AUC Score :
Short-term Tactic1 :
Dominant Strategy :
Time series to forecast n:
ML Model Testing : Modular Neural Network (Financial Sentiment Analysis)
Hypothesis Testing : Chi-Square
Surveillance : Major exchange and OTC
1Short-term revised.
2Time series is updated based on short-term trends.
Key Points
The Dow Jones U.S. Select Medical Equipment index is projected to experience moderate growth, fueled by ongoing technological advancements in medical devices and an aging global population driving increased healthcare demand. Expectation is that companies involved in diagnostic imaging, surgical instruments, and patient monitoring systems will show resilience. The index faces risks from potential supply chain disruptions, regulatory hurdles affecting product approvals, and heightened competition among medical device manufacturers, which could limit earnings growth or lead to increased volatility. Healthcare policy changes and potential economic slowdowns also pose significant risks.About Dow Jones U.S. Select Medical Equipment Index
The Dow Jones U.S. Select Medical Equipment Index serves as a benchmark designed to track the performance of publicly traded companies primarily involved in the medical equipment sector within the United States. These companies manufacture, distribute, and sell a broad range of medical devices and technologies used in healthcare settings, including hospitals, clinics, and laboratories. The index aims to represent a comprehensive overview of the medical equipment industry, reflecting the diverse range of products and services offered by its constituent companies.
The index is typically constructed using a market capitalization-weighted methodology, where the relative influence of each company is determined by its total market value. Periodic reviews are conducted to ensure the index accurately reflects the evolving landscape of the medical equipment sector, potentially involving additions or deletions of companies based on established criteria. Investment professionals and market participants frequently utilize this index as a tool for analyzing industry trends, evaluating investment opportunities, and assessing overall market performance within the medical equipment domain.
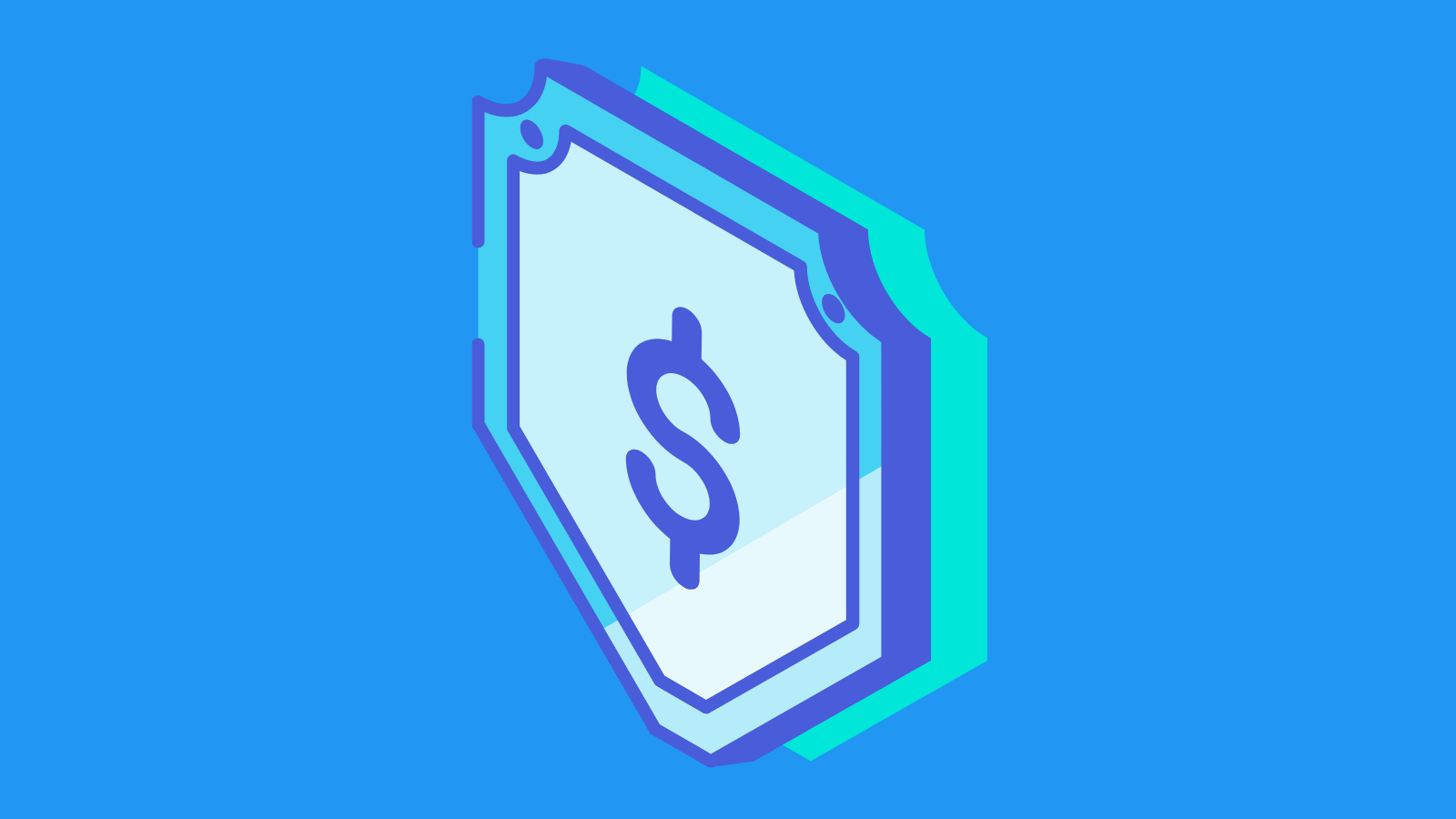
Dow Jones U.S. Select Medical Equipment Index Forecast Model
The development of a robust forecasting model for the Dow Jones U.S. Select Medical Equipment Index requires a multifaceted approach, leveraging the expertise of both data scientists and economists. Our primary objective is to predict the index's future movements, enabling informed investment decisions and strategic planning within the healthcare sector. The model incorporates a comprehensive set of predictor variables, classified into economic, financial, and industry-specific categories. Economic indicators include Gross Domestic Product (GDP) growth, inflation rates (CPI), interest rates (Federal Funds Rate), and unemployment figures. Financial metrics encompass corporate earnings reports from key medical equipment manufacturers, debt levels, and market capitalization data. Finally, industry-specific variables incorporate technological advancements in medical device development, regulatory changes (FDA approvals/rejections), healthcare spending trends, and demographic shifts such as aging populations. These variables are meticulously collected, cleaned, and preprocessed to ensure data quality and compatibility for model training.
The core of our forecasting model utilizes a hybrid approach, combining the strengths of time series analysis and machine learning techniques. For the time series component, we explore methods such as ARIMA (Autoregressive Integrated Moving Average) models to capture the inherent temporal dependencies within the index data, which is very important in the financial field. In parallel, we employ advanced machine learning algorithms, including Recurrent Neural Networks (RNNs), specifically Long Short-Term Memory (LSTM) networks, and Gradient Boosting Machines (GBMs), which is important for complex and nonlinear relationships among the predictor variables. These algorithms are chosen for their ability to handle non-linear relationships, identify complex patterns, and incorporate a broad range of features. The model's architecture involves training each algorithm independently, then combining their predictions using an ensemble method to improve overall accuracy and reduce the risk of overfitting.
Model evaluation and validation are crucial steps in ensuring the model's reliability. We employ a rigorous process that includes splitting the dataset into training, validation, and testing sets. Performance metrics, such as Mean Absolute Error (MAE), Root Mean Squared Error (RMSE), and R-squared, are used to assess the model's predictive accuracy and identify potential biases. The model's performance is continuously monitored and updated with new data to maintain its effectiveness and adaptability. Furthermore, we incorporate expert economic judgment and domain knowledge to interpret the model's outputs, ensuring the forecasts align with fundamental economic principles and industry insights. The model's output will be presented as probabilistic forecasts, providing a range of potential outcomes with associated confidence levels, thereby enabling users to make informed decisions.
ML Model Testing
n:Time series to forecast
p:Price signals of Dow Jones U.S. Select Medical Equipment index
j:Nash equilibria (Neural Network)
k:Dominated move of Dow Jones U.S. Select Medical Equipment index holders
a:Best response for Dow Jones U.S. Select Medical Equipment target price
For further technical information as per how our model work we invite you to visit the article below:
How do KappaSignal algorithms actually work?
Dow Jones U.S. Select Medical Equipment Index Forecast Strategic Interaction Table
Strategic Interaction Table Legend:
X axis: *Likelihood% (The higher the percentage value, the more likely the event will occur.)
Y axis: *Potential Impact% (The higher the percentage value, the more likely the price will deviate.)
Z axis (Grey to Black): *Technical Analysis%
Dow Jones U.S. Select Medical Equipment Index: Financial Outlook and Forecast
The Dow Jones U.S. Select Medical Equipment Index tracks the performance of companies involved in the development, manufacturing, and distribution of medical devices and equipment within the United States. These companies are crucial to the healthcare ecosystem, providing a broad range of products, from advanced diagnostic imaging systems and surgical instruments to life-sustaining devices and patient monitoring equipment. The sector is characterized by high barriers to entry, requiring significant capital investment in research and development, stringent regulatory approvals (primarily from the Food and Drug Administration), and complex manufacturing processes. The financial outlook for the index is significantly influenced by factors such as aging populations, the increasing prevalence of chronic diseases, advancements in medical technology, healthcare spending trends, and global economic conditions. The COVID-19 pandemic has had a mixed impact, accelerating demand for certain products like ventilators and diagnostics while simultaneously disrupting supply chains and delaying elective procedures. The sector's inherent resilience, driven by consistent demand for healthcare, often makes it attractive to investors seeking a degree of stability.
Several macroeconomic trends are expected to continue shaping the financial performance of the companies included in the index. Population aging in developed nations is a major driver, creating a greater need for medical devices and equipment to manage age-related illnesses. Furthermore, rising healthcare spending globally, particularly in emerging markets, presents significant growth opportunities. Technological innovation is also a key factor; advancements in areas like minimally invasive surgery, digital health, and personalized medicine are creating demand for innovative devices and equipment. Other variables include the impact of government regulations, such as approval processes and reimbursement policies, which can affect profitability and growth. Additionally, changes in currency exchange rates, trade policies, and geopolitical risks can influence the companies' revenue and operational costs. The ongoing integration of artificial intelligence and data analytics in medical devices is also poised to create significant avenues for growth. Mergers and acquisitions are also commonly observed activity that also affects the financial forecasts as well.
Looking ahead, the Dow Jones U.S. Select Medical Equipment Index is expected to exhibit solid growth, albeit with varying degrees of performance depending on specific sub-sectors and company characteristics. The continued expansion of global healthcare markets, coupled with technological innovation, will provide ample opportunities for growth. Companies specializing in areas with particularly strong tailwinds, such as robotic surgery, digital health solutions, and cardiovascular devices, are anticipated to outperform the broader market. The potential for increased healthcare spending driven by aging populations and the rise of chronic diseases creates a solid foundation. However, individual company performance can vary considerably. The strength of a company's product pipeline, its ability to navigate the regulatory landscape, its market positioning, and its management team's expertise will be crucial determinants of success. Diversified portfolios that are exposed to different types of medical device products are expected to offer better results than narrow portfolios that are concentrated on a specific product or technology.
Based on these factors, a positive outlook is forecasted for the Dow Jones U.S. Select Medical Equipment Index over the next five years. However, this forecast is subject to several risks. These include shifts in government healthcare policies, changes in reimbursement rates, supply chain disruptions, and increased competition. Economic downturns could impact healthcare spending. Delays in new product approvals from regulatory bodies can hurt revenue and growth, while rapid technological advances can lead to obsolescence. Other risks include intellectual property disputes, and the success and financial viability of smaller companies that can be acquired or have an initial public offering (IPO). Further risks are increasing labor costs, and the ability to mitigate the effects of inflation. Despite these risks, the underlying secular trends driving demand, coupled with ongoing innovation, suggest that the index will perform well, although investor should still practice due diligence.
Rating | Short-Term | Long-Term Senior |
---|---|---|
Outlook | Caa2 | B1 |
Income Statement | C | Baa2 |
Balance Sheet | C | Ba1 |
Leverage Ratios | B1 | Caa2 |
Cash Flow | Caa2 | Ba2 |
Rates of Return and Profitability | C | Caa2 |
*An aggregate rating for an index summarizes the overall sentiment towards the companies it includes. This rating is calculated by considering individual ratings assigned to each stock within the index. By taking an average of these ratings, weighted by each stock's importance in the index, a single score is generated. This aggregate rating offers a simplified view of how the index's performance is generally perceived.
How does neural network examine financial reports and understand financial state of the company?
References
- V. Borkar. A sensitivity formula for the risk-sensitive cost and the actor-critic algorithm. Systems & Control Letters, 44:339–346, 2001
- J. Filar, L. Kallenberg, and H. Lee. Variance-penalized Markov decision processes. Mathematics of Opera- tions Research, 14(1):147–161, 1989
- M. J. Hausknecht. Cooperation and Communication in Multiagent Deep Reinforcement Learning. PhD thesis, The University of Texas at Austin, 2016
- T. Shardlow and A. Stuart. A perturbation theory for ergodic Markov chains and application to numerical approximations. SIAM journal on numerical analysis, 37(4):1120–1137, 2000
- Keane MP. 2013. Panel data discrete choice models of consumer demand. In The Oxford Handbook of Panel Data, ed. BH Baltagi, pp. 54–102. Oxford, UK: Oxford Univ. Press
- Lai TL, Robbins H. 1985. Asymptotically efficient adaptive allocation rules. Adv. Appl. Math. 6:4–22
- Doudchenko N, Imbens GW. 2016. Balancing, regression, difference-in-differences and synthetic control methods: a synthesis. NBER Work. Pap. 22791