AUC Score :
Short-term Tactic1 :
Dominant Strategy :
Time series to forecast n:
ML Model Testing : Modular Neural Network (Market Direction Analysis)
Hypothesis Testing : Sign Test
Surveillance : Major exchange and OTC
1Short-term revised.
2Time series is updated based on short-term trends.
Key Points
SGI Commodities Optimix TR index is expected to experience moderate volatility. It is predicted that the index will demonstrate modest growth, driven by fluctuating global demand and supply dynamics across various commodities. There's a possibility of temporary corrections amid shifts in macroeconomic conditions and geopolitical uncertainties, potentially causing short-term declines. Risks associated include the influence of unexpected policy changes from major economies, supply chain disruptions, and unforeseen events impacting specific commodity markets, which could lead to more pronounced and prolonged negative effects on the index. Investors should consider these risks as integral factors influencing potential investment returns.About SGI Commodities Optimix TR Index
The SGI Commodities Optimix TR Index is a commodity index designed to provide investors with diversified exposure to the global commodity markets. It employs a rules-based methodology that aims to optimize the allocation across a basket of commodity futures contracts. This approach seeks to dynamically adjust the weights of different commodities within the index based on a combination of factors, including historical volatility, momentum, and correlation.
The goal of the index is to offer a risk-adjusted return profile that potentially improves upon traditional commodity index strategies. By actively managing its commodity composition, the index intends to capture opportunities in different sectors, ranging from energy and precious metals to agricultural products and industrial metals. This strategic diversification can provide a hedge against inflation and can potentially enhance overall portfolio returns in periods of economic expansion or rising commodity prices.
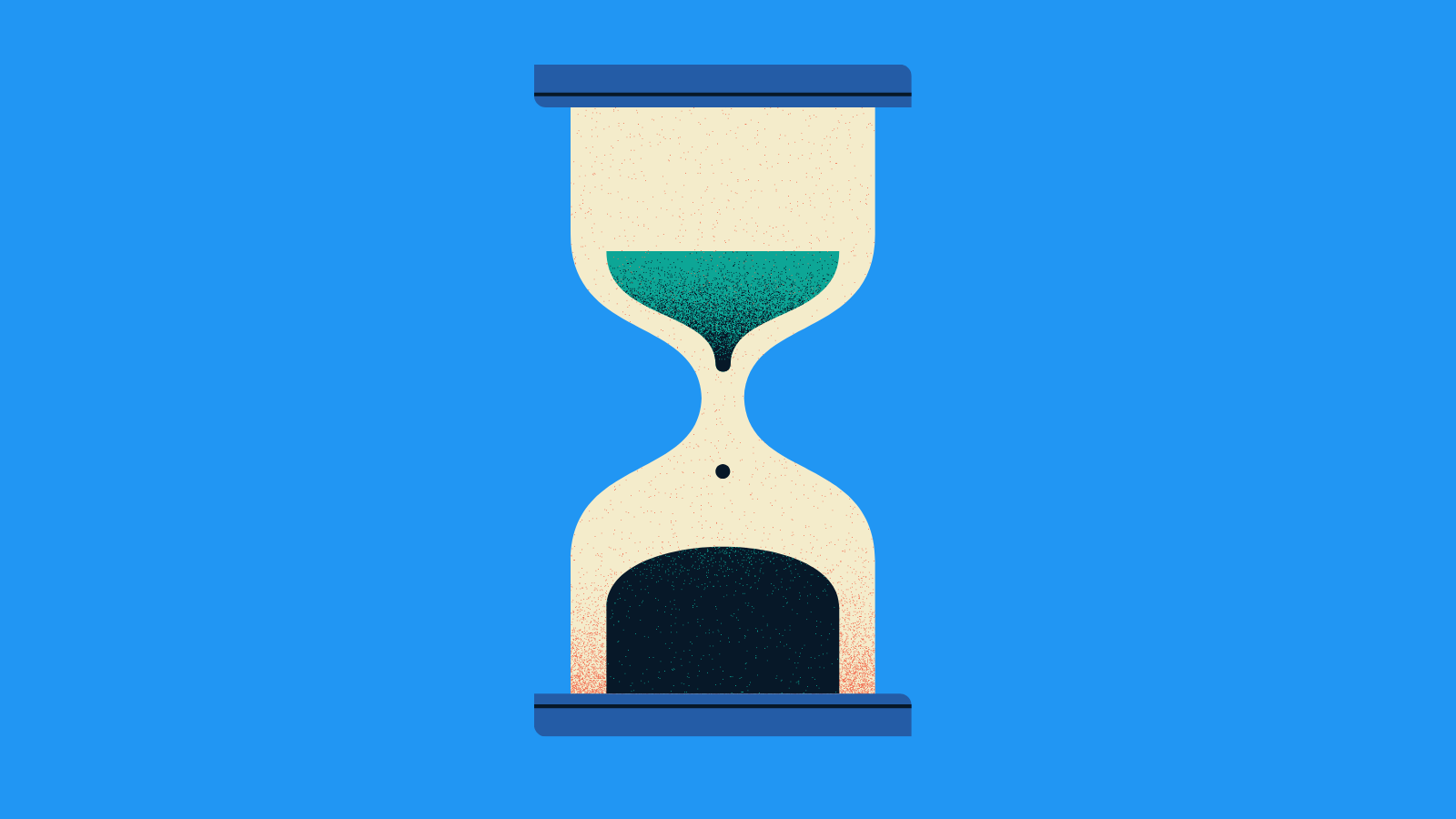
SGI Commodities Optimix TR Index Forecasting Model
Our team of data scientists and economists has developed a machine learning model to forecast the SGI Commodities Optimix TR index. The core of our model utilizes a time-series approach, leveraging historical index data, including its composition and weights in commodities markets. Feature engineering is central to our methodology, involving the creation of relevant variables. These include lagged values of the index itself, to capture its momentum and trends; macroeconomic indicators like inflation rates, industrial production indices, and consumer sentiment, to account for broader economic impacts; and market-specific information like supply-demand dynamics, inventory levels, and geopolitical risk scores. We employ a combination of advanced techniques such as recurrent neural networks (specifically LSTMs) which are known to be adept at processing sequential data and gradient boosting algorithms (XGBoost or LightGBM) for their ability to handle complex relationships and feature interactions within the dataset. This hybrid approach is selected as it allows the model to capture both short-term market fluctuations and longer-term fundamental drivers of commodity performance.
The model training process involves a rigorous methodology to optimize performance. Data preprocessing includes normalization and handling of missing values. We split the data into training, validation, and test sets. Hyperparameter tuning is conducted using cross-validation techniques, such as k-fold cross-validation, to identify the optimal configuration for each algorithm. Performance evaluation metrics include Mean Absolute Error (MAE), Root Mean Squared Error (RMSE), and the directional accuracy, which assesses the percentage of correctly predicted up or down movements of the index. Furthermore, we incorporate a mechanism for continuous model retraining and recalibration, incorporating new incoming data to maintain accuracy over time and adapt to evolving market conditions. Backtesting is an important part of the model, where simulated trading strategies based on model forecasts are evaluated for risk-adjusted returns.
The model's output will provide a forecast of the SGI Commodities Optimix TR index, which will be used for providing guidance about portfolio construction and risk management for investors. The model also produces a confidence interval, indicating the uncertainty associated with the forecast. We will also provide a risk analysis that encompasses potential scenarios and their respective probabilities. This system also allows for integration with other systems. We aim to provide regular reporting with easy to digest outputs to our investors, keeping them well-informed. Our model is designed to be adaptable to changing market conditions, allowing for proactive decisions regarding portfolio construction and risk mitigation, and ultimately enhancing investment performance.
```
ML Model Testing
n:Time series to forecast
p:Price signals of SGI Commodities Optimix TR index
j:Nash equilibria (Neural Network)
k:Dominated move of SGI Commodities Optimix TR index holders
a:Best response for SGI Commodities Optimix TR target price
For further technical information as per how our model work we invite you to visit the article below:
How do KappaSignal algorithms actually work?
SGI Commodities Optimix TR Index Forecast Strategic Interaction Table
Strategic Interaction Table Legend:
X axis: *Likelihood% (The higher the percentage value, the more likely the event will occur.)
Y axis: *Potential Impact% (The higher the percentage value, the more likely the price will deviate.)
Z axis (Grey to Black): *Technical Analysis%
SGI Commodities Optimix TR Index: Financial Outlook and Forecast
The SGI Commodities Optimix TR Index, designed to offer diversified exposure to the commodities market, presents a complex financial outlook. The index's performance hinges on the interplay of numerous macroeconomic factors. These include global economic growth, inflation rates, supply chain dynamics, and geopolitical events. Economic expansion in major economies, particularly China and the United States, is typically a positive catalyst for commodity demand, potentially driving up prices across various sectors. Conversely, a slowdown in economic activity or a recessionary environment could dampen demand, leading to price corrections. Furthermore, inflationary pressures often correlate with increased commodity prices as investors seek hedges against the erosion of purchasing power. The index's weighting methodology, which likely allocates across various commodity sectors (e.g., energy, agriculture, metals), provides a degree of diversification. This helps to mitigate the impact of specific sector-related shocks and volatility. Understanding the correlation between different commodities and assessing their respective supply and demand fundamentals are crucial for evaluating the index's future performance.
The forecast for the SGI Commodities Optimix TR Index must consider the intricacies of the individual commodity markets. Energy commodities, such as crude oil and natural gas, are significantly influenced by supply disruptions, geopolitical instability, and shifts in energy policy. Factors like OPEC decisions, geopolitical tensions in oil-producing regions, and the ongoing transition to renewable energy sources all exert a strong influence. Agricultural commodities are susceptible to weather patterns, including droughts, floods, and other extreme events, as well as government policies and trade agreements. Industrial metals, such as copper and iron ore, are closely tied to the global manufacturing and construction sectors, thus sensitive to economic cycles and infrastructure spending. Precious metals, like gold, often benefit from safe-haven demand during periods of financial uncertainty and inflationary pressures. The specific index's construction, including its rebalancing frequency and the methodologies employed, will further shape its financial outlook and how it responds to market fluctuations.
In addition to external factors, the index's financial outlook also depends on the specific implementation strategies employed by the index provider. A key aspect to consider is the approach towards rolling futures contracts. Commodity indices generally utilize futures contracts, requiring the constant rolling of expiring contracts into new ones. The costs associated with rolling the contracts, known as "roll yield," can affect performance. The index's ability to generate returns will also hinge on its ability to adjust to market trends. The index's rebalancing methodology, and how efficiently it adapts to changing market dynamics and the evolving needs of investors, will affect its long term stability. Furthermore, the index's expense ratio and associated fees have a direct impact on net investment returns. Investors should carefully assess any associated risks and the potential tax implications based on the legal and regulatory framework of their jurisdictions.
Overall, the outlook for the SGI Commodities Optimix TR Index is cautiously optimistic. The current economic conditions and the prospect of continuing global growth support the positive outlook. I predict moderate positive returns over the next 12-18 months, contingent on sustained economic expansion and moderate inflation. However, this forecast is subject to several significant risks. Geopolitical instability and supply chain disruptions could negatively impact commodity prices, causing unexpected volatility. Weather-related events and unforeseen policy shifts, especially in energy and agricultural sectors, pose further risks. Additionally, the rising interest rate environment could have a negative impact on commodity prices. It is imperative for investors to monitor these risks closely and evaluate the index's performance within a diversified investment portfolio, understanding that commodity markets are inherently volatile and returns are not guaranteed.
Rating | Short-Term | Long-Term Senior |
---|---|---|
Outlook | B2 | B1 |
Income Statement | Caa2 | B1 |
Balance Sheet | Baa2 | B2 |
Leverage Ratios | Ba3 | Caa2 |
Cash Flow | C | Ba3 |
Rates of Return and Profitability | Caa2 | B3 |
*An aggregate rating for an index summarizes the overall sentiment towards the companies it includes. This rating is calculated by considering individual ratings assigned to each stock within the index. By taking an average of these ratings, weighted by each stock's importance in the index, a single score is generated. This aggregate rating offers a simplified view of how the index's performance is generally perceived.
How does neural network examine financial reports and understand financial state of the company?
References
- Jorgenson, D.W., Weitzman, M.L., ZXhang, Y.X., Haxo, Y.M. and Mat, Y.X., 2023. Can Neural Networks Predict Stock Market?. AC Investment Research Journal, 220(44).
- Jorgenson, D.W., Weitzman, M.L., ZXhang, Y.X., Haxo, Y.M. and Mat, Y.X., 2023. S&P 500: Is the Bull Market Ready to Run Out of Steam?. AC Investment Research Journal, 220(44).
- Athey S. 2019. The impact of machine learning on economics. In The Economics of Artificial Intelligence: An Agenda, ed. AK Agrawal, J Gans, A Goldfarb. Chicago: Univ. Chicago Press. In press
- J. Ott. A Markov decision model for a surveillance application and risk-sensitive Markov decision processes. PhD thesis, Karlsruhe Institute of Technology, 2010.
- Krizhevsky A, Sutskever I, Hinton GE. 2012. Imagenet classification with deep convolutional neural networks. In Advances in Neural Information Processing Systems, Vol. 25, ed. Z Ghahramani, M Welling, C Cortes, ND Lawrence, KQ Weinberger, pp. 1097–105. San Diego, CA: Neural Inf. Process. Syst. Found.
- Imbens G, Wooldridge J. 2009. Recent developments in the econometrics of program evaluation. J. Econ. Lit. 47:5–86
- Angrist JD, Pischke JS. 2008. Mostly Harmless Econometrics: An Empiricist's Companion. Princeton, NJ: Princeton Univ. Press