AUC Score :
Short-term Tactic1 :
Dominant Strategy :
Time series to forecast n:
ML Model Testing : Modular Neural Network (Market News Sentiment Analysis)
Hypothesis Testing : Linear Regression
Surveillance : Major exchange and OTC
1Short-term revised.
2Time series is updated based on short-term trends.
Key Points
The S&P Bitcoin index is predicted to experience substantial volatility. The primary prediction is a continued, albeit potentially slower, upward trajectory, fueled by increased institutional adoption and mainstream acceptance, alongside developments in cryptocurrency regulations. However, the index faces significant risks, including heightened regulatory scrutiny from various global bodies that could stifle growth. Market sentiment is also a key factor, as significant price corrections could be triggered by negative news or shifts in investor confidence. Extreme price swings are expected due to the volatile nature of digital assets, which is exacerbated by global economic uncertainty. Furthermore, the index's performance is vulnerable to technological advancements and disruptions, as well as cyber security threats.About S&P Bitcoin Index
The S&P Bitcoin Index, a product of S&P Dow Jones Indices, aims to provide a benchmark for the performance of the Bitcoin market. It seeks to reflect the price movements of Bitcoin in a transparent and rules-based manner, offering investors a standardized way to track the digital currency's value. The index is designed to be replicable, allowing for the potential creation of financial products, such as exchange-traded funds (ETFs), that track its performance.
The methodology of the S&P Bitcoin Index typically involves selecting and weighting Bitcoin based on established criteria, possibly considering factors such as liquidity and trading volume. It adheres to S&P's rigorous index governance framework, ensuring the index's integrity and reliability. This index provides a valuable tool for market participants, enabling them to monitor Bitcoin's performance and compare it against other traditional and digital assets.
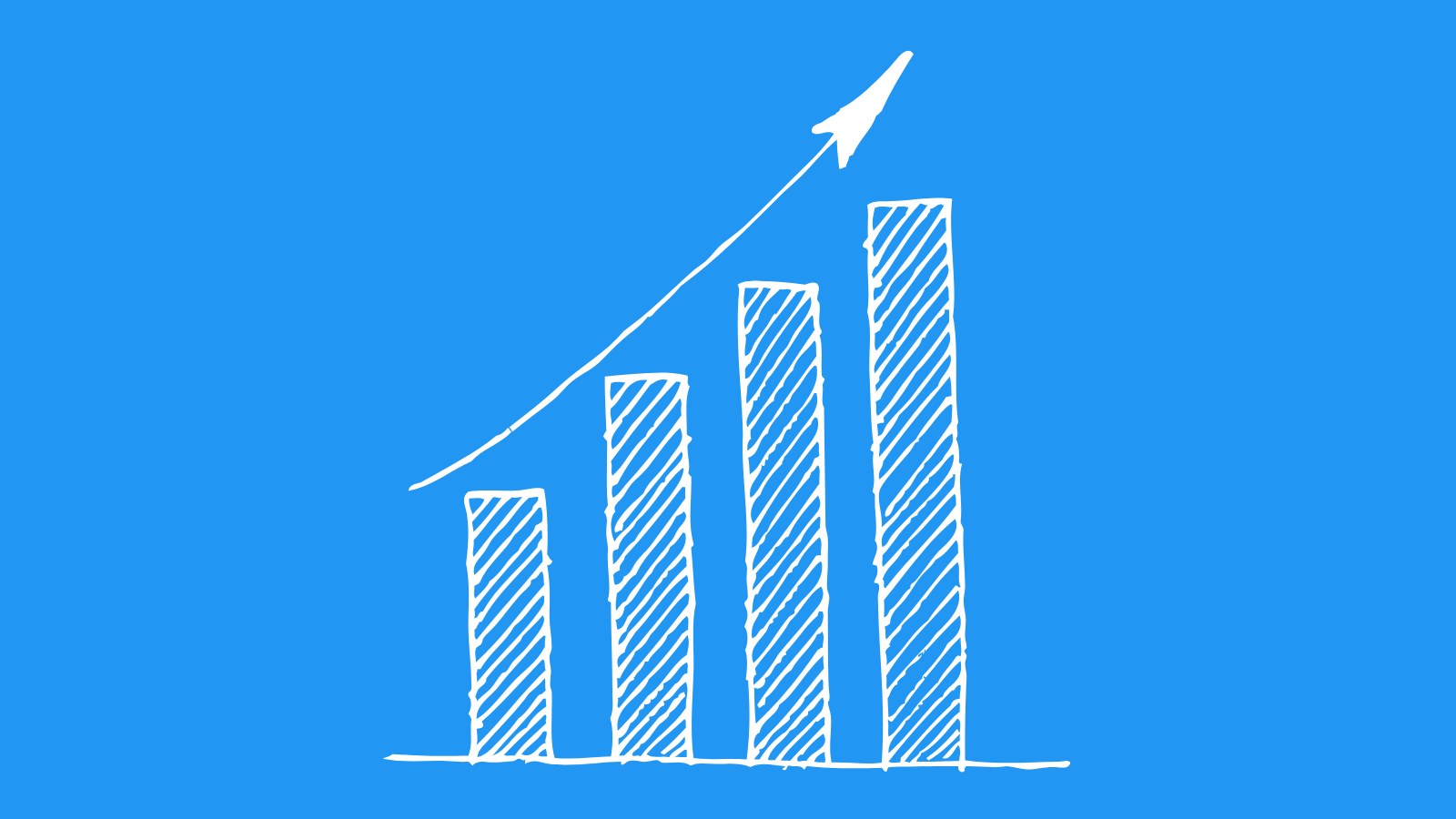
S&P Bitcoin Index Forecasting Model
The development of a robust forecasting model for the S&P Bitcoin Index necessitates a multifaceted approach incorporating both time series analysis and economic indicators. Our model utilizes a hybrid architecture, combining the strengths of Recurrent Neural Networks (RNNs), specifically Long Short-Term Memory (LSTM) networks, with traditional econometric methodologies. The LSTM networks will effectively capture the non-linear dependencies and temporal dynamics inherent in the Bitcoin market, addressing the volatility and noise that often plague financial time series. Economic data, selected through rigorous feature engineering and feature selection techniques, will be integrated to account for external influences. This dataset includes, but is not limited to, global macroeconomic indicators (GDP growth, inflation rates, interest rates), cryptocurrency market data (Bitcoin trading volume, market capitalization, exchange rates), and sentiment analysis derived from news articles and social media platforms. Data pre-processing, including standardization, normalization, and handling missing values, is a crucial step to ensure optimal model performance. The model will be trained on historical index data and relevant economic variables, optimizing for accuracy and efficiency.
Feature engineering is a key component of our model's success. We construct a variety of lagged variables from both the index itself and the economic indicators to capture historical patterns and dependencies. Furthermore, we apply technical indicators commonly used in financial analysis, such as moving averages, relative strength index (RSI), and Bollinger Bands, to enhance the model's ability to identify trends, volatility, and overbought/oversold conditions. Economic data indicators are carefully transformed and aggregated to provide a representative signal. For example, sentiment scores are created by processing textual data from news articles, assessing the overall sentiment surrounding Bitcoin. Statistical tests such as the Augmented Dickey-Fuller (ADF) test and the Kwiatkowski-Phillips-Schmidt-Shin (KPSS) test are used to examine the stationarity of time series, a fundamental requirement for many time series analysis techniques. Hyperparameter tuning through cross-validation is conducted to optimize the model's predictive capabilities, including the number of LSTM layers, the size of the hidden units, and the learning rate. The model is trained for a period of time to avoid overfitting.
Model evaluation is conducted using a variety of metrics to ensure a comprehensive assessment of its performance. Metrics such as Mean Absolute Error (MAE), Mean Squared Error (MSE), Root Mean Squared Error (RMSE), and R-squared are employed to quantify the accuracy of the predictions. Furthermore, we incorporate metrics such as directional accuracy, which evaluates the model's ability to correctly predict the direction of price movements, a critical factor for practical application. The robustness of the model is assessed through out-of-sample testing and backtesting, simulating real-world trading scenarios. A rigorous sensitivity analysis is conducted to understand the influence of each input variable on the forecast, providing valuable insights into the drivers of index movements. The model's output will be provided as a probability distribution to convey the model's uncertainty.
ML Model Testing
n:Time series to forecast
p:Price signals of S&P Bitcoin index
j:Nash equilibria (Neural Network)
k:Dominated move of S&P Bitcoin index holders
a:Best response for S&P Bitcoin target price
For further technical information as per how our model work we invite you to visit the article below:
How do KappaSignal algorithms actually work?
S&P Bitcoin Index Forecast Strategic Interaction Table
Strategic Interaction Table Legend:
X axis: *Likelihood% (The higher the percentage value, the more likely the event will occur.)
Y axis: *Potential Impact% (The higher the percentage value, the more likely the price will deviate.)
Z axis (Grey to Black): *Technical Analysis%
S&P Bitcoin Index: Financial Outlook and Forecast
The S&P Bitcoin Index, designed to track the performance of Bitcoin, presents a unique and evolving financial outlook. The index's value is intrinsically tied to the cryptocurrency market's overall health and sentiment, offering exposure to Bitcoin's price fluctuations. The index's financial outlook is characterized by high volatility, reflecting the inherent uncertainty within the cryptocurrency space. Institutional adoption, regulatory developments, and macroeconomic factors significantly influence this outlook. Increased institutional participation, driven by the index's ability to offer a standardized and regulated access point to Bitcoin, can boost market capitalization and potentially lead to upward price movements. Conversely, any unfavorable regulatory actions, such as increased scrutiny or outright bans in key jurisdictions, would likely trigger a downturn. The impact of these factors is further amplified by the limited liquidity compared to traditional asset classes.
Forecasting the S&P Bitcoin Index requires considering several market dynamics. Demand and supply factors remain pivotal; increased demand, whether from individual or institutional investors, tends to fuel price appreciation. Moreover, supply constraints, inherent in Bitcoin's limited total supply, contribute to a potential "scarcity premium." Macroeconomic trends, including inflation and interest rate policies, play a crucial role. Some investors perceive Bitcoin as a hedge against inflation, potentially driving demand during inflationary periods. Technological advancements within the Bitcoin ecosystem and broader blockchain space, such as scalability improvements and the development of layer-2 solutions, can positively impact the index by increasing the utility and efficiency of Bitcoin transactions. Furthermore, the ongoing evolution of the crypto infrastructure, including the emergence of regulated exchanges and custodial services, is a key indicator of maturity and potential growth for the underlying asset.
Analyzing the financial outlook of the S&P Bitcoin Index requires evaluating specific factors. Market sentiment, often reflected in social media trends and news cycles, influences short-term price volatility. News regarding security breaches, scams, or regulatory crackdowns can significantly impact investor confidence and lead to abrupt market corrections. The impact of these factors are also highly dependent on the economic trends. The interest rate decisions are key. Higher rates increase the costs of holding the asset, while lower rates facilitate access to capital. Global adoption rates across different investor segments are a strong indicator of long-term viability and potential for sustainable growth, as are the dynamics of competing cryptocurrencies and alternative investment avenues. The index's performance is also intertwined with broader trends in the digital asset market, including the adoption of stablecoins and the evolution of decentralized finance (DeFi).
Looking ahead, the S&P Bitcoin Index is expected to experience continued volatility, yet the long-term outlook is conditionally positive. Continued institutional adoption and the maturation of regulatory frameworks would support this scenario. Improved scalability of blockchain and enhanced user experience could lead to wider adoption, enhancing the index. The primary risk to this positive forecast involves unfavorable regulatory developments, which could hinder market growth. Additionally, potential security breaches or a loss of confidence in the broader cryptocurrency market could trigger sharp declines in the index's value. The impact of macroeconomic factors such as inflation and interest rates also represents a significant risk. Unexpected events, such as major technological glitches or geopolitical instability, pose further challenges to the index's performance. Investors should carefully monitor the evolving landscape and consider the high-risk, high-reward characteristics of this asset class before investing.
Rating | Short-Term | Long-Term Senior |
---|---|---|
Outlook | B2 | Ba3 |
Income Statement | Baa2 | B3 |
Balance Sheet | C | Baa2 |
Leverage Ratios | Caa2 | Baa2 |
Cash Flow | C | Ba3 |
Rates of Return and Profitability | Baa2 | Caa2 |
*An aggregate rating for an index summarizes the overall sentiment towards the companies it includes. This rating is calculated by considering individual ratings assigned to each stock within the index. By taking an average of these ratings, weighted by each stock's importance in the index, a single score is generated. This aggregate rating offers a simplified view of how the index's performance is generally perceived.
How does neural network examine financial reports and understand financial state of the company?
References
- M. Colby, T. Duchow-Pressley, J. J. Chung, and K. Tumer. Local approximation of difference evaluation functions. In Proceedings of the Fifteenth International Joint Conference on Autonomous Agents and Multiagent Systems, Singapore, May 2016
- V. Borkar and R. Jain. Risk-constrained Markov decision processes. IEEE Transaction on Automatic Control, 2014
- V. Mnih, K. Kavukcuoglu, D. Silver, A. Rusu, J. Veness, M. Bellemare, A. Graves, M. Riedmiller, A. Fidjeland, G. Ostrovski, S. Petersen, C. Beattie, A. Sadik, I. Antonoglou, H. King, D. Kumaran, D. Wierstra, S. Legg, and D. Hassabis. Human-level control through deep reinforcement learning. Nature, 518(7540):529–533, 02 2015.
- Swaminathan A, Joachims T. 2015. Batch learning from logged bandit feedback through counterfactual risk minimization. J. Mach. Learn. Res. 16:1731–55
- R. Howard and J. Matheson. Risk sensitive Markov decision processes. Management Science, 18(7):356– 369, 1972
- H. Kushner and G. Yin. Stochastic approximation algorithms and applications. Springer, 1997.
- M. L. Littman. Friend-or-foe q-learning in general-sum games. In Proceedings of the Eighteenth International Conference on Machine Learning (ICML 2001), Williams College, Williamstown, MA, USA, June 28 - July 1, 2001, pages 322–328, 2001