AUC Score :
Short-term Tactic1 :
Dominant Strategy :
Time series to forecast n:
ML Model Testing : Modular Neural Network (News Feed Sentiment Analysis)
Hypothesis Testing : Stepwise Regression
Surveillance : Major exchange and OTC
1Short-term revised.
2Time series is updated based on short-term trends.
Key Points
Kornit Digital faces a mixed outlook. Increased adoption of digital textile printing, driven by demand for sustainable solutions and on-demand production, strongly favors the company's growth. Kornit Digital is likely to benefit from expanding into new geographic markets and further innovation in its product portfolio. However, the company may face challenges in the form of supply chain disruptions, economic slowdowns impacting discretionary spending on fashion, and increased competition from both established players and emerging firms in the digital printing market. Currency fluctuations and potential geopolitical instability could also introduce volatility. Ultimately, while the long-term trends are favorable, the company's performance could be subject to market and competitive pressures that should be closely monitored.About Kornit Digital
Kornit Digital Ltd. (KRNT) is a global company that designs, develops, and markets digital printing solutions for the textile industry. Their technology focuses on direct-to-garment (DTG), direct-to-fabric (DTF), and roll-to-roll printing applications. KRNT's systems are used for on-demand production, enabling businesses to personalize apparel, home décor, and other textile products with high-quality, sustainable printing methods. The company's innovative approach aims to transform the traditional textile manufacturing process, reducing waste and enabling localized production.
KRNT's customer base includes apparel brands, contract printers, and online retailers, who benefit from the ability to offer customized products in shorter runs. The company provides integrated solutions, including printers, consumables (inks and pretreatment liquids), software, and services. They emphasize sustainability, offering eco-friendly printing solutions that minimize water usage and waste. KRNT's focus on technological advancements and industry partnerships continues to expand its presence and market share in the growing digital textile printing sector.
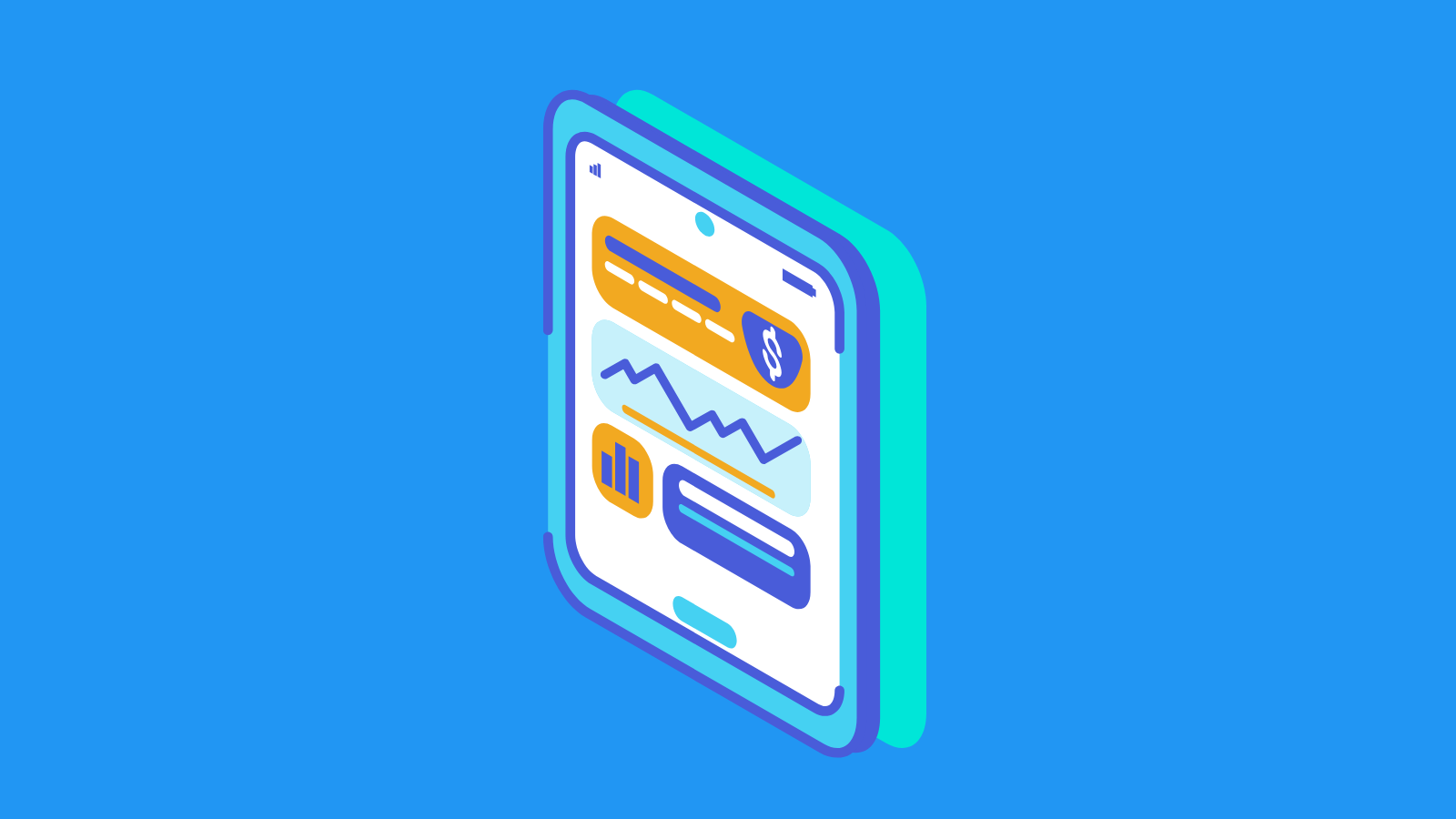
KRNT Stock Forecasting Model
Our multidisciplinary team has developed a comprehensive machine learning model designed to forecast the performance of Kornit Digital Ltd. Ordinary Shares (KRNT). This model integrates a variety of data sources and employs sophisticated analytical techniques to generate predictions. Core components include: (1) a time-series analysis module, incorporating historical KRNT price data, trading volumes, and volatility metrics. (2) A fundamental analysis component that extracts and analyzes financial statements (balance sheets, income statements, cash flow statements), key performance indicators (KPIs), and industry-specific data. This data is sourced from reputable financial data providers. (3) A sentiment analysis module that monitors news articles, social media, and financial reports to assess market sentiment and gauge investor perception. (4) We use both supervised and unsupervised learning techniques, including Recurrent Neural Networks (RNNs), Support Vector Machines (SVMs), and ensemble methods like Random Forests, to provide predictions.
The model architecture is designed for dynamic adaptation and continuous improvement. Feature engineering plays a crucial role, transforming raw data into relevant inputs for the machine learning algorithms. Feature selection techniques are employed to identify the most influential variables driving KRNT stock performance. The time-series module incorporates lagged variables, moving averages, and exponential smoothing to capture temporal dependencies. The fundamental analysis module extracts relevant financial ratios and growth rates. The sentiment analysis component employs natural language processing (NLP) techniques, including sentiment scoring and topic modeling, to quantify market sentiment. The output from each module is combined with the final forecast, using a weighted averaging approach determined by model performance. The model's performance will be evaluated against various metrics, including mean absolute error (MAE), root mean squared error (RMSE), and directional accuracy, on a rolling basis.
This model allows us to forecast the likely movements of KRNT stocks. The model's outputs include both point estimates and confidence intervals. A rigorous backtesting methodology is employed to validate the model's accuracy and reliability. The model will be regularly retrained with new data and the weights and parameters for each component are regularly updated to account for changes in market conditions and data trends. Sensitivity analysis is performed to understand how the model's predictions respond to changes in input variables. This allows us to create potential investment strategies for KRNT stocks to assist stakeholders with informed decisions. We emphasize that no model can guarantee future results and the forecasts are provided for informational purposes only.
```ML Model Testing
n:Time series to forecast
p:Price signals of Kornit Digital stock
j:Nash equilibria (Neural Network)
k:Dominated move of Kornit Digital stock holders
a:Best response for Kornit Digital target price
For further technical information as per how our model work we invite you to visit the article below:
How do KappaSignal algorithms actually work?
Kornit Digital Stock Forecast (Buy or Sell) Strategic Interaction Table
Strategic Interaction Table Legend:
X axis: *Likelihood% (The higher the percentage value, the more likely the event will occur.)
Y axis: *Potential Impact% (The higher the percentage value, the more likely the price will deviate.)
Z axis (Grey to Black): *Technical Analysis%
Kornit Digital Financial Outlook and Forecast
Kornit's business model is centered on providing digital textile printing solutions, a market experiencing ongoing expansion. The company offers advanced printing systems, consumables, and software that enable on-demand production for the fashion, apparel, and home décor industries. Their direct-to-garment (DTG) and direct-to-fabric (DTF) technologies are designed to meet the growing demand for sustainable and personalized textile production. The Company's focus on innovation, particularly with its eco-friendly pigment-based inks and waterless printing processes, aligns well with current market trends. This emphasis on sustainability could give the company a competitive edge as brands increasingly prioritize environmentally responsible practices.
Financial performance is closely tied to the overall health of the textile and apparel industries. The Company has seen revenues fluctuate, with periods of strong growth followed by more moderate performance, affected by macroeconomic conditions. The strategic expansion of their customer base to include both large-scale manufacturers and smaller, on-demand print shops provides some diversification and stability. Furthermore, the company's recurring revenue streams from consumables and service contracts are crucial. Investing in research and development (R&D) to maintain its technological lead is vital for long-term success. Keeping pace with technological developments and integrating its offerings into new product areas will be crucial to maintaining its market standing.
The company's outlook depends on a multitude of factors. Demand for personalized and on-demand textile production is expected to grow, giving a positive long-term outlook. Investments in R&D should yield new and improved products, driving future revenue growth. The company's capacity to manage its supply chain and production costs will be critical. Strong partnerships with key suppliers and the ability to maintain efficient inventory management are essential. Effective marketing and sales strategies are needed to penetrate new markets and reinforce relationships with existing customers. Also, the success of its expansion into new market segments, like sustainable fashion and industrial applications, may determine financial outcomes.
Overall, the company's future looks promising. The continued growth of digital textile printing and its commitment to eco-friendly technologies indicate potential for revenue and profit growth. The primary risk to this positive outlook is the volatile nature of the global economy. Furthermore, rising raw material costs, supply chain disruptions, and intensified market competition may negatively impact financial results. Also, technological innovation and competitive pressure from established players and new entrants represent additional downside risks. Successful execution of the company's strategic plans and its adaptability to changing market dynamics will ultimately determine its success.
Rating | Short-Term | Long-Term Senior |
---|---|---|
Outlook | B2 | B1 |
Income Statement | Caa2 | Caa2 |
Balance Sheet | Caa2 | B1 |
Leverage Ratios | B1 | Baa2 |
Cash Flow | B1 | Caa2 |
Rates of Return and Profitability | Caa2 | B3 |
*Financial analysis is the process of evaluating a company's financial performance and position by neural network. It involves reviewing the company's financial statements, including the balance sheet, income statement, and cash flow statement, as well as other financial reports and documents.
How does neural network examine financial reports and understand financial state of the company?
References
- M. Petrik and D. Subramanian. An approximate solution method for large risk-averse Markov decision processes. In Proceedings of the 28th International Conference on Uncertainty in Artificial Intelligence, 2012.
- Imai K, Ratkovic M. 2013. Estimating treatment effect heterogeneity in randomized program evaluation. Ann. Appl. Stat. 7:443–70
- Kallus N. 2017. Balanced policy evaluation and learning. arXiv:1705.07384 [stat.ML]
- Swaminathan A, Joachims T. 2015. Batch learning from logged bandit feedback through counterfactual risk minimization. J. Mach. Learn. Res. 16:1731–55
- Bai J. 2003. Inferential theory for factor models of large dimensions. Econometrica 71:135–71
- Hornik K, Stinchcombe M, White H. 1989. Multilayer feedforward networks are universal approximators. Neural Netw. 2:359–66
- D. Bertsekas and J. Tsitsiklis. Neuro-dynamic programming. Athena Scientific, 1996.