AUC Score :
Short-term Tactic1 :
Dominant Strategy :
Time series to forecast n:
ML Model Testing : Transductive Learning (ML)
Hypothesis Testing : Statistical Hypothesis Testing
Surveillance : Major exchange and OTC
1Short-term revised.
2Time series is updated based on short-term trends.
Key Points
NIP Group Inc.'s future stock performance hinges on its ability to navigate the evolving risk landscape of the property and casualty insurance market. Predictions suggest NIP may experience moderate revenue growth fueled by strategic acquisitions and expansion into new markets, alongside increased adoption of its technology platform. However, a significant risk lies in potential regulatory changes that could impact the company's operations and profitability, as well as increasing competition in the insurance technology space. Another key risk includes the possibility of underwriting losses stemming from unforeseen catastrophic events, potentially leading to stock price volatility.About NIP Group: NIP Group Inc. ADS
NIP Group Inc. (NIPG) is an American holding company with a focus on specialty insurance programs and services. The company operates primarily through its subsidiaries, offering a range of insurance products and services designed to meet the unique needs of specific industries and niches. NIPG specializes in providing tailored insurance solutions, typically involving risk assessment, underwriting, and claims management. Their target customers include businesses and organizations that often face complex or specialized insurance requirements.
NIPG's operational strategy involves a mix of program management, underwriting, and claims administration. They often collaborate with insurance carriers, providing the expertise and infrastructure necessary to develop and distribute insurance products. The company has cultivated a strong focus on building long-term relationships with their partners and customers. They aim to provide specialized expertise and responsive service within the insurance industry.
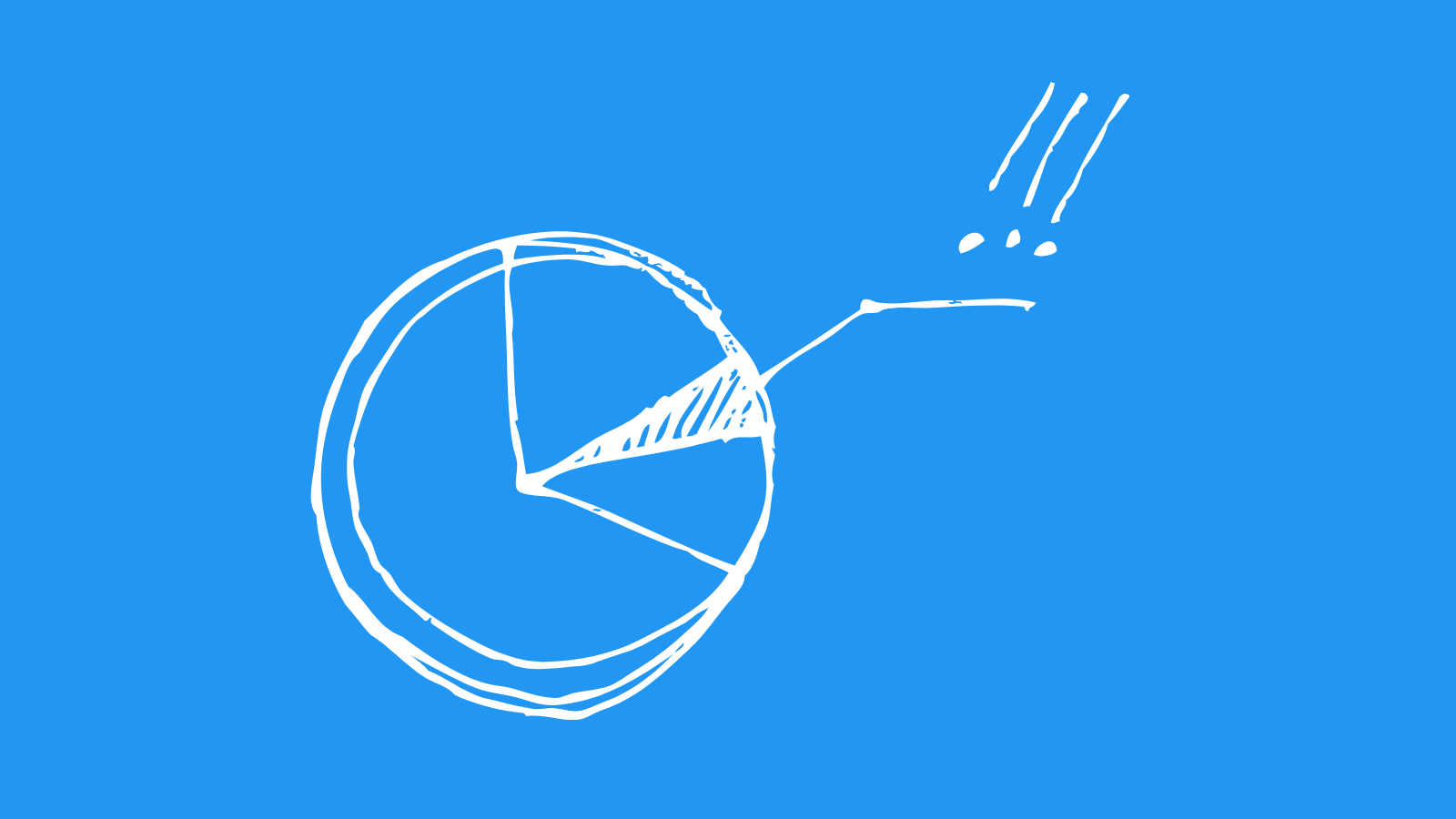
Machine Learning Model for NIPG Stock Forecast
Our team proposes a comprehensive machine learning model for forecasting the performance of NIP Group Inc. American Depositary Shares (NIPG). This model will leverage a diverse range of data sources to capture the multifaceted nature of the financial markets and the specific dynamics impacting NIPG. We will primarily focus on time-series data, incorporating historical NIPG trading volumes, moving averages, and technical indicators. Furthermore, we will integrate macroeconomic variables such as inflation rates, interest rates, and GDP growth, to assess the broader economic environment's influence. Industry-specific data, encompassing market trends, competitive landscape, and regulatory changes within the relevant sectors, will be crucial. Finally, we will implement sentiment analysis from news articles and social media platforms to gauge investor confidence and market perception surrounding NIPG and the industries it serves. Feature engineering will play a vital role in creating relevant features from the raw data, incorporating techniques like lagged variables and interaction terms.
To construct a robust forecasting system, we will employ a combination of machine learning algorithms. Initially, we will utilize ensemble methods, such as Random Forests and Gradient Boosting, due to their strong performance in handling complex, non-linear relationships and their ability to mitigate overfitting. Recurrent Neural Networks (RNNs), particularly Long Short-Term Memory (LSTM) networks, will also be considered, as they are well-suited for capturing temporal dependencies in time-series data. Furthermore, we will test various model configurations and hyperparameters to optimize performance. We will employ cross-validation and rigorous evaluation metrics, including mean squared error (MSE), mean absolute error (MAE), and root mean squared error (RMSE), to assess model accuracy and generalization capabilities. The model output will provide predicted directional movement or magnitude of change, alongside associated confidence intervals, allowing for a comprehensive understanding of potential market movements.
The development and deployment of this model will follow a structured process. Firstly, we will collect and preprocess the diverse datasets, ensuring data quality and consistency. Secondly, we will build and train the various machine learning models, comparing their performance across different metrics. Thirdly, the best-performing model will be chosen, validated, and fine-tuned. The model's effectiveness will be continuously monitored and updated through retraining, incorporating new data and refining model parameters to adapt to market shifts. We will emphasize the importance of explainable AI (XAI) techniques, providing stakeholders with clear insights into the model's predictions and underlying drivers. Furthermore, we will build a robust and reliable system with automated model updates, designed to deliver real-time insights, empowering NIPG to make informed investment decisions. We'll also focus on generating alerts to highlight potential market inefficiencies.
```
ML Model Testing
n:Time series to forecast
p:Price signals of NIP Group: NIP Group Inc. ADS stock
j:Nash equilibria (Neural Network)
k:Dominated move of NIP Group: NIP Group Inc. ADS stock holders
a:Best response for NIP Group: NIP Group Inc. ADS target price
For further technical information as per how our model work we invite you to visit the article below:
How do KappaSignal algorithms actually work?
NIP Group: NIP Group Inc. ADS Stock Forecast (Buy or Sell) Strategic Interaction Table
Strategic Interaction Table Legend:
X axis: *Likelihood% (The higher the percentage value, the more likely the event will occur.)
Y axis: *Potential Impact% (The higher the percentage value, the more likely the price will deviate.)
Z axis (Grey to Black): *Technical Analysis%
NIP Group Inc. (NIP) Financial Outlook and Forecast
NIP, a significant player in the insurance and risk management sector, currently demonstrates a complex financial landscape. The company's performance is intrinsically linked to the volatility of the insurance market, macroeconomic trends, and its strategic investments in technology and expansion. Recent financial reports indicate a mixed bag; while revenue streams show resilience, profitability margins are subject to pressure due to escalating operational costs, particularly within claims processing and regulatory compliance. Furthermore, NIP's ability to attract and retain top talent is critical, as the competitive landscape intensifies. The company's capital allocation strategies, including potential acquisitions and share repurchases, warrant close scrutiny as they will significantly influence future growth. Overall, NIP's financial position is solid, supported by its diversified portfolio and a strong client base; however, the company must effectively manage its expenses and navigate the challenges of an evolving market to maintain its competitive advantage.
The forecast for NIP hinges on its ability to adapt to technological advancements and market dynamics. The rise of insurtech poses both challenges and opportunities. NIP must invest strategically in data analytics, automation, and digital platforms to streamline operations and improve customer service. The company's expansion plans into emerging markets could create new revenue streams but also introduce new risks. Growth is also dependent on maintaining and strengthening partnerships with brokers and distribution channels, as they play a crucial role in accessing target markets. Moreover, changes in regulations and legislation concerning the insurance industry, such as data privacy rules and cybersecurity standards, could impact the cost of doing business, therefore, NIP must stay compliant. The continued development and implementation of innovative insurance products will be essential to address changing customer needs and maintain relevance.
Key indicators to watch for include NIP's claims ratio, expense management metrics, customer retention rates, and the success of any new product launches. The company's debt levels and cash flow generation capacity will be key to ensuring financial flexibility and allow it to fund growth initiatives. Additionally, it's imperative that NIP maintains a positive reputation and strong brand image within the industry and with its clientele to avoid long term complications. NIP's management team must demonstrate effective leadership and a clear strategic vision, particularly in navigating potential economic downturns and external pressures. The company's ability to successfully integrate acquired businesses and generate synergies will also be a crucial determinant of its overall future performance. This is important because in such mergers or acquisitions, risks of failed integration could weaken the company's finances.
Based on current trends and NIP's strategic direction, a moderate positive outlook seems appropriate. The company's established market presence, coupled with its investments in innovation, positions it well for growth. However, there are risks to this forecast. These include increased competition from both established players and new insurtech entrants, potential economic slowdowns impacting insurance demand, and unexpected catastrophes that could lead to large claims payouts. Furthermore, regulatory changes and data breaches pose significant risks. Successfully navigating these challenges is crucial. Therefore, a successful future depends on management's ability to implement its strategic plan effectively, adapt to evolving market conditions and effectively navigate the complexities of the insurance industry.
Rating | Short-Term | Long-Term Senior |
---|---|---|
Outlook | Caa2 | Ba3 |
Income Statement | Caa2 | C |
Balance Sheet | Caa2 | Ba3 |
Leverage Ratios | C | Baa2 |
Cash Flow | B3 | Ba1 |
Rates of Return and Profitability | Caa2 | Baa2 |
*Financial analysis is the process of evaluating a company's financial performance and position by neural network. It involves reviewing the company's financial statements, including the balance sheet, income statement, and cash flow statement, as well as other financial reports and documents.
How does neural network examine financial reports and understand financial state of the company?
References
- Chernozhukov V, Chetverikov D, Demirer M, Duflo E, Hansen C, Newey W. 2017. Double/debiased/ Neyman machine learning of treatment effects. Am. Econ. Rev. 107:261–65
- K. Boda, J. Filar, Y. Lin, and L. Spanjers. Stochastic target hitting time and the problem of early retirement. Automatic Control, IEEE Transactions on, 49(3):409–419, 2004
- K. Tumer and D. Wolpert. A survey of collectives. In K. Tumer and D. Wolpert, editors, Collectives and the Design of Complex Systems, pages 1–42. Springer, 2004.
- Bai J. 2003. Inferential theory for factor models of large dimensions. Econometrica 71:135–71
- K. Boda and J. Filar. Time consistent dynamic risk measures. Mathematical Methods of Operations Research, 63(1):169–186, 2006
- Bottou L. 1998. Online learning and stochastic approximations. In On-Line Learning in Neural Networks, ed. D Saad, pp. 9–42. New York: ACM
- V. Mnih, K. Kavukcuoglu, D. Silver, A. Rusu, J. Veness, M. Bellemare, A. Graves, M. Riedmiller, A. Fidjeland, G. Ostrovski, S. Petersen, C. Beattie, A. Sadik, I. Antonoglou, H. King, D. Kumaran, D. Wierstra, S. Legg, and D. Hassabis. Human-level control through deep reinforcement learning. Nature, 518(7540):529–533, 02 2015.