AUC Score :
Short-term Tactic1 :
Dominant Strategy :
Time series to forecast n:
ML Model Testing : Inductive Learning (ML)
Hypothesis Testing : Beta
Surveillance : Major exchange and OTC
1Short-term revised.
2Time series is updated based on short-term trends.
Key Points
RIO's future appears cautiously optimistic, predicated on sustained demand for commodities, particularly iron ore, and the company's strategic focus on decarbonization initiatives. However, potential risks include volatile commodity prices, geopolitical instability impacting supply chains and operations, and the increasing pressure from environmental regulations and community relations, particularly related to its mining operations. Further, challenges associated with project execution, such as delays or cost overruns, could hinder RIO's ability to meet production targets and impact profitability. The company also faces risks associated with its significant exposure to China, a key market and source of demand, and the ongoing global economic uncertainty, which could dampen overall demand for raw materials.About Rio Tinto Plc
Rio Tinto (RIO) is a leading global mining and metals company. Headquartered in London, the company has operations across numerous countries, producing a wide array of materials essential for infrastructure, construction, and manufacturing. Its primary commodities include iron ore, aluminum, copper, diamonds, and industrial minerals. RIO operates through a diverse portfolio of mines and processing facilities, focusing on efficient extraction and responsible resource management.
RIO is committed to sustainable development, emphasizing environmental stewardship, community engagement, and ethical business practices. It strives to balance its economic objectives with its social and environmental responsibilities. The company is publicly listed on the London Stock Exchange, the Australian Securities Exchange, and the New York Stock Exchange, attracting investors globally. Its strategic priorities include portfolio optimization, operational excellence, and innovation in the mining and processing sectors.
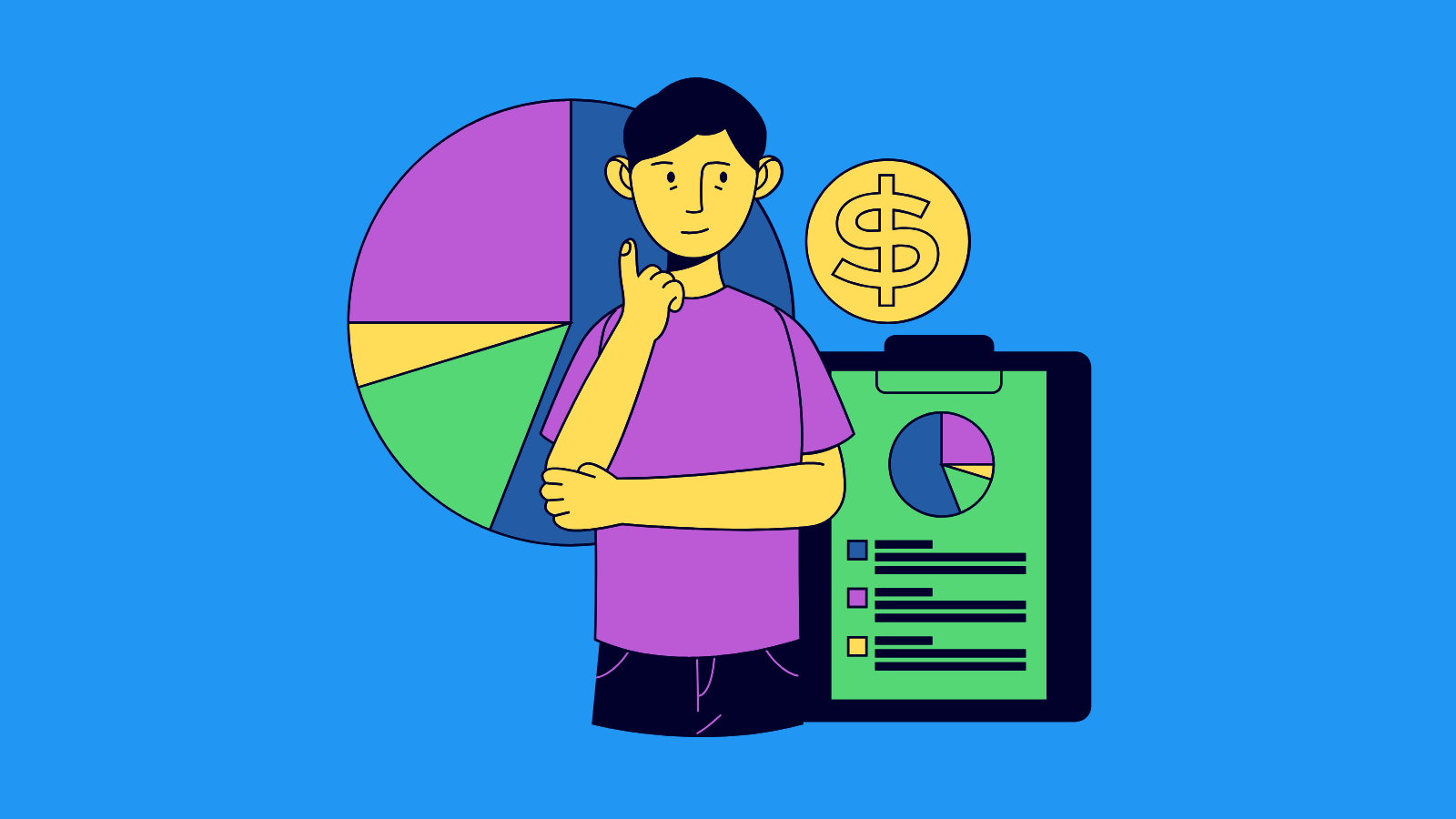
RIO Stock: Machine Learning Forecasting Model
Our team proposes a robust machine learning model for forecasting the performance of Rio Tinto Plc Common Stock (RIO). This model integrates diverse datasets to capture the multifaceted nature of the mining industry and global economic influences. We will utilize a combination of supervised learning techniques, focusing on time series analysis and predictive modeling. The core components of our model will include, but not be limited to, a recurrent neural network (RNN), specifically a Long Short-Term Memory (LSTM) network, chosen for its proficiency in capturing sequential dependencies in time series data. This will be complemented by a gradient boosting machine (GBM) to incorporate a broad range of economic and industry-specific indicators. Feature engineering will be a critical step, encompassing the construction of relevant variables from raw data and the inclusion of macroeconomic indicators such as GDP growth, inflation rates, and exchange rates, along with commodity prices (iron ore, copper, aluminum).
The model's architecture will incorporate both technical and fundamental data inputs. Technical indicators will derive from historical price and volume data, including moving averages, relative strength index (RSI), and volume oscillators to detect trends and patterns. Fundamental analysis will include financial statements (income statement, balance sheet, cash flow statement) of RIO and its competitors, as well as, industry-specific data such as global demand projections, production capacity, and geopolitical factors impacting the mining sector. These distinct data sources will be preprocessed to address data quality issues, including missing values and outliers. Feature selection techniques, such as recursive feature elimination, will be employed to identify the most impactful variables, enhance model performance, and mitigate the risk of overfitting. The dataset will be split into training, validation, and test sets to accurately measure model performance and generalization capabilities.
The model's output will be a forecast of RIO's performance over a specified timeframe, with associated confidence intervals. The evaluation metrics will include mean squared error (MSE), root mean squared error (RMSE), and mean absolute percentage error (MAPE) for accuracy. We will use the validation dataset to fine-tune hyperparameters of the RNN and GBM models, while the test dataset will be used to assess the model's predictive power. The model's performance will be rigorously evaluated using a combination of backtesting and simulation techniques. Regular model retraining and updates will be performed, incorporating the latest data and evolving market dynamics. The final product is a comprehensive and dynamic tool to forecast the RIO stock that would provide valuable information to investment decisions.
ML Model Testing
n:Time series to forecast
p:Price signals of Rio Tinto Plc stock
j:Nash equilibria (Neural Network)
k:Dominated move of Rio Tinto Plc stock holders
a:Best response for Rio Tinto Plc target price
For further technical information as per how our model work we invite you to visit the article below:
How do KappaSignal algorithms actually work?
Rio Tinto Plc Stock Forecast (Buy or Sell) Strategic Interaction Table
Strategic Interaction Table Legend:
X axis: *Likelihood% (The higher the percentage value, the more likely the event will occur.)
Y axis: *Potential Impact% (The higher the percentage value, the more likely the price will deviate.)
Z axis (Grey to Black): *Technical Analysis%
Rio Tinto's Financial Outlook and Forecast
The financial outlook for Rio Tinto (RIO) appears to be cautiously optimistic, driven by a confluence of factors impacting the global commodities market. The company, a global leader in the mining industry, benefits from a diversified portfolio of assets spanning iron ore, aluminum, copper, diamonds, and other minerals. Strong demand from emerging markets, particularly in Asia, is projected to continue to fuel the demand for its core products, specifically iron ore, which constitutes a significant portion of its revenue. Infrastructure development, urbanization, and the growing middle class in these regions are key drivers behind this sustained demand. Furthermore, Rio Tinto's ongoing efforts to enhance operational efficiency, including technological advancements and cost-cutting measures, are expected to positively impact profitability and strengthen its financial position. The company is also strategically investing in projects that are aligned with the global energy transition, indicating a commitment to sustainable practices and securing its long-term viability.
The aluminum and copper segments are also poised for growth. The increasing electrification of the global economy and the rising demand for electric vehicles are anticipated to drive demand for copper, a vital component in wiring and other electrical applications. Aluminum is essential to the transportation and construction industries and should see solid demand. However, the specific performance of each commodity segment will likely be influenced by a number of factors, including global economic cycles, shifts in supply and demand dynamics, and governmental regulations. Rio Tinto's financial performance is highly correlated to commodity prices, thus variations in these prices can cause fluctuations in the company's revenue and profitability. Moreover, the company's dividend policy, typically a significant draw for investors, will depend on financial performance, cash flow generation, and prevailing market conditions.
Rio Tinto's capital expenditure strategy will have an important impact on future financial performance. Investments in new mines, expansions of existing operations, and acquisitions will drive future output and revenue. Careful management of capital allocation will be essential. The company will be prudent in evaluating projects, and focus on maximizing return on capital. The company's response to governmental regulation and environmental concerns should also be carefully observed. The mining industry is subject to significant scrutiny regarding its environmental impact. Compliance with stricter environmental regulations and implementing sustainable mining practices will be crucial for maintaining its social license to operate and mitigating potential risks associated with environmental liabilities. Additionally, geopolitical risks and trade tensions, particularly in key markets like China, could influence the supply chain.
In conclusion, the overall financial outlook for RIO is positive. The company is well-positioned to benefit from long-term trends in the commodities market, its diversified portfolio, and efficiency initiatives will serve the company well. However, there are several key risks. A significant slowdown in the Chinese economy or other emerging markets, a downturn in global commodity prices, or stricter environmental regulations could negatively impact financial results. Geopolitical instability and supply chain disruptions are also concerns. In the long term, RIO is predicted to perform better than other companies but there are many potential risks.
Rating | Short-Term | Long-Term Senior |
---|---|---|
Outlook | B1 | Ba3 |
Income Statement | Baa2 | B1 |
Balance Sheet | Baa2 | B3 |
Leverage Ratios | Caa2 | Baa2 |
Cash Flow | C | B2 |
Rates of Return and Profitability | B2 | Ba1 |
*Financial analysis is the process of evaluating a company's financial performance and position by neural network. It involves reviewing the company's financial statements, including the balance sheet, income statement, and cash flow statement, as well as other financial reports and documents.
How does neural network examine financial reports and understand financial state of the company?
References
- M. J. Hausknecht. Cooperation and Communication in Multiagent Deep Reinforcement Learning. PhD thesis, The University of Texas at Austin, 2016
- Hirano K, Porter JR. 2009. Asymptotics for statistical treatment rules. Econometrica 77:1683–701
- Athey S, Bayati M, Imbens G, Zhaonan Q. 2019. Ensemble methods for causal effects in panel data settings. NBER Work. Pap. 25675
- Wooldridge JM. 2010. Econometric Analysis of Cross Section and Panel Data. Cambridge, MA: MIT Press
- P. Marbach. Simulated-Based Methods for Markov Decision Processes. PhD thesis, Massachusetts Institute of Technology, 1998
- Athey S, Imbens GW. 2017a. The econometrics of randomized experiments. In Handbook of Economic Field Experiments, Vol. 1, ed. E Duflo, A Banerjee, pp. 73–140. Amsterdam: Elsevier
- Imbens G, Wooldridge J. 2009. Recent developments in the econometrics of program evaluation. J. Econ. Lit. 47:5–86