AUC Score :
Short-term Tactic1 :
Dominant Strategy :
Time series to forecast n:
ML Model Testing : Modular Neural Network (Speculative Sentiment Analysis)
Hypothesis Testing : Multiple Regression
Surveillance : Major exchange and OTC
1Short-term revised.
2Time series is updated based on short-term trends.
Key Points
S&P Global's future appears promising, predicated on its strong market position in ratings, indices, and analytics. The company is expected to benefit from increasing demand for data and insights across financial markets and various industries. Expansion into emerging markets and strategic acquisitions are anticipated to contribute to revenue growth. However, potential risks include economic downturns impacting credit ratings demand, regulatory scrutiny, and heightened competition from other financial information providers. Furthermore, the company's growth trajectory could be affected by the integration of acquired businesses and shifts in financial market dynamics.About S&P Global
S&P Global is a prominent financial information and analytics company. It operates through four main divisions: S&P Global Ratings, which provides credit ratings, research, and analysis; S&P Global Market Intelligence, which delivers data, research, and insights on various markets; S&P Global Commodity Insights, focusing on energy and commodity markets; and S&P Global Mobility, providing automotive intelligence and forecasting.
The company serves a diverse customer base including financial institutions, corporations, governments, and educational institutions. Its products and services are essential for investment decisions, risk management, and strategic planning across multiple industries. S&P Global is headquartered in New York City and has a global presence, with offices and operations worldwide.
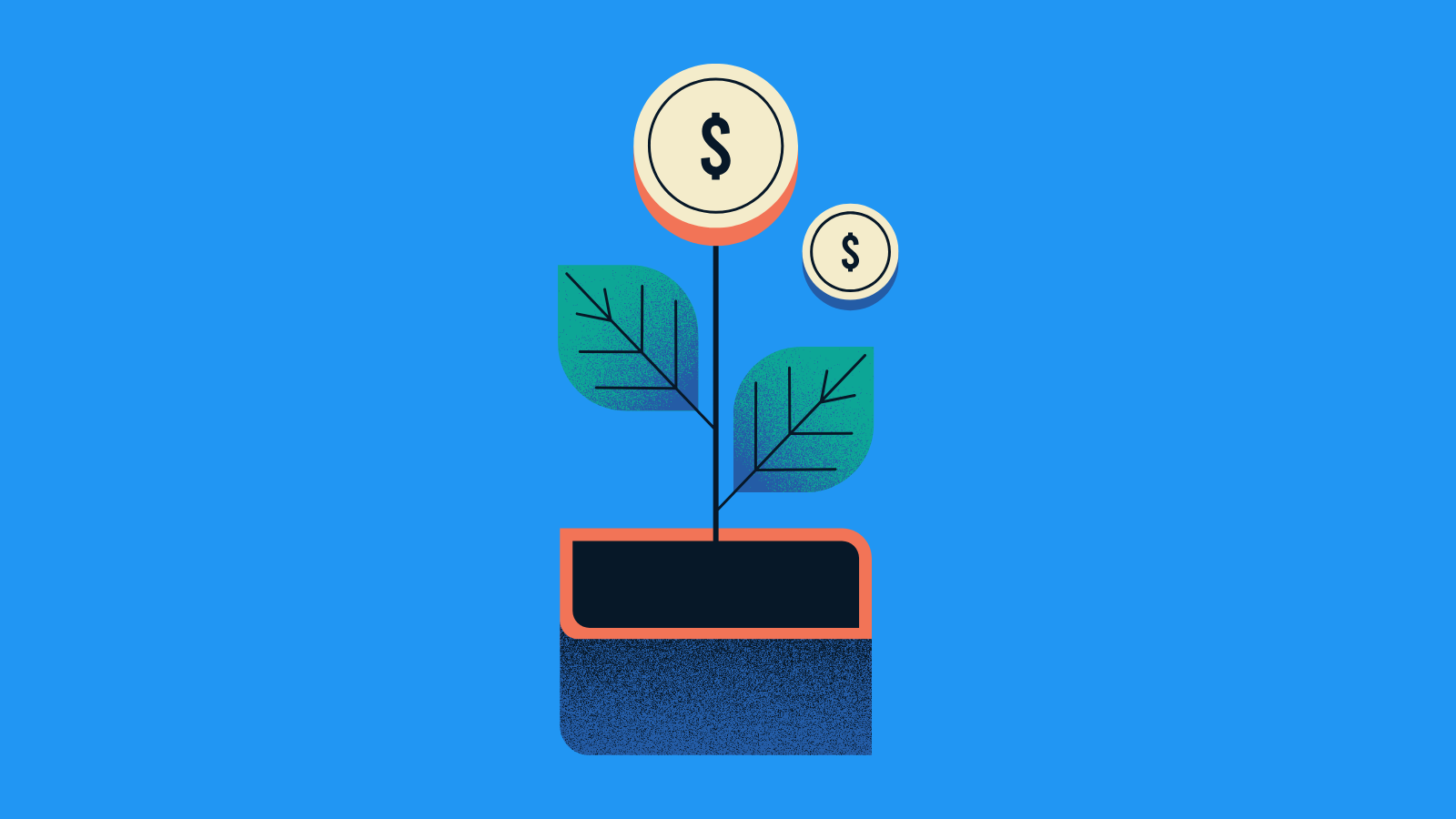
SPGI Stock Forecast Model
The forecasting model for S&P Global Inc. (SPGI) stock leverages a multifaceted approach, incorporating both fundamental and technical analysis, underpinned by machine learning algorithms. Fundamental factors considered include revenue growth, profit margins, debt levels, and market capitalization, reflecting the company's financial health and competitive positioning. These factors are sourced from publicly available financial statements, analyst reports, and industry publications. Technical indicators, such as moving averages, Relative Strength Index (RSI), and volume data, are integrated to capture short-term trends and market sentiment. A comprehensive dataset is constructed by compiling historical data from these diverse sources. We emphasize data cleaning and preprocessing to address missing values and standardize data formats, which is crucial for the model's accuracy and robustness.
The core of the model employs a hybrid machine learning architecture. Initially, a set of features will be selected using feature importance algorithms, reducing the noise and enhancing model interpretability. Then, we will train a combination of models: a Gradient Boosting Regressor for capturing complex non-linear relationships in the data, and a Long Short-Term Memory (LSTM) network, well-suited for time series forecasting. Each model will be trained on the historical data, and its performance will be evaluated using metrics such as Mean Squared Error (MSE), and R-squared on a separate validation dataset. This ensures the model's capability to generalize and to forecast unseen trends. The final forecast will be generated by combining the predictions from these models, utilizing an ensemble technique to enhance the accuracy of the forecasts.
The model's forecasts are periodically updated and backtested to incorporate the newest available data, market trends, and economic shifts. Furthermore, the results are evaluated against benchmark models and expert forecasts for validation and improvement. Risk management is a key component of the model's deployment, with considerations for factors such as data uncertainties, model limitations, and sensitivity analyses. The model's outputs are presented in a clear, easy-to-understand format, including the forecasted direction, predicted magnitude of change, and confidence intervals. These results will support investment decision-making and offer valuable market insights for SPGI investors and stakeholders.
ML Model Testing
n:Time series to forecast
p:Price signals of S&P Global stock
j:Nash equilibria (Neural Network)
k:Dominated move of S&P Global stock holders
a:Best response for S&P Global target price
For further technical information as per how our model work we invite you to visit the article below:
How do KappaSignal algorithms actually work?
S&P Global Stock Forecast (Buy or Sell) Strategic Interaction Table
Strategic Interaction Table Legend:
X axis: *Likelihood% (The higher the percentage value, the more likely the event will occur.)
Y axis: *Potential Impact% (The higher the percentage value, the more likely the price will deviate.)
Z axis (Grey to Black): *Technical Analysis%
S&P Global Inc. Financial Outlook and Forecast
The financial outlook for S&P Global (SPGI) appears robust, supported by its diversified business model and strong market positions in key sectors. SPGI's primary revenue streams include ratings, market intelligence, and indices, each of which benefits from long-term structural tailwinds. The company's ratings business, Standard & Poor's, is a critical component of global financial markets, providing assessments that influence debt issuances and investment decisions. Its Market Intelligence division, encompassing data and analytics, is poised to benefit from the increasing demand for information in financial markets and associated areas. Moreover, the indices business, including the S&P 500, generates recurring revenue tied to assets under management. The company's strategic initiatives, such as its acquisition of IHS Markit, have significantly expanded its data and analytics capabilities, bolstering its competitive advantage and long-term growth prospects. This expansion strategically positions SPGI to capitalize on evolving market needs and reinforces its leadership within the financial information industry.
Future growth will be fueled by several factors. The ongoing growth of global financial markets, including the rise of emerging markets, creates a larger addressable market for SPGI's services. The increasing complexity of financial instruments and regulatory requirements necessitates the use of sophisticated data, analytics, and ratings, driving demand for SPGI's products. Furthermore, the company is actively investing in technological advancements, including data analytics and artificial intelligence, to enhance its product offerings and improve operational efficiency. This commitment to innovation enables the company to remain competitive and meet the evolving needs of its clients. The integration of IHS Markit is anticipated to deliver significant synergy benefits, improving profitability and driving revenue growth. SPGI's recurring revenue model, with substantial subscription-based revenue, offers stability and predictability in financial results. This will allow for strategic long-term investment and the pursuit of future development.
S&P Global's financial performance is expected to be positive in the medium to long term, with revenue growth driven by increasing market participation in key markets and continued growth in the data and analytics segments. The company's operational efficiency improvements and cost management strategies should support margin expansion, leading to strong profit growth. Continued growth in the index business, with stable inflows, will strengthen its profitability. The strategic acquisitions and internal investments are projected to contribute to long-term value creation for shareholders. The company's balance sheet strength and disciplined capital allocation strategies provide flexibility to manage challenges and pursue strategic opportunities. The company will continue to manage its portfolio and focus on strategic initiatives that will lead to the long-term success of the firm. Management's strong track record of execution adds confidence to the positive outlook.
The forecast for S&P Global is positive, with continued growth expected across its various business segments. This prediction is based on the company's strategic advantages, market position, and operational execution. However, there are potential risks. These include the risk of an economic downturn, which could reduce debt issuance and investment activity, affecting the ratings business. The evolving regulatory landscape and the potential for increased competition in the data and analytics markets are risks that SPGI needs to manage. Another risk would be geopolitical instability which could affect global financial markets. Despite these risks, SPGI's diversified revenue streams, strategic initiatives, and commitment to innovation position it favorably to achieve its long-term financial goals and deliver shareholder value.
Rating | Short-Term | Long-Term Senior |
---|---|---|
Outlook | B1 | B1 |
Income Statement | Baa2 | Ba1 |
Balance Sheet | B1 | C |
Leverage Ratios | C | C |
Cash Flow | B2 | Baa2 |
Rates of Return and Profitability | Ba3 | Baa2 |
*Financial analysis is the process of evaluating a company's financial performance and position by neural network. It involves reviewing the company's financial statements, including the balance sheet, income statement, and cash flow statement, as well as other financial reports and documents.
How does neural network examine financial reports and understand financial state of the company?
References
- Bottou L. 1998. Online learning and stochastic approximations. In On-Line Learning in Neural Networks, ed. D Saad, pp. 9–42. New York: ACM
- R. Sutton and A. Barto. Introduction to reinforcement learning. MIT Press, 1998
- Jorgenson, D.W., Weitzman, M.L., ZXhang, Y.X., Haxo, Y.M. and Mat, Y.X., 2023. Can Neural Networks Predict Stock Market?. AC Investment Research Journal, 220(44).
- Krizhevsky A, Sutskever I, Hinton GE. 2012. Imagenet classification with deep convolutional neural networks. In Advances in Neural Information Processing Systems, Vol. 25, ed. Z Ghahramani, M Welling, C Cortes, ND Lawrence, KQ Weinberger, pp. 1097–105. San Diego, CA: Neural Inf. Process. Syst. Found.
- Bertsimas D, King A, Mazumder R. 2016. Best subset selection via a modern optimization lens. Ann. Stat. 44:813–52
- Jorgenson, D.W., Weitzman, M.L., ZXhang, Y.X., Haxo, Y.M. and Mat, Y.X., 2023. Tesla Stock: Hold for Now, But Watch for Opportunities. AC Investment Research Journal, 220(44).
- Semenova V, Goldman M, Chernozhukov V, Taddy M. 2018. Orthogonal ML for demand estimation: high dimensional causal inference in dynamic panels. arXiv:1712.09988 [stat.ML]