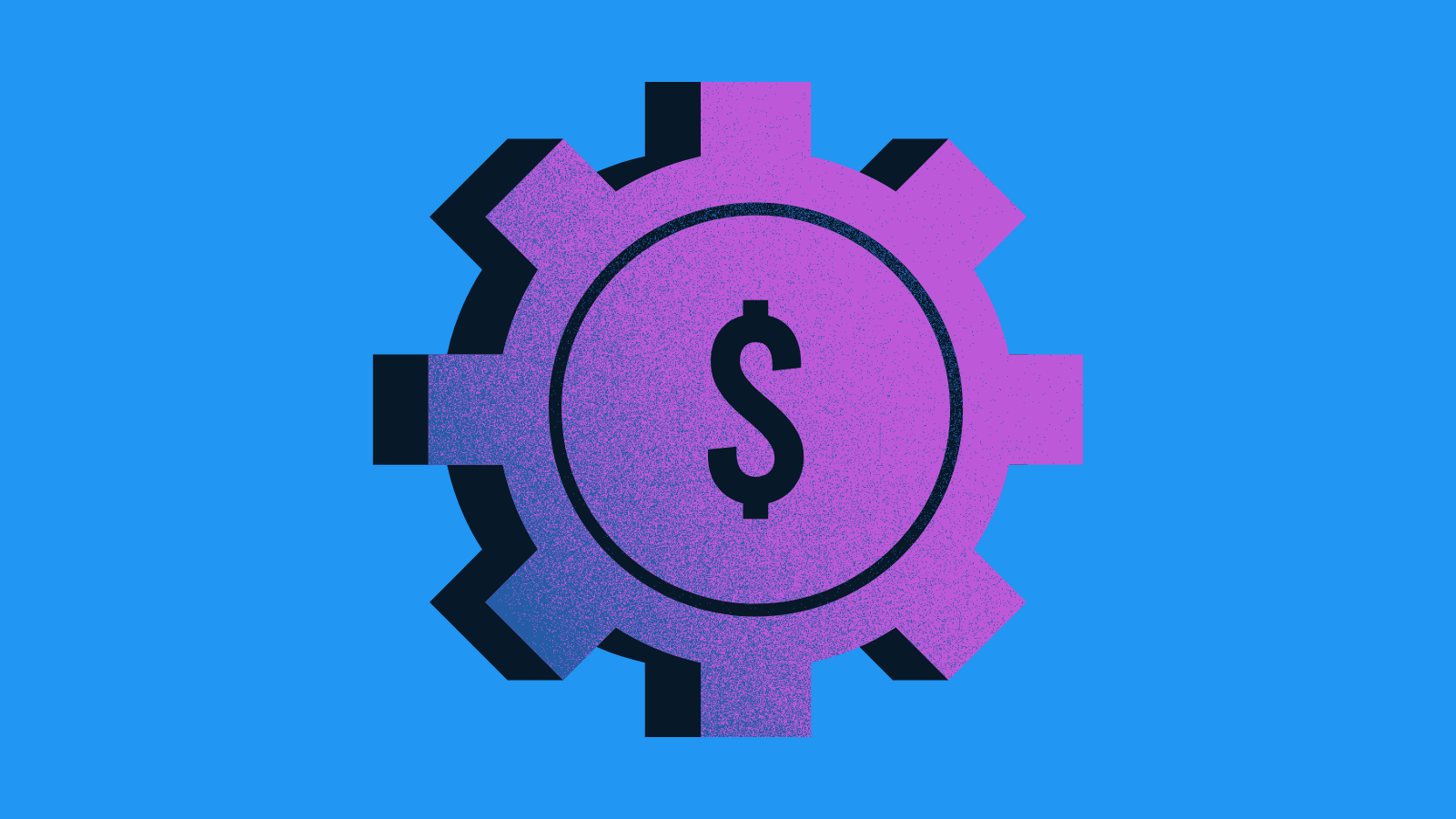
Dow Jones U.S. Semiconductors Index Forecasting Machine Learning Model
Our team of data scientists and economists proposes a comprehensive machine learning model for forecasting the Dow Jones U.S. Semiconductors Index. The model will leverage a diverse set of features categorized into fundamental, technical, and macroeconomic indicators. Fundamental features will include company-specific financial metrics such as revenue, earnings per share (EPS), debt-to-equity ratios, and profit margins for the major companies within the index. Technical features will incorporate historical price data, including moving averages (MA), Relative Strength Index (RSI), and trading volume. Macroeconomic variables, encompassing factors that significantly affect the semiconductor sector's profitability, will be added. These include interest rates, inflation rates, consumer spending figures, industrial production indices, and global semiconductor sales data. The model will be trained on a substantial historical dataset, covering at least the past ten years of relevant data, to capture cyclical trends and provide robust forecasting capabilities.
The core of our model will be a hybrid approach combining several machine learning algorithms. We intend to experiment with Recurrent Neural Networks (RNNs), specifically Long Short-Term Memory (LSTM) networks, which are well-suited for time-series data and capturing long-term dependencies within the index. These will be complemented by Gradient Boosting Machines (GBM), such as XGBoost or LightGBM, known for their robust performance and ability to handle a diverse range of features. Additionally, we will investigate the utility of Support Vector Machines (SVM) for classification or regression tasks. Feature engineering will play a critical role, including the creation of lagged variables, interaction terms, and volatility measures, to optimize model accuracy. Model performance will be rigorously evaluated using metrics like Mean Squared Error (MSE), Root Mean Squared Error (RMSE), and Mean Absolute Percentage Error (MAPE) on a holdout dataset, to ensure the model generalizes effectively to unseen data.
The model's output will be a probabilistic forecast, providing both point estimates and confidence intervals for the index's future values over different time horizons (e.g., one week, one month, and one quarter). Model interpretability will be emphasized through techniques such as SHAP values to identify the most influential features driving the forecasts, facilitating a better understanding of the model's predictions. This enhanced understanding will empower stakeholders with actionable insights. Moreover, we plan to continuously monitor and retrain the model with fresh data to maintain its accuracy and incorporate new information, and to stay ahead of changes in the market. A comprehensive risk management strategy will be implemented to account for market volatility.
ML Model Testing
n:Time series to forecast
p:Price signals of Dow Jones U.S. Semiconductors index
j:Nash equilibria (Neural Network)
k:Dominated move of Dow Jones U.S. Semiconductors index holders
a:Best response for Dow Jones U.S. Semiconductors target price
For further technical information as per how our model work we invite you to visit the article below:
How do KappaSignal algorithms actually work?
Dow Jones U.S. Semiconductors Index Forecast Strategic Interaction Table
Strategic Interaction Table Legend:
X axis: *Likelihood% (The higher the percentage value, the more likely the event will occur.)
Y axis: *Potential Impact% (The higher the percentage value, the more likely the price will deviate.)
Z axis (Grey to Black): *Technical Analysis%
Dow Jones U.S. Semiconductors Index: Financial Outlook and Forecast
The outlook for the Dow Jones U.S. Semiconductors Index remains robust, underpinned by several key factors. Firstly, the ongoing global demand for semiconductors, driven by the proliferation of artificial intelligence (AI), 5G technology, and the Internet of Things (IoT), will continue to fuel growth. These technologies require increasingly sophisticated and powerful chips, creating substantial opportunities for companies within the index. Secondly, government initiatives aimed at bolstering domestic semiconductor manufacturing, particularly in the United States, are expected to provide significant tailwinds. These initiatives often involve substantial financial incentives and infrastructure development, supporting expansion and innovation within the sector. Thirdly, the long-term secular trends of digitalization and automation across various industries will persistently drive demand for semiconductor components. From automotive to healthcare, the dependence on semiconductors is expanding, creating sustained revenue streams for index constituents. Furthermore, technological advancements, such as the miniaturization of transistors and the development of new materials, contribute to ongoing improvements in chip performance and efficiency, making them essential for future technologies.
The financial performance of companies within the Dow Jones U.S. Semiconductors Index is also expected to be strengthened by several operational dynamics. Supply chain disruptions, a major concern in the past, are gradually easing, which should lead to improved production and profitability. Companies are also investing heavily in research and development (R&D), further improving product cycles. These initiatives are focused on developing the next generation of chips, including specialized processors for AI applications. Additionally, strategic acquisitions and mergers within the industry are leading to increased market consolidation and efficiency. The industry is constantly adapting to technological advancements and evolving end-market demands. Furthermore, effective cost management and operational efficiency are vital, allowing for more significant profit margins and improved returns on investment. The competitive landscape is highly dynamic, with companies constantly competing for market share and technological leadership. Therefore, maintaining high levels of operational efficiency and technological innovation is crucial for long-term success.
The expected growth in the industry, however, could be tempered by certain macro-economic and geopolitical factors. The global economy, particularly any slowdown in economic activity, could reduce demand for electronics and, consequently, semiconductors. Geopolitical tensions, including trade wars and export restrictions, can disrupt supply chains and limit market access for companies within the index. Furthermore, any abrupt changes in interest rates by central banks could increase borrowing costs for companies. A surge in raw material prices or labor costs could diminish profit margins. Moreover, the rapid pace of technological change means that companies must continuously invest in R&D to stay relevant, which can be expensive and carries inherent risks. Other concerns include the cyclical nature of the semiconductor industry, with periods of high demand followed by periods of oversupply. Finally, any major disruptions to the supply of critical materials, like rare earth minerals, could severely impact semiconductor production.
In conclusion, the Dow Jones U.S. Semiconductors Index is likely to experience continued growth over the next several years, driven by sustained demand and technological advancement. Therefore, the prediction is positive. However, the pace of growth could be moderated by economic volatility, geopolitical risks, and industry-specific challenges. The main risks to this positive outlook include a deeper-than-expected global economic downturn, intensified geopolitical tensions, and significant disruptions to supply chains. Successful navigation of these risks, along with continued innovation and effective cost management, will be key to maintaining strong financial performance for companies within the index. Investors should closely monitor macro-economic developments, geopolitical events, and industry-specific trends when assessing the performance of this sector.
Rating | Short-Term | Long-Term Senior |
---|---|---|
Outlook | Ba2 | B1 |
Income Statement | Baa2 | Caa2 |
Balance Sheet | B1 | B3 |
Leverage Ratios | Ba1 | B1 |
Cash Flow | Caa2 | Baa2 |
Rates of Return and Profitability | Baa2 | Caa2 |
*An aggregate rating for an index summarizes the overall sentiment towards the companies it includes. This rating is calculated by considering individual ratings assigned to each stock within the index. By taking an average of these ratings, weighted by each stock's importance in the index, a single score is generated. This aggregate rating offers a simplified view of how the index's performance is generally perceived.
How does neural network examine financial reports and understand financial state of the company?
References
- Burgess, D. F. (1975), "Duality theory and pitfalls in the specification of technologies," Journal of Econometrics, 3, 105–121.
- R. Sutton and A. Barto. Reinforcement Learning. The MIT Press, 1998
- Bessler, D. A. T. Covey (1991), "Cointegration: Some results on U.S. cattle prices," Journal of Futures Markets, 11, 461–474.
- Mazumder R, Hastie T, Tibshirani R. 2010. Spectral regularization algorithms for learning large incomplete matrices. J. Mach. Learn. Res. 11:2287–322
- K. Tuyls and G. Weiss. Multiagent learning: Basics, challenges, and prospects. AI Magazine, 33(3): 41–52, 2012
- Ruiz FJ, Athey S, Blei DM. 2017. SHOPPER: a probabilistic model of consumer choice with substitutes and complements. arXiv:1711.03560 [stat.ML]
- V. Borkar. Q-learning for risk-sensitive control. Mathematics of Operations Research, 27:294–311, 2002.