AUC Score :
Short-term Tactic1 :
Dominant Strategy :
Time series to forecast n:
ML Model Testing : Modular Neural Network (Financial Sentiment Analysis)
Hypothesis Testing : Chi-Square
Surveillance : Major exchange and OTC
1Short-term revised.
2Time series is updated based on short-term trends.
Key Points
TR/CC CRB Lean Hogs index is predicted to exhibit a period of moderate volatility. The index may experience a modest upward trend, potentially driven by seasonal demand fluctuations and evolving supply dynamics. However, this prediction carries risks. A contraction in global economic conditions or unforeseen disease outbreaks among hog populations could lead to significant price declines. Moreover, fluctuations in feed costs and export demand present further downside risks.About TR/CC CRB Lean Hogs Index
The TR/CC CRB Lean Hogs index provides a benchmark for the price performance of lean hog futures contracts. This index is designed to reflect the overall trend in the lean hog market, offering a comprehensive view of price movements for this specific commodity. It's often used by investors and traders as a tool to assess market conditions and to make informed decisions regarding investment strategies related to the pork industry. The index incorporates a rules-based methodology to select and weigh its components, aiming to provide a representative and tradable measure of the lean hog futures market.
The TR/CC CRB Lean Hogs index plays a vital role in the financial markets by enabling various investment opportunities. It can be employed to track and analyze market volatility, assess hedging strategies, or to create investment products that provide exposure to the lean hog market. Moreover, the index's construction and regular maintenance ensure it continues to reflect the dynamics of the lean hog futures market, offering valuable insights for market participants and financial professionals alike.
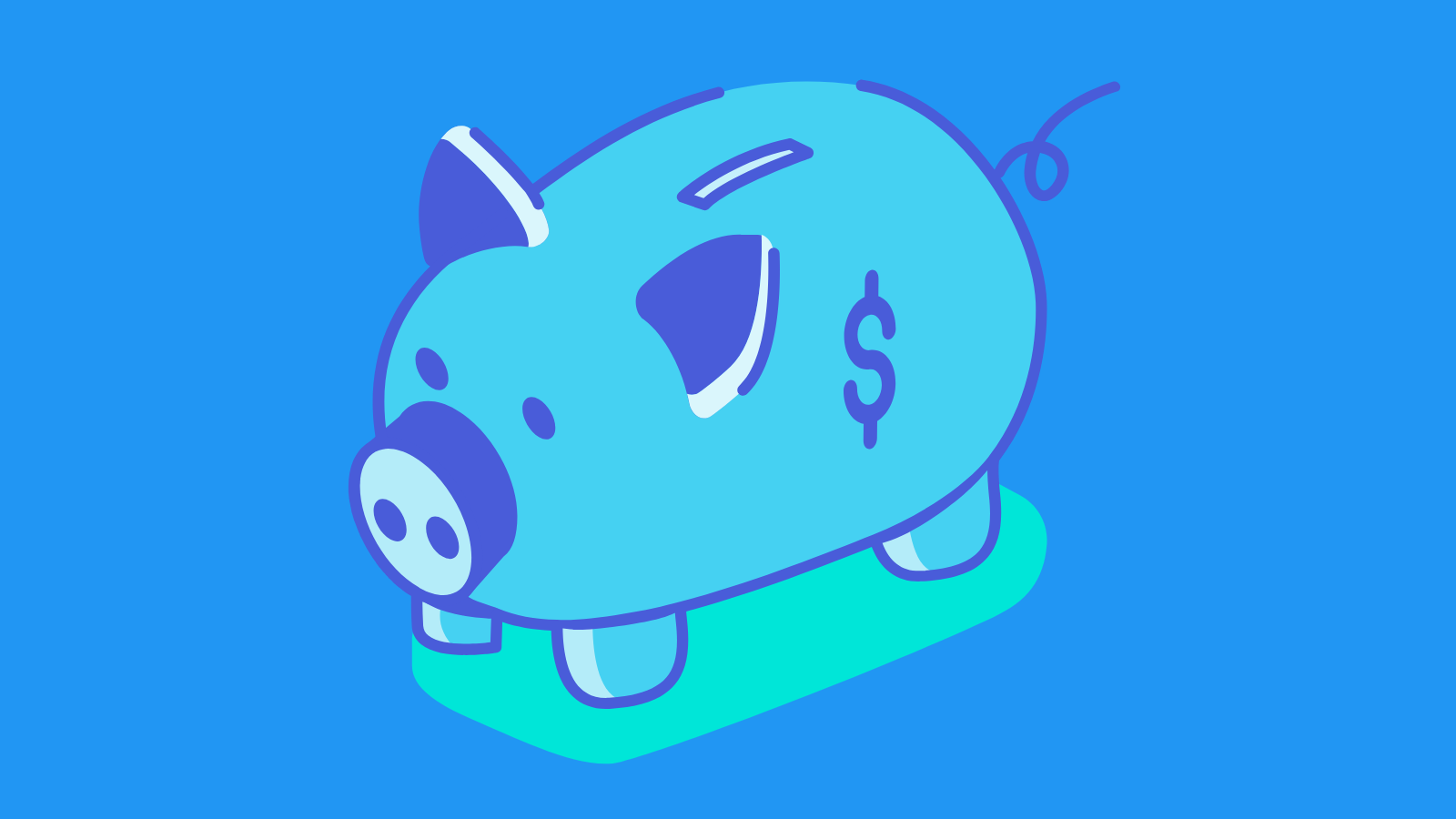
TR/CC CRB Lean Hogs Index Forecasting Model
Our team of data scientists and economists has developed a machine learning model to forecast the TR/CC CRB Lean Hogs index. The model leverages a diverse range of economic and market data to predict future index values. Key input features include historical price data (lagged values of the index itself), supply-side indicators such as hog inventory levels, slaughter rates, and feed costs (corn and soybean prices). We also incorporate demand-side factors, including measures of consumer demand for pork (e.g., retail pork prices), export data, and macroeconomic variables like GDP growth and consumer confidence indices. Furthermore, external shocks such as disease outbreaks (e.g., African Swine Fever) and government regulations are considered. The model is trained on a historical dataset, incorporating data spanning multiple decades to capture long-term trends and cyclical patterns.
The core of our model employs a hybrid approach combining the strengths of various machine learning algorithms. We primarily utilize an ensemble method, specifically a Gradient Boosting Regressor, which is well-suited for time-series forecasting and can effectively handle non-linear relationships in the data. The Gradient Boosting model is enhanced by a careful selection of features, feature engineering techniques, and hyperparameter optimization. To address potential autocorrelation in the time series data, we integrate ARIMA (Autoregressive Integrated Moving Average) modeling to capture short-term dependencies. Model validation is rigorous, including holdout validation and rolling origin validation to assess predictive accuracy across time. This helps ensure robustness and reliability. Model performance is evaluated using standard metrics, including Mean Absolute Error (MAE), Root Mean Squared Error (RMSE), and R-squared.
The model's output provides a forecast for the TR/CC CRB Lean Hogs index, typically for periods ranging from one week to several months ahead. The model also provides confidence intervals associated with the forecasts, which allow users to gauge the uncertainty of each prediction. These forecasts are regularly updated with new data. The model can be used by market participants, including commodity traders, agricultural producers, and policymakers, for informed decision-making. It is a tool to provide insights, but it does not substitute human judgment. Moreover, we are committed to continuous improvement and will periodically update the model to incorporate new data sources, refine existing features, and optimize algorithm parameters to ensure the model's accuracy and adaptability to evolving market dynamics.
```ML Model Testing
n:Time series to forecast
p:Price signals of TR/CC CRB Lean Hogs index
j:Nash equilibria (Neural Network)
k:Dominated move of TR/CC CRB Lean Hogs index holders
a:Best response for TR/CC CRB Lean Hogs target price
For further technical information as per how our model work we invite you to visit the article below:
How do KappaSignal algorithms actually work?
TR/CC CRB Lean Hogs Index Forecast Strategic Interaction Table
Strategic Interaction Table Legend:
X axis: *Likelihood% (The higher the percentage value, the more likely the event will occur.)
Y axis: *Potential Impact% (The higher the percentage value, the more likely the price will deviate.)
Z axis (Grey to Black): *Technical Analysis%
TR/CC CRB Lean Hogs Index: Financial Outlook and Forecast
The TR/CC CRB Lean Hogs Index, a commodity index tracking the price of lean hogs, offers a glimpse into the dynamics of the pork industry and provides valuable insights for investors and agricultural stakeholders. The outlook for lean hog prices is intricately linked to several key factors. These include the availability of feed (primarily corn and soybean meal), disease outbreaks (such as African Swine Fever, or ASF), domestic and international demand, and seasonal fluctuations in production. Changes in any of these areas can significantly impact the index's performance. Furthermore, government regulations, trade policies, and global economic conditions also play crucial roles in determining the future trajectory of hog prices. Understanding these elements and their interplay is essential to formulating a comprehensive financial outlook for the lean hogs market.
Analyzing the current environment, the hog market is significantly influenced by factors beyond just supply and demand. The global economic outlook and the health of the Chinese economy, a major consumer of pork, are critical determinants. Strong demand from China could boost prices, while economic slowdowns there or elsewhere can dampen it. Feed costs represent a constant expense, and volatility in these markets directly impacts profitability for hog farmers, subsequently influencing supply and price. The health status of hog populations, especially the potential spread of ASF, remains a significant threat, with outbreaks potentially leading to drastic price swings. Changes in international trade policies, for instance, tariffs or trade agreements, can influence the volume of pork exports and imports, thereby impacting domestic hog prices. Therefore, market participants need to monitor global events and factors impacting both supply and demand to make informed investment decisions.
Forecasting the TR/CC CRB Lean Hogs Index requires a nuanced understanding of market trends and a disciplined approach to risk assessment. Over the short to medium term, analysts are carefully watching the USDA's reports for its updates on hog inventories, slaughter rates, and pork production numbers. Changes in U.S. Department of Agriculture (USDA) reports, global trade negotiations, and consumer preferences shape the supply and demand dynamics. Examining seasonality is essential, as hog prices often exhibit predictable seasonal patterns, with peaks and troughs tied to consumer demand and production cycles. Additionally, investor sentiment and speculative activity in the futures market can amplify price movements. Therefore, a forecast should integrate both fundamental analysis (based on supply/demand factors) and technical analysis (based on price trends and market sentiment) to make a reasoned estimate.
Considering the various factors, a cautiously optimistic outlook seems appropriate for the TR/CC CRB Lean Hogs Index. Demand is expected to remain relatively stable given that pork is an essential protein source. However, the primary risks to this prediction involve unexpected disease outbreaks, particularly ASF, which could significantly disrupt supply chains and lead to higher price volatility. Unforeseen shifts in global trade policies could impede access to key export markets or increase the cost of imported feed. Further, any major economic downturn, particularly in key pork-consuming nations, could diminish demand and exert downward pressure on prices. The combination of relatively stable demand, the importance of risk management to address supply chain disruptions, and the continuing need to monitor economic trends underscore the need for a disciplined approach to investing in the lean hog market.
Rating | Short-Term | Long-Term Senior |
---|---|---|
Outlook | Ba3 | B2 |
Income Statement | Ba3 | Caa2 |
Balance Sheet | B1 | Caa2 |
Leverage Ratios | Caa2 | C |
Cash Flow | B2 | B1 |
Rates of Return and Profitability | Baa2 | B1 |
*An aggregate rating for an index summarizes the overall sentiment towards the companies it includes. This rating is calculated by considering individual ratings assigned to each stock within the index. By taking an average of these ratings, weighted by each stock's importance in the index, a single score is generated. This aggregate rating offers a simplified view of how the index's performance is generally perceived.
How does neural network examine financial reports and understand financial state of the company?
References
- Imbens GW, Lemieux T. 2008. Regression discontinuity designs: a guide to practice. J. Econom. 142:615–35
- Bottomley, P. R. Fildes (1998), "The role of prices in models of innovation diffusion," Journal of Forecasting, 17, 539–555.
- Wan M, Wang D, Goldman M, Taddy M, Rao J, et al. 2017. Modeling consumer preferences and price sensitiv- ities from large-scale grocery shopping transaction logs. In Proceedings of the 26th International Conference on the World Wide Web, pp. 1103–12. New York: ACM
- Jorgenson, D.W., Weitzman, M.L., ZXhang, Y.X., Haxo, Y.M. and Mat, Y.X., 2023. Can Neural Networks Predict Stock Market?. AC Investment Research Journal, 220(44).
- R. Rockafellar and S. Uryasev. Conditional value-at-risk for general loss distributions. Journal of Banking and Finance, 26(7):1443 – 1471, 2002
- Scott SL. 2010. A modern Bayesian look at the multi-armed bandit. Appl. Stoch. Models Bus. Ind. 26:639–58
- Bamler R, Mandt S. 2017. Dynamic word embeddings via skip-gram filtering. In Proceedings of the 34th Inter- national Conference on Machine Learning, pp. 380–89. La Jolla, CA: Int. Mach. Learn. Soc.