AUC Score :
Short-term Tactic1 :
Dominant Strategy :
Time series to forecast n:
ML Model Testing : Transfer Learning (ML)
Hypothesis Testing : Paired T-Test
Surveillance : Major exchange and OTC
1Short-term revised.
2Time series is updated based on short-term trends.
Key Points
IFRX may experience significant volatility due to its reliance on a single drug candidate, vilobelimab, and its ongoing clinical trials. Successful trial results, particularly for indications with high unmet medical needs, could lead to substantial share price appreciation. Conversely, clinical trial failures, regulatory setbacks, or unexpected adverse events could trigger dramatic declines. Further risks include competition from established pharmaceutical companies developing similar treatments and the company's financial position, which may require additional funding through dilutive offerings. The company's ability to secure partnerships and successfully commercialize vilobelimab are critical factors for its future prospects.About InflaRx N.V.
InflaRx N.V. is a clinical-stage biopharmaceutical company focused on the development and commercialization of therapeutics to treat severe inflammation and related diseases. Its core technology centers on the inhibition of complement factor C5a, a potent inflammatory mediator implicated in a range of serious illnesses. The company is primarily engaged in research and development activities with the aim of bringing novel therapies to patients suffering from unmet medical needs in areas such as autoimmune diseases and infectious diseases.
InflaRx's clinical programs are investigating the efficacy and safety of its lead drug candidate, vilobelimab, in various indications. The company strives to advance its pipeline of innovative therapeutic approaches through clinical trials and strategic collaborations. InflaRx aims to address significant market opportunities by targeting inflammatory pathways with the potential to provide better treatment options for patients impacted by severe conditions. Regulatory approvals are a key part of the long-term strategy.
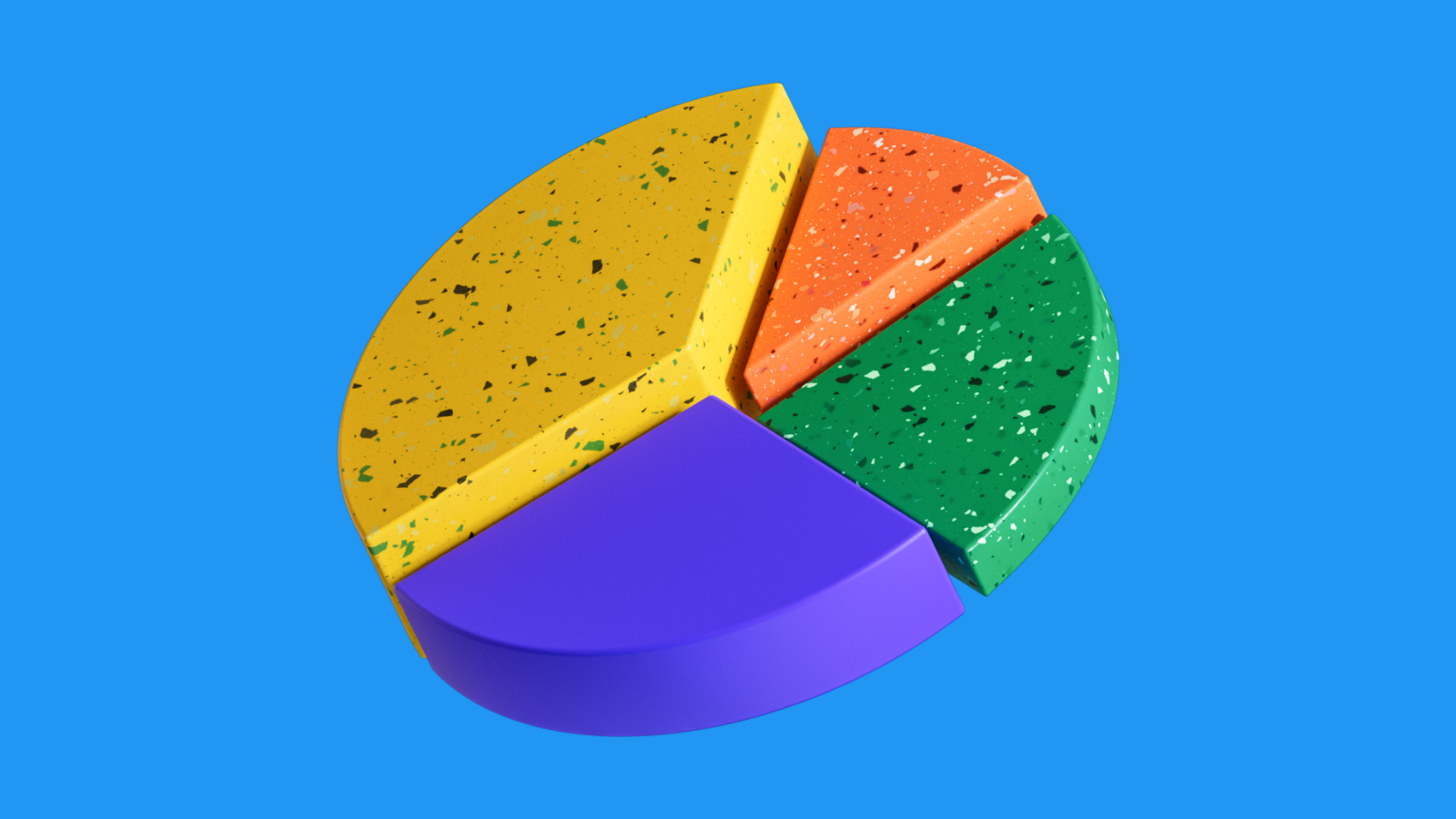
IFRX Stock Forecast Model: A Data-Driven Approach
Our team has developed a comprehensive machine learning model to forecast the performance of InflaRx N.V. Common Stock (IFRX). The model leverages a diverse dataset encompassing various factors known to influence stock prices, including historical trading data (price, volume, and volatility metrics), financial statements (revenue, earnings, cash flow), and market sentiment indicators (news articles, social media data). Additionally, we incorporate macroeconomic variables such as interest rates, inflation data, and overall market indices. The model utilizes a combination of advanced machine learning techniques, including time series analysis (specifically, LSTM networks) and ensemble methods (like Random Forests and Gradient Boosting) to capture both linear and non-linear relationships within the data. Feature engineering is critical; we construct leading indicators derived from fundamental and technical analysis to optimize prediction accuracy. The output of the model is a predicted direction of price movement for a defined time horizon.
The model's architecture is designed to adapt to the dynamic nature of the market. Regular model retraining and validation are performed using the latest data to ensure optimal predictive power. The model's performance is assessed using a range of evaluation metrics, including Mean Absolute Error (MAE), Root Mean Squared Error (RMSE), and directional accuracy. We implement rigorous cross-validation techniques to mitigate overfitting and maintain generalizability across various market conditions. The selection of model parameters and hyperparameter tuning are driven by these evaluation metrics and are conducted using a combination of grid search and Bayesian optimization. Furthermore, we consider economic principles, incorporating the relationship between industry-specific factors (e.g., clinical trial results, regulatory approvals) and IFRX's performance. The team will provide an extensive report on the performance of the model including limitations.
The final model presents a probabilistic forecast, reflecting the inherent uncertainty in financial markets. The output is a prediction of likely price movement trends that can be used to make trading decisions. The team's economists and data scientists will regularly refine and update the model with new data and emerging market trends. The user interface will be designed to be comprehensive and easy to use. Users will have the option to simulate various inputs. We offer ongoing support and documentation to facilitate understanding and interpretation of the model's outputs. The model will be subjected to rigorous stress tests to evaluate performance under extreme market scenarios and identify potential vulnerabilities.
ML Model Testing
n:Time series to forecast
p:Price signals of InflaRx N.V. stock
j:Nash equilibria (Neural Network)
k:Dominated move of InflaRx N.V. stock holders
a:Best response for InflaRx N.V. target price
For further technical information as per how our model work we invite you to visit the article below:
How do KappaSignal algorithms actually work?
InflaRx N.V. Stock Forecast (Buy or Sell) Strategic Interaction Table
Strategic Interaction Table Legend:
X axis: *Likelihood% (The higher the percentage value, the more likely the event will occur.)
Y axis: *Potential Impact% (The higher the percentage value, the more likely the price will deviate.)
Z axis (Grey to Black): *Technical Analysis%
InflaRx Financial Outlook and Forecast
InflaRx, a clinical-stage biopharmaceutical company, is developing innovative therapies targeting the complement system for the treatment of various inflammatory and autoimmune diseases. The company's financial outlook is primarily driven by the clinical progress of its lead drug candidate, vilobelimab, and its potential for commercialization. Positive data from pivotal Phase III trials, particularly for hidradenitis suppurativa (HS) and ANCA-associated vasculitis (AAV), will be crucial for securing regulatory approvals and driving future revenue. Successful commercialization will depend on market access, physician adoption, and competitive landscape. Strong partnerships and collaborations, alongside efficient operational strategies, will significantly impact its ability to reach its full potential. Furthermore, the company's cash position is a critical factor. InflaRx is reliant on raising funds through equity offerings, debt financing, or collaborations to fund ongoing clinical trials, research, and development activities until vilobelimab gains regulatory approval and achieves commercial revenue.
The market for InflaRx's target indications, particularly HS and AAV, represents significant unmet medical needs. The potential for vilobelimab to offer a novel mechanism of action by specifically inhibiting the complement component C5a, could give the drug a competitive advantage. The financial forecast hinges on successful execution of its clinical development plan and securing regulatory approvals in key markets like the United States and Europe. Successful clinical trials will increase InflaRx's attractiveness to potential partners, which can help in expanding clinical trials for other indications. Key drivers of revenue will be pricing strategies, the effectiveness of the drug in treating the targeted conditions, and the ability of the sales force to reach physicians. Also, the company's intellectual property rights, which protect vilobelimab, will play an important role in future profitability.
Regarding expenses, InflaRx's financial performance will be heavily influenced by research and development (R&D) costs, including the expenses for clinical trials, manufacturing of drug products, and other related activities. Operating expenses are expected to remain elevated in the short to medium term as the company advances clinical programs. Successful commercialization of vilobelimab will involve expenses related to building a sales and marketing infrastructure, manufacturing the drug, and distribution logistics. Also, general and administrative (G&A) expenses, including personnel, legal, and administrative costs, will also have a significant impact on the financials. Therefore, the management team must be very efficient to control those costs. InflaRx's valuation will be highly sensitive to data from clinical trials, particularly for vilobelimab, along with the company's ability to raise capital and establish strategic partnerships.
Based on current information, the financial outlook for InflaRx appears positive, assuming successful clinical outcomes and regulatory approvals for vilobelimab. Commercialization of vilobelimab could lead to significant revenue and market share growth. However, there are significant risks. Any failure in clinical trials or regulatory setbacks could lead to adverse results for its financials. Also, the company's reliance on external funding presents a risk of dilution and, if the company can't secure future funding, could put its development plans in jeopardy. The competitive landscape, particularly from well-established pharmaceutical companies developing therapies for similar indications, also poses a risk. Despite the potential rewards, investors need to be aware of the risks and consider them before making financial decisions.
Rating | Short-Term | Long-Term Senior |
---|---|---|
Outlook | Ba1 | Ba3 |
Income Statement | Ba3 | Caa2 |
Balance Sheet | Baa2 | B2 |
Leverage Ratios | Baa2 | B2 |
Cash Flow | B2 | Baa2 |
Rates of Return and Profitability | Baa2 | Baa2 |
*Financial analysis is the process of evaluating a company's financial performance and position by neural network. It involves reviewing the company's financial statements, including the balance sheet, income statement, and cash flow statement, as well as other financial reports and documents.
How does neural network examine financial reports and understand financial state of the company?
References
- Holland PW. 1986. Statistics and causal inference. J. Am. Stat. Assoc. 81:945–60
- Matzkin RL. 2007. Nonparametric identification. In Handbook of Econometrics, Vol. 6B, ed. J Heckman, E Learner, pp. 5307–68. Amsterdam: Elsevier
- Bell RM, Koren Y. 2007. Lessons from the Netflix prize challenge. ACM SIGKDD Explor. Newsl. 9:75–79
- E. van der Pol and F. A. Oliehoek. Coordinated deep reinforcement learners for traffic light control. NIPS Workshop on Learning, Inference and Control of Multi-Agent Systems, 2016.
- R. Rockafellar and S. Uryasev. Optimization of conditional value-at-risk. Journal of Risk, 2:21–42, 2000.
- Wager S, Athey S. 2017. Estimation and inference of heterogeneous treatment effects using random forests. J. Am. Stat. Assoc. 113:1228–42
- Pennington J, Socher R, Manning CD. 2014. GloVe: global vectors for word representation. In Proceedings of the 2014 Conference on Empirical Methods on Natural Language Processing, pp. 1532–43. New York: Assoc. Comput. Linguist.