AUC Score :
Short-term Tactic1 :
Dominant Strategy :
Time series to forecast n:
ML Model Testing : Modular Neural Network (DNN Layer)
Hypothesis Testing : Logistic Regression
Surveillance : Major exchange and OTC
1Short-term revised.
2Time series is updated based on short-term trends.
Key Points
NUE is predicted to experience moderate growth, driven by increased infrastructure spending and continued demand in the construction and automotive sectors. However, the company faces risks related to fluctuating steel prices, impacted by global economic conditions, and the potential for increased competition from both domestic and international producers. Trade policies and tariffs remain a significant factor that could either positively or negatively affect profitability. Any economic downturn or slowdown in the construction and manufacturing industries could significantly impact its performance, potentially leading to reduced earnings.About Nucor Corporation
Nucor Corporation, headquartered in Charlotte, North Carolina, is a leading North American steel producer. The company operates a network of steel mills and fabrication facilities that manufacture a wide range of steel products, including carbon and alloy steel, sheet steel, and structural steel. Nucor's business model focuses on producing steel using electric arc furnaces (EAFs), which allows for greater flexibility, energy efficiency, and the use of recycled steel scrap. The company emphasizes a decentralized management structure and a performance-based compensation system.
Nucor's product portfolio serves diverse markets, including the automotive, construction, energy, and infrastructure sectors. Beyond steel production, Nucor is also involved in the fabrication of steel products like joists, decking, and metal buildings. A key aspect of Nucor's strategy is its commitment to sustainability and responsible manufacturing practices. The company continuously invests in new technologies and process improvements to minimize its environmental impact.
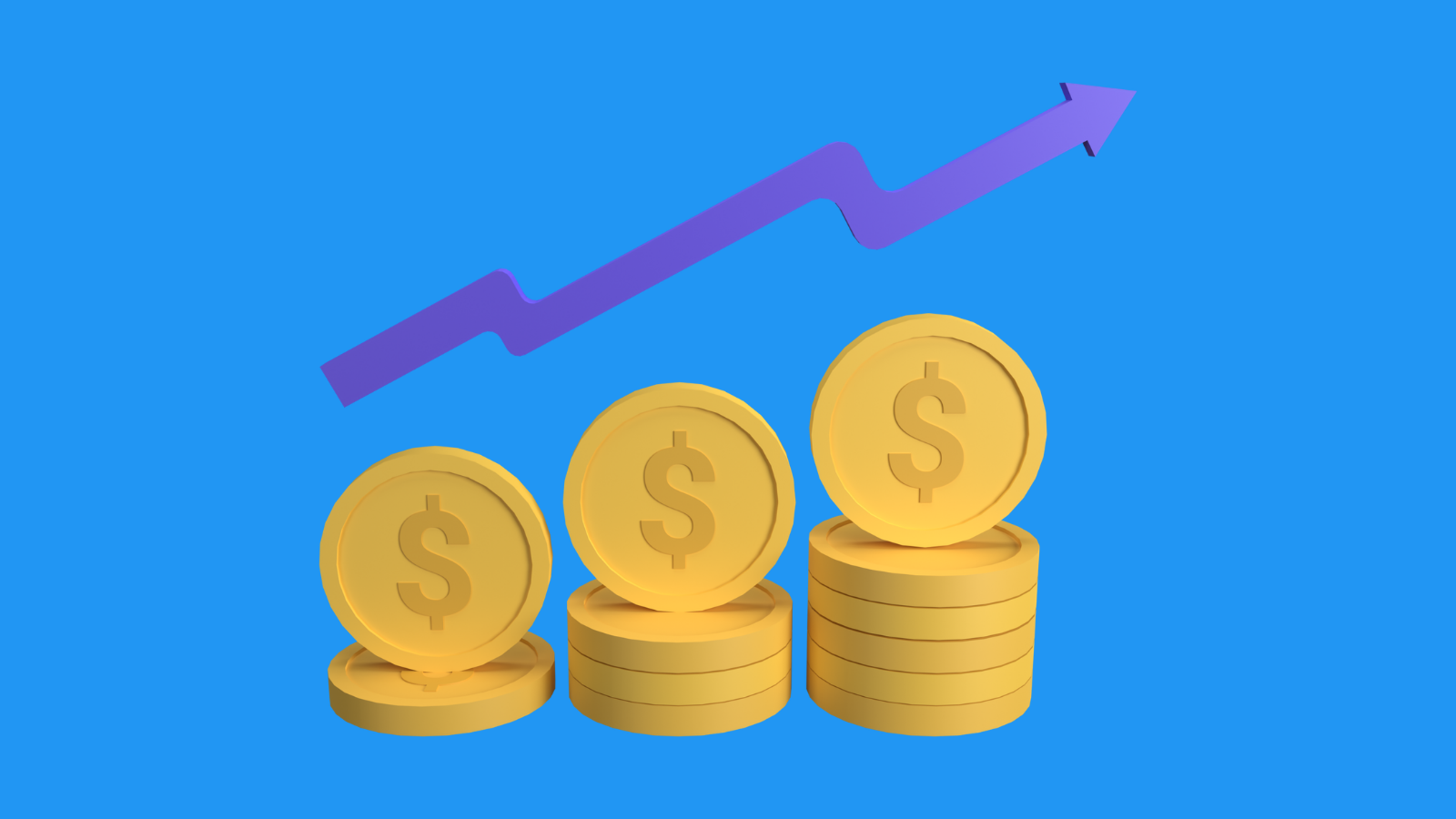
NUE Stock Price Prediction Model
Our team of data scientists and economists proposes a machine learning model to forecast the performance of Nucor Corporation (NUE) stock. The model will leverage a combination of fundamental and technical indicators to predict future stock behavior. **Fundamental indicators** will include financial ratios such as price-to-earnings (P/E) ratio, debt-to-equity ratio, and revenue growth. These ratios will be calculated using data from quarterly and annual reports, providing insights into Nucor's financial health and operational efficiency. Further, we will incorporate macroeconomic factors such as **steel demand**, **interest rates**, and **inflation**, which have a significant impact on the steel industry. Technical indicators, encompassing moving averages, Relative Strength Index (RSI), and trading volume, will capture market sentiment and identify potential trends. This comprehensive approach aims to create a robust and reliable model.
We will employ a **time-series forecasting framework** utilizing several machine learning algorithms, including **Recurrent Neural Networks (RNNs), specifically Long Short-Term Memory (LSTM) networks**, and **Gradient Boosting Machines (GBMs)**. These algorithms are well-suited for capturing complex non-linear relationships and temporal dependencies within the data. The model will be trained on a historical dataset spanning at least 10 years, with a significant portion of the data used for model training and validation. The remaining data will serve as a hold-out set for out-of-sample testing and performance evaluation. The model's performance will be assessed using various metrics, including Mean Absolute Error (MAE), Root Mean Squared Error (RMSE), and the coefficient of determination (R-squared). Regular model retraining and hyperparameter tuning will be implemented to maintain model accuracy and adaptability to changing market conditions.
The final model output will be a forecast of Nucor's future performance, encompassing potential price trends, volatility estimates, and confidence intervals. The forecasts can be used by investors to support informed decision-making. Furthermore, the model's output, along with supporting analysis, will be communicated in a clear and concise format. **Regular model monitoring**, including sensitivity analysis and performance evaluation against actual market outcomes, will be conducted. **This iterative process will ensure the model's ongoing relevance** and improve its predictive capability over time, providing a valuable tool for assessing NUE stock.
ML Model Testing
n:Time series to forecast
p:Price signals of Nucor Corporation stock
j:Nash equilibria (Neural Network)
k:Dominated move of Nucor Corporation stock holders
a:Best response for Nucor Corporation target price
For further technical information as per how our model work we invite you to visit the article below:
How do KappaSignal algorithms actually work?
Nucor Corporation Stock Forecast (Buy or Sell) Strategic Interaction Table
Strategic Interaction Table Legend:
X axis: *Likelihood% (The higher the percentage value, the more likely the event will occur.)
Y axis: *Potential Impact% (The higher the percentage value, the more likely the price will deviate.)
Z axis (Grey to Black): *Technical Analysis%
Nucor Corporation Common Stock: Financial Outlook and Forecast
The financial outlook for Nucor (NUE) remains robust, underpinned by several key factors. Strong domestic demand for steel, particularly from the automotive, construction, and infrastructure sectors, provides a solid foundation for continued growth. Government initiatives, such as the Infrastructure Investment and Jobs Act, are expected to significantly boost demand in the coming years. NUE's strategic investments in expanding its production capacity, including new mills and upgrades to existing facilities, position it to capitalize on this rising demand. Furthermore, the company's focus on producing high-quality, value-added steel products strengthens its ability to maintain profitability even during periods of market fluctuations. NUE's ability to integrate new technologies, such as electric arc furnaces, not only enhances efficiency but also allows for greater flexibility in sourcing raw materials and responding to changes in market conditions.
NUE's financial performance is closely tied to the steel industry's cyclical nature. Raw material costs, particularly for scrap metal, represent a significant expense and can impact profit margins depending on market dynamics. However, NUE's vertically integrated business model helps mitigate some of these risks. Its strategic approach, which involves operating a diverse portfolio of mills and product offerings, offers it some protection during periods of economic uncertainty. This diversification enables the company to adapt to shifting customer needs and geographic variations in demand. Strong financial discipline, demonstrated by its conservative approach to debt and consistent focus on shareholder returns, also strengthens its long-term resilience. NUE's commitment to operational excellence, including cost control and efficiency improvements, positions the company to outperform its competitors during more challenging times.
Looking ahead, the financial forecast for NUE is generally positive. The company is expected to benefit from ongoing infrastructure projects and a continued strong demand for its products across various end markets. The global supply chain disruptions from recent years are subsiding, which is helping normalize raw material costs and logistics expenses. Although economic uncertainty is a factor, the company's diversified product portfolio and geographic presence will provide a degree of protection. NUE's initiatives to reduce its carbon footprint and adopt sustainable manufacturing practices should enhance its appeal to environmentally conscious customers and investors. The company's strong financial position and disciplined capital allocation strategy provide it with flexibility to pursue strategic growth opportunities, including potential acquisitions or expansions.
In summary, the financial outlook for NUE is favorable, with anticipated growth driven by strong domestic demand, strategic investments, and operational efficiencies. This positive prediction is subject to several risks. Economic downturns and industry-specific challenges, such as import competition or a sudden drop in demand from key sectors, could negatively impact the company's financial performance. Volatility in raw material prices, especially scrap metal, presents another challenge, and any significant disruptions to NUE's supply chain could hamper production and distribution. Furthermore, geopolitical events and trade policies could affect the company's ability to compete in international markets. Despite these risks, NUE's robust financial position, strong operational capabilities, and strategic investments create a solid foundation for continued success, although potential investors should carefully monitor these factors to make well-informed decisions.
Rating | Short-Term | Long-Term Senior |
---|---|---|
Outlook | B1 | Ba2 |
Income Statement | Baa2 | Baa2 |
Balance Sheet | Baa2 | Baa2 |
Leverage Ratios | Ba1 | Caa2 |
Cash Flow | Caa2 | Baa2 |
Rates of Return and Profitability | C | B3 |
*Financial analysis is the process of evaluating a company's financial performance and position by neural network. It involves reviewing the company's financial statements, including the balance sheet, income statement, and cash flow statement, as well as other financial reports and documents.
How does neural network examine financial reports and understand financial state of the company?
References
- E. Collins. Using Markov decision processes to optimize a nonlinear functional of the final distribution, with manufacturing applications. In Stochastic Modelling in Innovative Manufacturing, pages 30–45. Springer, 1997
- Mnih A, Hinton GE. 2007. Three new graphical models for statistical language modelling. In International Conference on Machine Learning, pp. 641–48. La Jolla, CA: Int. Mach. Learn. Soc.
- Scholkopf B, Smola AJ. 2001. Learning with Kernels: Support Vector Machines, Regularization, Optimization, and Beyond. Cambridge, MA: MIT Press
- Krizhevsky A, Sutskever I, Hinton GE. 2012. Imagenet classification with deep convolutional neural networks. In Advances in Neural Information Processing Systems, Vol. 25, ed. Z Ghahramani, M Welling, C Cortes, ND Lawrence, KQ Weinberger, pp. 1097–105. San Diego, CA: Neural Inf. Process. Syst. Found.
- E. Altman. Constrained Markov decision processes, volume 7. CRC Press, 1999
- Candès E, Tao T. 2007. The Dantzig selector: statistical estimation when p is much larger than n. Ann. Stat. 35:2313–51
- J. Spall. Multivariate stochastic approximation using a simultaneous perturbation gradient approximation. IEEE Transactions on Automatic Control, 37(3):332–341, 1992.