AUC Score :
Short-term Tactic1 :
Dominant Strategy :
Time series to forecast n:
ML Model Testing : Statistical Inference (ML)
Hypothesis Testing : Beta
Surveillance : Major exchange and OTC
1Short-term revised.
2Time series is updated based on short-term trends.
Key Points
Omnicom Group may experience moderate growth in the advertising and marketing sector, driven by its diverse client portfolio and global presence. The company is likely to benefit from the increasing demand for digital advertising solutions and data-driven marketing strategies. However, risks include the potential for economic downturns impacting advertising spend, increased competition from technology giants, and fluctuations in currency exchange rates, particularly affecting international revenues. Furthermore, evolving privacy regulations and consumer behavior changes pose challenges to its traditional advertising models, potentially impacting its financial performance. The success of strategic acquisitions and ability to adapt to rapid technological advancements are critical for sustained growth and profitability.About Omnicom Group
Omnicom Group Inc. is a global marketing and corporate communications company. It provides advertising, marketing, and corporate communications services to over 5,000 clients in more than 70 countries. The company operates through a network of branded agencies, offering a wide range of services including advertising, strategic media planning and buying, digital and interactive marketing, public relations, and specialty communications.
OMC's structure encompasses several agency networks and standalone businesses. These entities operate within the advertising, customer relationship management (CRM), and public relations sectors. The company's diversified portfolio allows it to serve a broad spectrum of industries, supporting clients in developing and executing integrated marketing strategies. OMC emphasizes innovation and the utilization of data analytics to enhance its service offerings and maintain a competitive edge in the evolving media landscape.
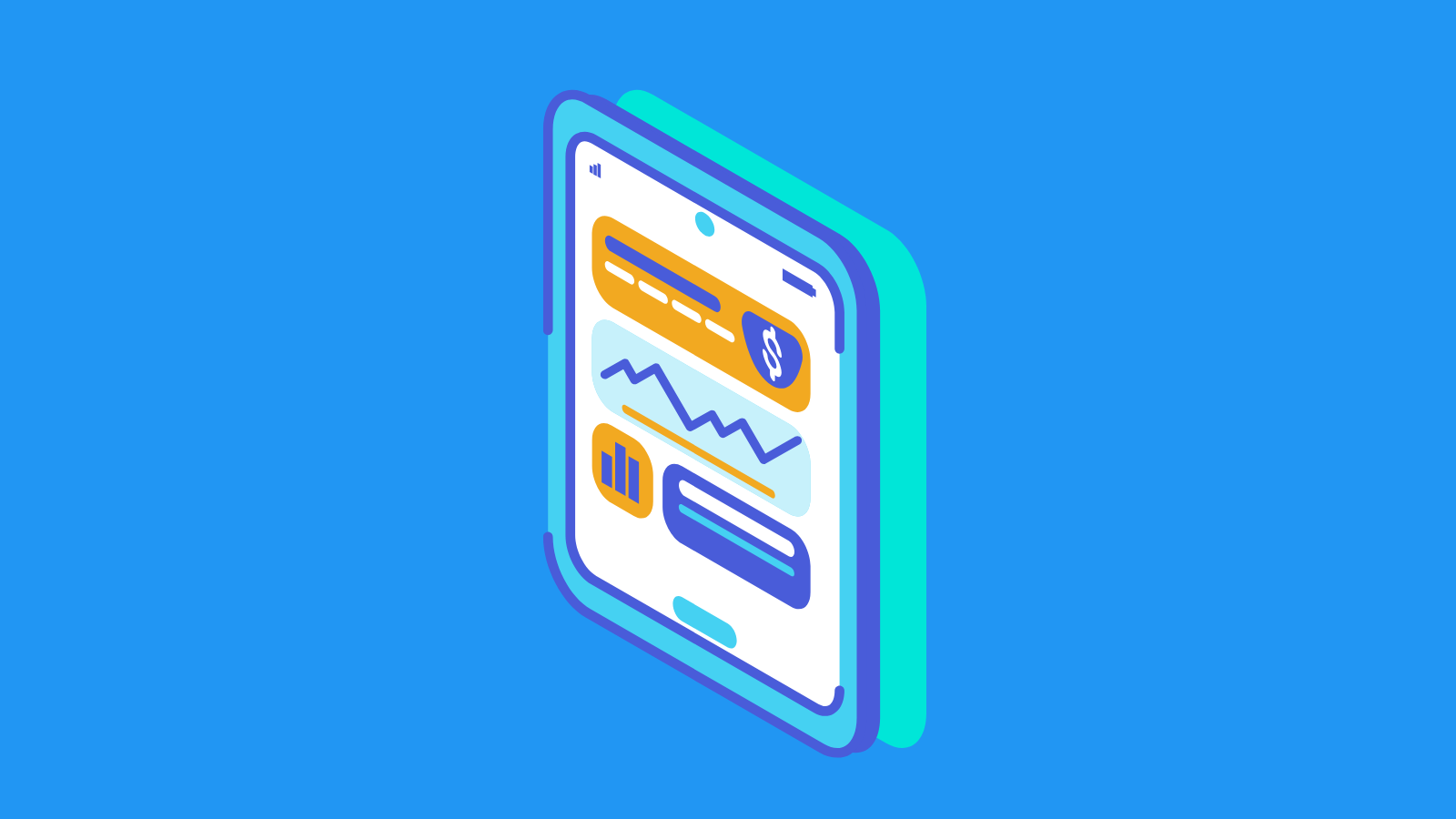
OMC Stock Prediction Model
Our team of data scientists and economists has developed a machine learning model for forecasting the future performance of Omnicom Group Inc. (OMC) common stock. This model leverages a comprehensive set of financial and economic indicators. Input data includes, but is not limited to, historical stock price data, trading volumes, quarterly and annual financial reports (including revenue, earnings per share, and debt levels), industry-specific performance metrics (advertising expenditure, marketing spend, and agency performance data), macroeconomic factors (GDP growth, inflation rates, interest rates, consumer confidence indexes), and competitive landscape information (market share, competitor analysis). Various machine learning algorithms are tested and evaluated, including time series analysis methods (like ARIMA and Exponential Smoothing), regression models (such as linear regression, support vector regression), and ensemble methods (Random Forest, Gradient Boosting). Feature engineering is used extensively to create new variables from the raw data, allowing for a more sophisticated model.
The model is trained using a significant historical dataset, split into training, validation, and testing sets. The training set is used to teach the model the relationship between the input variables and the desired output (stock performance). The validation set is used to tune the model's hyperparameters and avoid overfitting. The testing set, which the model has never seen, is used to evaluate the model's performance on new data. Model evaluation uses several key metrics, including Mean Absolute Error (MAE), Mean Squared Error (MSE), Root Mean Squared Error (RMSE), and R-squared. We also conduct a thorough analysis of the model's predictions, assessing their accuracy, precision, and volatility. We use techniques for model explanation, like SHAP values, to understand which features influence the predictions most significantly. Regular backtesting will be conducted to maintain the predictive power of the model.
Furthermore, our model includes a mechanism for handling market events, such as economic recessions or mergers and acquisitions, which might have an influence on the predictions. We plan to continuously improve the model by updating the input data with fresh information, incorporating new relevant factors, and refining the machine learning algorithms. To provide robust, reliable results, a risk management framework is included. This framework ensures that the model's predictions are used in conjunction with other forms of analysis and that the model's uncertainty is communicated clearly to all stakeholders. We also plan to make use of sentiment analysis extracted from news articles and social media to detect and react to market sentiment, which could affect OMC stock performance.
```
ML Model Testing
n:Time series to forecast
p:Price signals of Omnicom Group stock
j:Nash equilibria (Neural Network)
k:Dominated move of Omnicom Group stock holders
a:Best response for Omnicom Group target price
For further technical information as per how our model work we invite you to visit the article below:
How do KappaSignal algorithms actually work?
Omnicom Group Stock Forecast (Buy or Sell) Strategic Interaction Table
Strategic Interaction Table Legend:
X axis: *Likelihood% (The higher the percentage value, the more likely the event will occur.)
Y axis: *Potential Impact% (The higher the percentage value, the more likely the price will deviate.)
Z axis (Grey to Black): *Technical Analysis%
Omnicom Group Inc. (OMC) Financial Outlook and Forecast
OMC, a leading global marketing and corporate communications company, displays a robust financial outlook underpinned by its diversified service offerings and strong client relationships. The company's integrated approach, encompassing advertising, strategic media planning and buying, digital marketing, public relations, and specialty communications, positions it to capitalize on the evolving demands of the marketing landscape. OMC's global footprint, with operations across numerous countries, provides diversification and reduces dependence on any single geographic market. Furthermore, OMC's proven ability to adapt to changing marketing trends, particularly in the digital realm, has enabled it to maintain its competitive edge and attract significant client spend. The company's commitment to data analytics and technology integration is critical for delivering measurable results for clients and driving future growth. OMC's disciplined approach to cost management and a history of consistent profitability supports its financial stability and attractiveness as an investment.
The company's revenue streams are expected to exhibit moderate but consistent growth in the coming years. The increasing reliance of businesses on marketing services, especially digital marketing, coupled with OMC's diversified service portfolio will likely contribute to this growth. The ongoing recovery of the global economy and the resurgence of advertising spending in various sectors are also positive tailwinds. OMC's focus on acquiring and integrating new technologies, as well as expanding its offerings, should enable it to attract new clients and increase revenue from existing clients. Additionally, the company's ability to cross-sell its various services to its extensive client base presents a significant opportunity for revenue expansion. Strategic acquisitions, although potentially impacting margins in the short term, are likely to reinforce OMC's position in key growth areas, such as digital transformation and data analytics.
OMC's financial performance is influenced by macroeconomic factors, including global economic growth, industry-specific trends, and currency fluctuations. The marketing industry is subject to cyclical trends, with spending potentially declining during economic downturns. While digital marketing has shown resilience, the overall advertising market is susceptible to economic volatility. Furthermore, changes in client spending patterns and the consolidation within the advertising industry could pose challenges. Increased competition from both traditional and digital-focused marketing agencies also represents a risk, as does the potential for technological disruptions. The company's ability to effectively manage costs, including labor and overhead, is crucial for maintaining profitability. The regulatory environment is also a key consideration, especially regarding data privacy and advertising standards.
Overall, the financial outlook for OMC is positive. The company's strong market position, diversified service portfolio, and strategic investments position it well for moderate revenue growth and continued profitability. While risks exist related to economic cycles, competition, and changing client demands, OMC's proven track record of adapting to market changes and managing its operations mitigates these risks. The prediction is that OMC will likely continue to deliver consistent financial results, supporting its position as a leader in the global marketing and communications industry. Key risks include a slower than expected recovery in advertising spending, an inability to effectively integrate acquired businesses, and increased competition from digital-first marketing agencies.
Rating | Short-Term | Long-Term Senior |
---|---|---|
Outlook | Ba3 | B2 |
Income Statement | Baa2 | C |
Balance Sheet | Ba1 | C |
Leverage Ratios | Ba1 | C |
Cash Flow | B2 | Baa2 |
Rates of Return and Profitability | B2 | Baa2 |
*Financial analysis is the process of evaluating a company's financial performance and position by neural network. It involves reviewing the company's financial statements, including the balance sheet, income statement, and cash flow statement, as well as other financial reports and documents.
How does neural network examine financial reports and understand financial state of the company?
References
- J. G. Schneider, W. Wong, A. W. Moore, and M. A. Riedmiller. Distributed value functions. In Proceedings of the Sixteenth International Conference on Machine Learning (ICML 1999), Bled, Slovenia, June 27 - 30, 1999, pages 371–378, 1999.
- Zubizarreta JR. 2015. Stable weights that balance covariates for estimation with incomplete outcome data. J. Am. Stat. Assoc. 110:910–22
- A. Y. Ng, D. Harada, and S. J. Russell. Policy invariance under reward transformations: Theory and application to reward shaping. In Proceedings of the Sixteenth International Conference on Machine Learning (ICML 1999), Bled, Slovenia, June 27 - 30, 1999, pages 278–287, 1999.
- G. J. Laurent, L. Matignon, and N. L. Fort-Piat. The world of independent learners is not Markovian. Int. J. Know.-Based Intell. Eng. Syst., 15(1):55–64, 2011
- Tibshirani R, Hastie T. 1987. Local likelihood estimation. J. Am. Stat. Assoc. 82:559–67
- Chamberlain G. 2000. Econometrics and decision theory. J. Econom. 95:255–83
- Alpaydin E. 2009. Introduction to Machine Learning. Cambridge, MA: MIT Press