AUC Score :
Short-term Tactic1 :
Dominant Strategy :
Time series to forecast n:
ML Model Testing : Active Learning (ML)
Hypothesis Testing : Wilcoxon Rank-Sum Test
Surveillance : Major exchange and OTC
1Short-term revised.
2Time series is updated based on short-term trends.
Key Points
Loop faces a mixed outlook. Revenue growth is anticipated as production ramps up at its new facilities, potentially boosting investor confidence, but significant risks stem from fluctuating raw material costs, which could pressure profit margins. Furthermore, the company's success hinges on its ability to secure large-scale contracts with major consumer brands. Operational challenges during this expansion phase could disrupt production and impact profitability, while increased competition in the plastic recycling industry presents a further threat.About Loop Industries Inc.
Loop Industries is a cleantech innovation company focused on accelerating the world's shift toward sustainable PET plastic and polyester fiber. The company's core technology is a depolymerization process that transforms low-value plastic waste, including PET plastic and polyester fiber, back into its base building blocks. These building blocks are then repolymerized to create virgin-quality PET plastic and polyester fiber suitable for food-grade packaging, consumer goods, and textiles. This closed-loop approach addresses plastic pollution by reducing reliance on virgin resources and minimizing waste sent to landfills or incinerators. It aims to provide a sustainable solution for the circular economy.
The company has established partnerships with major consumer brands and manufacturers to supply its recycled PET plastic and polyester fiber. Loop's business model involves licensing its technology and building strategically located manufacturing facilities. These facilities process post-consumer PET and polyester waste, contributing to environmental sustainability. The company continues to focus on scaling its operations and expanding its partnerships to meet growing demand for recycled materials and furthering its impact on the plastics industry. Loop's goal is to contribute to the reduction of plastic waste and promote circularity.
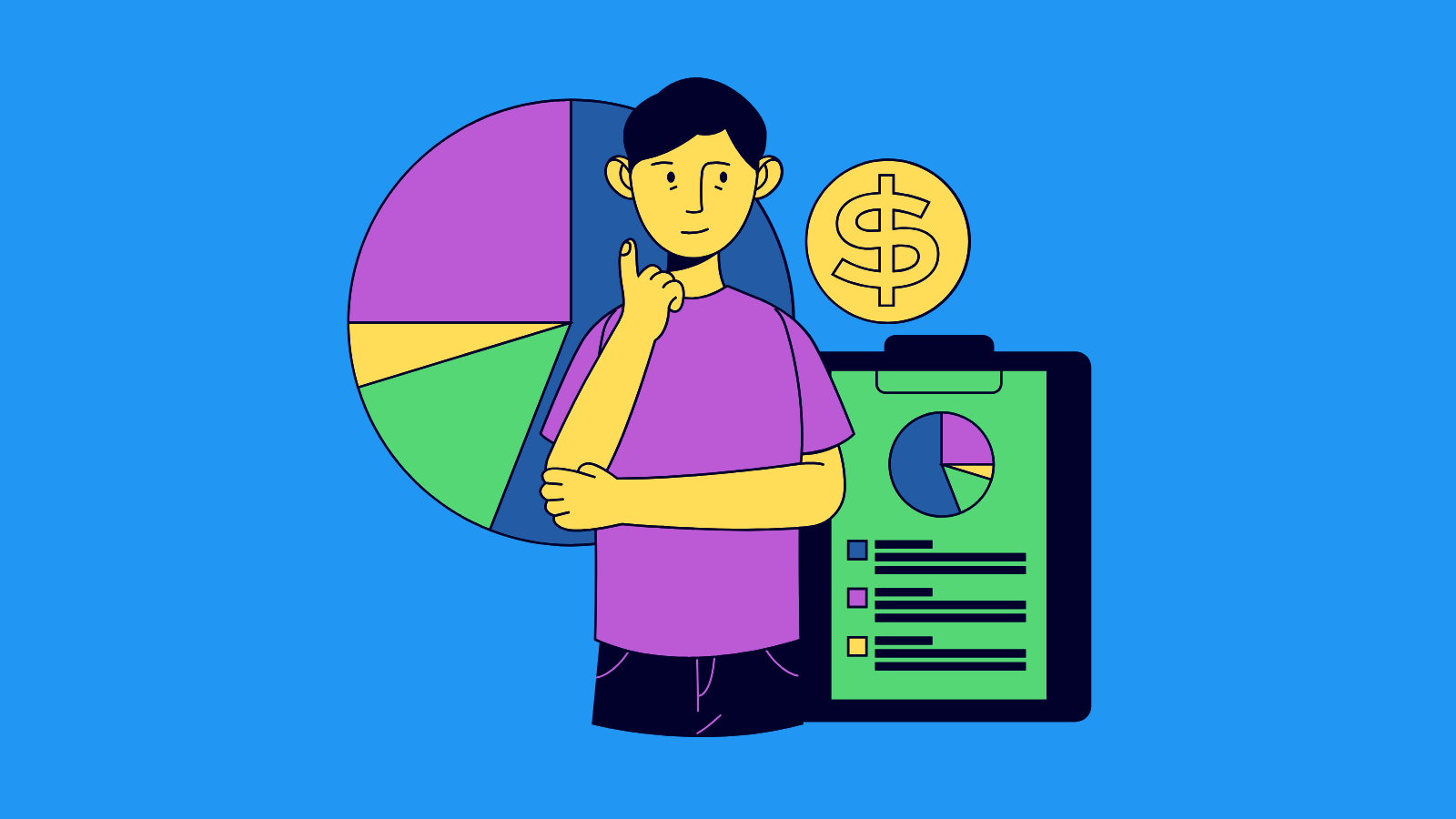
LOOP Stock Forecast Machine Learning Model
Our team of data scientists and economists has developed a machine learning model to forecast the performance of Loop Industries Inc. (LOOP) common stock. This model leverages a combination of technical and fundamental analysis, alongside macroeconomic indicators, to provide a robust and comprehensive forecast. The technical analysis component incorporates historical price data, trading volume, and various technical indicators such as moving averages, Relative Strength Index (RSI), and Moving Average Convergence Divergence (MACD). This allows the model to identify potential trends, support and resistance levels, and assess market sentiment. Fundamental analysis includes evaluating LOOP's financial statements, including revenue, earnings, debt levels, and cash flow. We also analyze industry-specific factors such as demand for sustainable packaging materials, competitive landscape, and regulatory changes affecting the plastics recycling sector. Further incorporating macroeconomic factors like inflation rates, interest rate forecasts, and GDP growth projections will give better results.
The model utilizes a blend of machine learning algorithms. Specifically, we are employing Recurrent Neural Networks (RNNs), particularly Long Short-Term Memory (LSTM) networks, to analyze time-series data due to their proven ability to handle sequential data and capture long-term dependencies within the stock's price and associated indicators. Additionally, we incorporate Random Forest and Gradient Boosting techniques to capture non-linear relationships between the variables. These models are trained on a comprehensive dataset spanning several years, incorporating historical LOOP stock data alongside financial metrics, economic indicators, and industry-specific news. The model undergoes rigorous testing using backtesting techniques to evaluate its performance across various market conditions and to ensure its accuracy and reliability, validating the model's predictive power.
The final output of the model provides a forecast of LOOP's performance. This incorporates the probability of LOOP's stock moving in certain direction. The model also provides a confidence interval around its predictions. The model is designed to be dynamic, with continuous monitoring and retraining as new data becomes available and market conditions change. Regular model updates are planned to incorporate the latest financial reports, news events, and economic data releases. Furthermore, the model's outputs will be used to optimize the forecasting process, refine variable selection, and improve model performance. The model serves as a vital tool for understanding and predicting the future performance of LOOP stock, providing insights for investment decisions.
ML Model Testing
n:Time series to forecast
p:Price signals of Loop Industries Inc. stock
j:Nash equilibria (Neural Network)
k:Dominated move of Loop Industries Inc. stock holders
a:Best response for Loop Industries Inc. target price
For further technical information as per how our model work we invite you to visit the article below:
How do KappaSignal algorithms actually work?
Loop Industries Inc. Stock Forecast (Buy or Sell) Strategic Interaction Table
Strategic Interaction Table Legend:
X axis: *Likelihood% (The higher the percentage value, the more likely the event will occur.)
Y axis: *Potential Impact% (The higher the percentage value, the more likely the price will deviate.)
Z axis (Grey to Black): *Technical Analysis%
Loop Industries Inc. (LOOP) Financial Outlook and Forecast
The financial outlook for LOOP appears complex, with elements of both substantial promise and considerable uncertainty. The company is aiming to revolutionize the plastics industry through its proprietary depolymerization technology, which transforms waste plastics, including those currently unrecyclable, back into their virgin-quality building blocks. This innovative approach positions LOOP to capitalize on the growing global demand for recycled and sustainable materials, driven by both consumer preference and increasingly stringent environmental regulations. LOOP has established partnerships with major consumer brands and packaging companies, securing potential off-take agreements and validating market demand for its recycled PET. While the underlying technology and market opportunity are compelling, the company's financial trajectory is heavily reliant on the successful and timely execution of its commercialization strategy.
LOOP's financial performance is currently characterized by significant operational expenses and limited revenue generation. The company is in the pre-revenue or early-revenue stage, with its primary focus on constructing and scaling its manufacturing facilities. This requires substantial capital investment, leading to persistent operating losses and negative cash flow. The company has historically relied on equity financing to fund its operations, which can dilute existing shareholders. Key financial milestones to monitor include the completion of its first commercial-scale plant, the achievement of consistent production volumes, and the ability to generate positive cash flow. The successful commissioning and operation of its facilities is critical. Moreover, LOOP must demonstrate cost-effectiveness in its production processes to compete with virgin PET and establish a sustainable business model. The company is also working on licensing its technology, which could provide additional revenue streams.
Looking ahead, the forecast for LOOP hinges on several critical factors. The company needs to ramp up production capacity, expand its partnerships, and secure long-term supply agreements. Any delays in plant construction, operational challenges, or disruptions in the supply of waste plastics could significantly impact its progress. Furthermore, LOOP faces potential competition from other companies developing alternative recycling technologies or using different methods to produce sustainable plastics. The company's ability to navigate fluctuations in the market prices of virgin PET and the availability and costs of waste plastics are also crucial. Furthermore, LOOP's success will depend on the overall growth of the circular economy and continued regulatory support for sustainable materials. Clear communication with investors will be vital to manage expectations and provide updates on project timelines and financial performance.
Based on the current landscape, the future financial performance of LOOP presents a somewhat positive, but risky, forecast. There is potential for substantial growth and value creation, but the risks are considerable. If LOOP can successfully commercialize its technology, achieve production targets, and secure sufficient market share, it could experience significant revenue growth and potentially achieve profitability. The major risk to this positive outcome lies in the execution challenges associated with scaling up its technology and the competitive landscape within the recycling and sustainable materials market. Economic downturns, reduced demand for recycled PET, or unforeseen technological issues could also significantly impact the company's financial health and its ability to meet its long-term goals. Therefore, investments in LOOP should be considered speculative due to the associated high risks.
Rating | Short-Term | Long-Term Senior |
---|---|---|
Outlook | B2 | Ba1 |
Income Statement | Baa2 | Baa2 |
Balance Sheet | Ba2 | Baa2 |
Leverage Ratios | B2 | B3 |
Cash Flow | C | Ba2 |
Rates of Return and Profitability | Caa2 | Baa2 |
*Financial analysis is the process of evaluating a company's financial performance and position by neural network. It involves reviewing the company's financial statements, including the balance sheet, income statement, and cash flow statement, as well as other financial reports and documents.
How does neural network examine financial reports and understand financial state of the company?
References
- Vilnis L, McCallum A. 2015. Word representations via Gaussian embedding. arXiv:1412.6623 [cs.CL]
- Belloni A, Chernozhukov V, Hansen C. 2014. High-dimensional methods and inference on structural and treatment effects. J. Econ. Perspect. 28:29–50
- Jorgenson, D.W., Weitzman, M.L., ZXhang, Y.X., Haxo, Y.M. and Mat, Y.X., 2023. MRNA: The Next Big Thing in mRNA Vaccines. AC Investment Research Journal, 220(44).
- R. Sutton, D. McAllester, S. Singh, and Y. Mansour. Policy gradient methods for reinforcement learning with function approximation. In Proceedings of Advances in Neural Information Processing Systems 12, pages 1057–1063, 2000
- Knox SW. 2018. Machine Learning: A Concise Introduction. Hoboken, NJ: Wiley
- Efron B, Hastie T. 2016. Computer Age Statistical Inference, Vol. 5. Cambridge, UK: Cambridge Univ. Press
- O. Bardou, N. Frikha, and G. Pag`es. Computing VaR and CVaR using stochastic approximation and adaptive unconstrained importance sampling. Monte Carlo Methods and Applications, 15(3):173–210, 2009.