AUC Score :
Short-term Tactic1 :
Dominant Strategy :
Time series to forecast n:
ML Model Testing : Transfer Learning (ML)
Hypothesis Testing : Paired T-Test
Surveillance : Major exchange and OTC
1Short-term revised.
2Time series is updated based on short-term trends.
Key Points
LiveRamp is projected to experience moderate growth in its core data connectivity and identity resolution services, fueled by ongoing demand for privacy-focused advertising solutions and increasing enterprise adoption of its platform. **A key prediction is continued expansion into international markets, potentially accelerating revenue growth, but this hinges on successful adaptation to local data privacy regulations and competition.** Risk factors include heightened competition from larger tech companies and specialized data providers, potential economic downturns affecting advertising budgets, and evolving regulatory landscapes, such as shifts in data privacy legislation. **The company faces the risk of slowing growth if it fails to innovate and adapt to new advertising technologies, particularly in areas like artificial intelligence.**About LiveRamp Holdings
LiveRamp (RAMP) is a leading data enablement company that helps brands and their partners transform data into meaningful connections. Founded in 2011, the company provides a comprehensive suite of solutions designed to improve customer experiences and drive business outcomes. Its core offering revolves around connecting people, data, and applications to enable seamless data interoperability across the digital ecosystem. LiveRamp's platform facilitates identity resolution, allowing businesses to connect disparate customer data sources and gain a unified view of their audiences.
The company's services cater to a wide range of industries, including advertising, retail, and financial services. LiveRamp empowers its clients to personalize customer interactions, optimize marketing campaigns, and measure advertising effectiveness. By focusing on privacy-conscious data practices and maintaining robust security measures, LiveRamp helps its clients navigate the complexities of data privacy regulations and build trust with their customers. The company's continuous innovation and strategic partnerships strengthen its market position and expand its capabilities within the evolving data landscape.
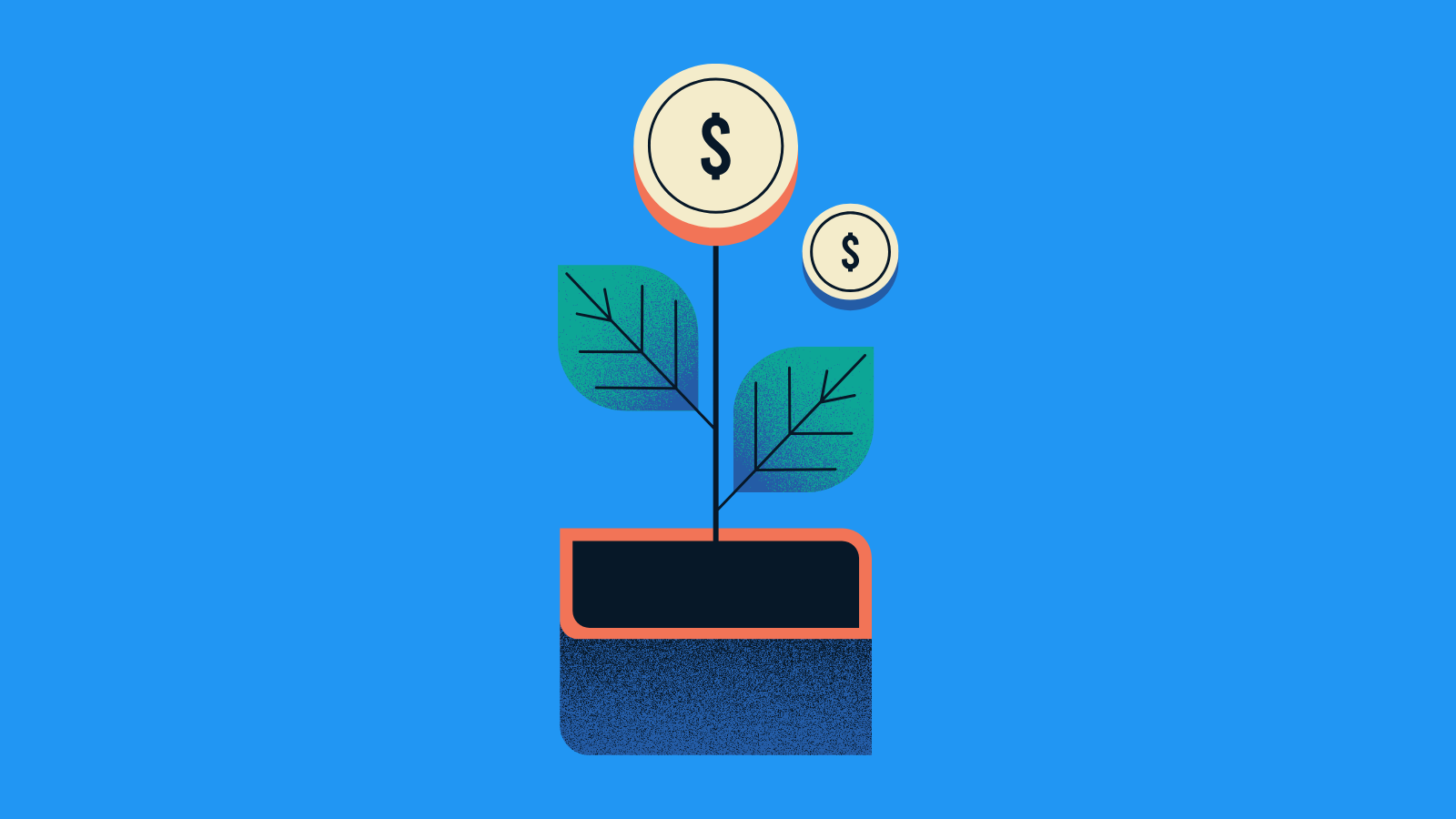
RAMP Stock Forecast Machine Learning Model
Our team of data scientists and economists has developed a machine learning model to forecast the performance of LiveRamp Holdings Inc. (RAMP) common stock. The core of our approach centers on a robust time-series analysis, employing a variety of algorithms to capture the complex dynamics inherent in financial markets. We utilize a diverse dataset encompassing historical stock prices, trading volumes, financial statements (including revenue, earnings, and cash flow), macroeconomic indicators (such as GDP growth, inflation rates, and interest rates), and industry-specific metrics relevant to the advertising technology sector. Furthermore, we incorporate sentiment analysis derived from news articles, social media, and analyst reports to gauge market perception and identify potential shifts in investor behavior. The data is preprocessed to handle missing values, remove outliers, and ensure data quality, followed by feature engineering to create new variables that may improve predictive accuracy.
The model architecture integrates several machine learning techniques, including Recurrent Neural Networks (RNNs) with Long Short-Term Memory (LSTM) units, to effectively capture the temporal dependencies within the time-series data. We also employ Gradient Boosting Machines (GBMs) and Random Forests to incorporate the non-linear relationships between the variables. The selection of the most suitable algorithm for forecasting and its optimal parameters is achieved through a rigorous process of model training, validation, and testing using a held-out dataset. Key performance metrics, such as Mean Absolute Error (MAE), Root Mean Squared Error (RMSE), and R-squared, are used to evaluate the predictive power of the model across various time horizons. Regularization techniques, such as L1 and L2 regularization, are applied to prevent overfitting and enhance generalization performance. Additionally, ensemble methods are employed to combine predictions from multiple models, mitigating the risk associated with individual model biases and enhancing overall forecast accuracy.
The model is designed to provide forecasts within a defined timeframe, allowing for adjustments based on emerging market conditions. A comprehensive backtesting process is implemented to assess the model's performance over extended historical periods and to identify potential weaknesses. Moreover, we are continually improving the model by regularly retraining it with updated data and incorporating new features. The model also integrates a risk assessment framework to quantify the uncertainty associated with the forecasts and to alert users about potential volatility. This includes confidence intervals for the predictions. The model output, presented in a user-friendly format, provides insights into factors driving the forecast and allows for scenario analysis to understand the potential impacts of different economic or company-specific events. This comprehensive approach ensures that the model remains a valuable tool for investment decisions and risk management related to RAMP common stock.
```
ML Model Testing
n:Time series to forecast
p:Price signals of LiveRamp Holdings stock
j:Nash equilibria (Neural Network)
k:Dominated move of LiveRamp Holdings stock holders
a:Best response for LiveRamp Holdings target price
For further technical information as per how our model work we invite you to visit the article below:
How do KappaSignal algorithms actually work?
LiveRamp Holdings Stock Forecast (Buy or Sell) Strategic Interaction Table
Strategic Interaction Table Legend:
X axis: *Likelihood% (The higher the percentage value, the more likely the event will occur.)
Y axis: *Potential Impact% (The higher the percentage value, the more likely the price will deviate.)
Z axis (Grey to Black): *Technical Analysis%
LiveRamp's Financial Outlook and Forecast
LiveRamp (RAMP) is a prominent player in the data enablement and identity resolution space, offering services that help businesses connect their data to improve customer experiences and marketing efforts. The company operates within a rapidly evolving digital advertising and marketing technology landscape. Its financial outlook is strongly linked to its ability to navigate industry shifts, maintain technological competitiveness, and capitalize on growing demand for data-driven solutions. LiveRamp's primary revenue streams include subscription-based services, data integration, and platform solutions. The company's strategy centers around its IdentityLink platform, a crucial element for connecting disparate data sources and enabling cross-channel marketing. Furthermore, LiveRamp is investing in areas such as data clean rooms and privacy-enhancing technologies to address increasing concerns over data privacy and evolving regulatory frameworks. The ability to secure and retain major clients is vital for LiveRamp's long-term financial health. Major partnerships with key advertising platforms and data providers are also essential for expanding its data network and service offerings.
Analysis of recent financial performance suggests a mixed picture. Revenue growth has shown fluctuations, impacted by industry-wide trends and the timing of larger deals. LiveRamp's profitability has been affected by significant investments in technology and research and development, as well as the cost of sales and marketing. However, the company's focus on high-margin subscription revenue and enterprise clients provides a degree of stability and potential for long-term growth. Key financial indicators to watch include customer acquisition cost (CAC), customer lifetime value (CLTV), and renewal rates. The company's cash flow is a crucial metric to be monitored, especially in relation to LiveRamp's long-term capital investments. Moreover, LiveRamp's success will depend on its ability to retain and attract talent, particularly in the fields of engineering, data science, and sales. The strength of its balance sheet, including the level of debt and cash reserves, is also an important determinant of financial flexibility and ability to withstand economic downturns or industry disruptions.
Looking forward, LiveRamp's forecast is influenced by several factors, including the growth rate of the digital advertising market, the adoption of data privacy regulations, and the overall economic climate. The increasing importance of first-party data and the ongoing deprecation of third-party cookies represent both challenges and opportunities for LiveRamp. The company's ability to deliver value by helping businesses collect, manage, and activate their first-party data will be a key determinant of future success. In addition, LiveRamp is facing increased competition from established players and emerging startups in the data and marketing technology sector. Acquisitions and strategic partnerships may become more critical in consolidating its market position and expanding its service offerings. Investments in international expansion could also create further growth opportunities. It is likely that the company will continue to innovate and introduce new products to address the evolving needs of its customer base and stay ahead of its competitors.
Based on current trends and the company's strategic direction, the financial forecast for LiveRamp is cautiously positive. The company's focus on privacy-enhancing technologies and its position as a data enablement leader make it well-positioned for long-term growth. However, several risks could impact this outlook. These risks include the potential for greater-than-expected economic slowdowns that could negatively impact marketing spending, increased competition, the inability to innovate at pace with rapid industry changes, and the potential for unfavorable changes in data privacy regulations. Failure to maintain strong partnerships and retain key clients is also a significant risk factor. The company may face headwinds related to inflation or supply chain issues. These risks could lead to lower revenue growth, reduced profitability, and pressure on the company's valuation. Therefore, careful monitoring of the industry landscape, the competitive environment, and the company's execution is crucial for investors.
Rating | Short-Term | Long-Term Senior |
---|---|---|
Outlook | Ba3 | B2 |
Income Statement | B3 | B2 |
Balance Sheet | Baa2 | Ba3 |
Leverage Ratios | Baa2 | B2 |
Cash Flow | Ba2 | C |
Rates of Return and Profitability | B3 | Caa2 |
*Financial analysis is the process of evaluating a company's financial performance and position by neural network. It involves reviewing the company's financial statements, including the balance sheet, income statement, and cash flow statement, as well as other financial reports and documents.
How does neural network examine financial reports and understand financial state of the company?
References
- Mnih A, Kavukcuoglu K. 2013. Learning word embeddings efficiently with noise-contrastive estimation. In Advances in Neural Information Processing Systems, Vol. 26, ed. Z Ghahramani, M Welling, C Cortes, ND Lawrence, KQ Weinberger, pp. 2265–73. San Diego, CA: Neural Inf. Process. Syst. Found.
- Allen, P. G. (1994), "Economic forecasting in agriculture," International Journal of Forecasting, 10, 81–135.
- Mazumder R, Hastie T, Tibshirani R. 2010. Spectral regularization algorithms for learning large incomplete matrices. J. Mach. Learn. Res. 11:2287–322
- Miller A. 2002. Subset Selection in Regression. New York: CRC Press
- Breusch, T. S. A. R. Pagan (1979), "A simple test for heteroskedasticity and random coefficient variation," Econometrica, 47, 1287–1294.
- Athey S. 2017. Beyond prediction: using big data for policy problems. Science 355:483–85
- D. Bertsekas. Dynamic programming and optimal control. Athena Scientific, 1995.