AUC Score :
Short-term Tactic1 :
Dominant Strategy :
Time series to forecast n:
ML Model Testing : Modular Neural Network (DNN Layer)
Hypothesis Testing : Polynomial Regression
Surveillance : Major exchange and OTC
1Short-term revised.
2Time series is updated based on short-term trends.
Key Points
The Dow Jones U.S. Select Home Construction Index is expected to experience a period of moderate growth driven by persistent demand for housing, albeit at a slower pace compared to recent years, potentially offset by rising interest rates and building material costs which could impact affordability and profit margins, increasing the risk of a market correction. Furthermore, supply chain disruptions and labor shortages pose significant challenges. The index is likely to exhibit volatility, susceptible to shifts in macroeconomic conditions and consumer confidence levels. The overall market could face a correction if the Federal Reserve continues aggressive monetary policy tightening.About Dow Jones U.S. Select Home Construction Index
The Dow Jones U.S. Select Home Construction Index is a market capitalization-weighted index designed to represent the performance of the U.S. home construction industry. It tracks the financial performance of companies primarily involved in building residential homes. The index includes companies engaged in activities such as homebuilding, construction, and related products and services directly linked to the residential housing market. This focus allows investors to gauge the overall health and performance of the home construction sector, which is a significant component of the U.S. economy.
The index serves as a benchmark for investors seeking exposure to the U.S. housing market and provides a means of tracking the sector's trends and fluctuations. The components of the index are carefully selected and weighted based on specific criteria related to market capitalization and sector relevance. Regularly reviewed and rebalanced, the Dow Jones U.S. Select Home Construction Index reflects the evolving dynamics and composition of the home construction industry, making it a valuable tool for market analysis and investment decisions.
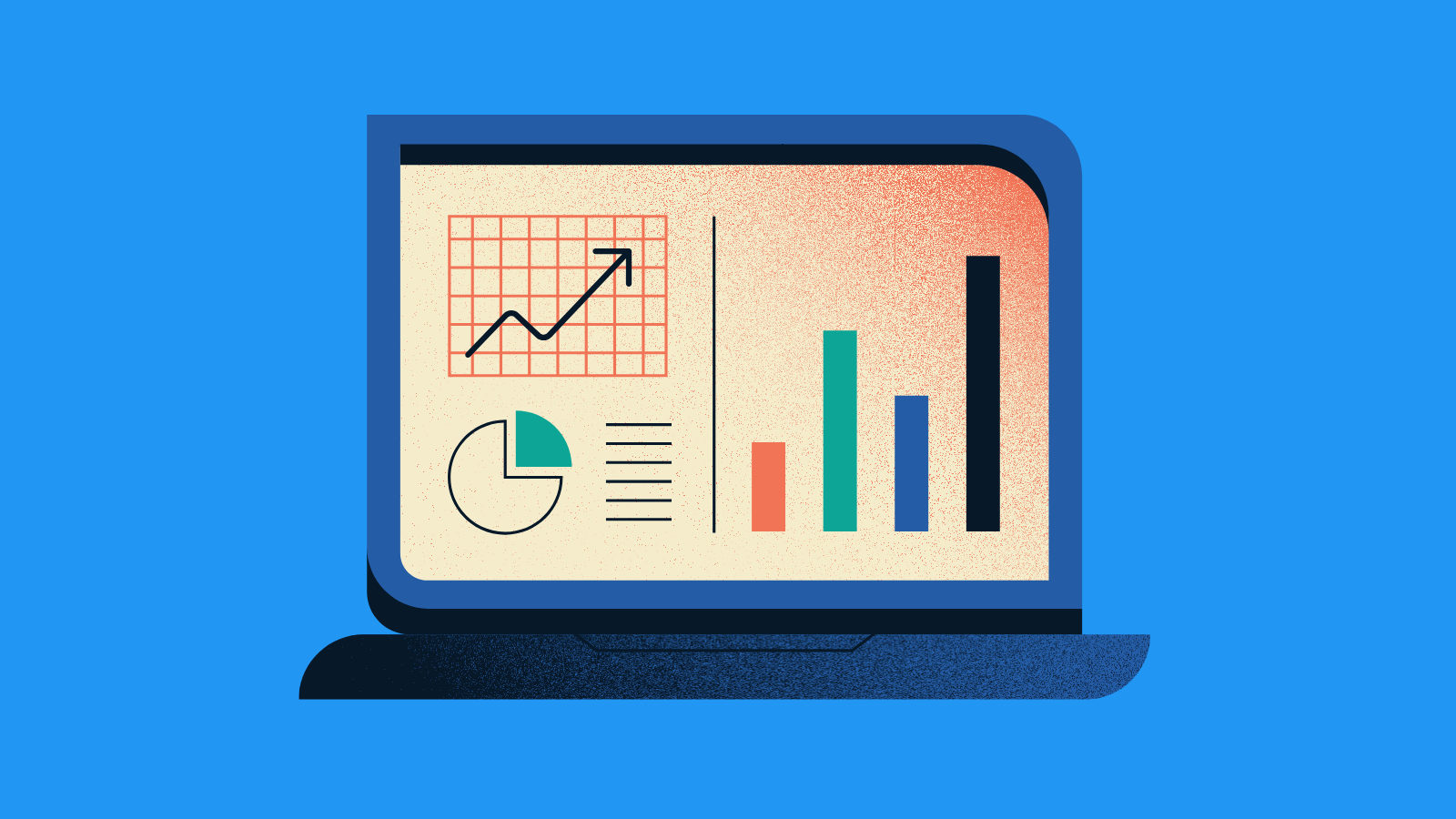
A Machine Learning Model for Forecasting the Dow Jones U.S. Select Home Construction Index
Our team proposes a machine learning model to forecast the Dow Jones U.S. Select Home Construction Index. The model will integrate a variety of economic and market-specific indicators to improve predictive accuracy. Key features will include housing starts, building permits, existing home sales, new home sales, consumer confidence indices, and mortgage rates. These are fundamental indicators reflecting the overall health of the housing market and consumer demand, influencing the index's performance. Furthermore, we will incorporate macroeconomic factors such as GDP growth, inflation rates (CPI), and employment data, providing a broader economic context. Finally, stock market performance of key companies within the home construction sector, represented by their individual stock prices and trading volumes, will be essential components for capturing industry-specific dynamics. The model's architecture will be designed to capture both linear and non-linear relationships.
We will explore and compare the performance of several machine learning algorithms, including Recurrent Neural Networks (RNNs), specifically Long Short-Term Memory (LSTM) networks, and Gradient Boosting Machines (GBMs), to identify the most effective forecasting approach. LSTM networks are well-suited for time series data, capable of capturing temporal dependencies and long-term trends inherent in financial markets. GBMs offer strong predictive power and handle complex interactions between various features effectively. Before modeling, comprehensive data pre-processing, including normalization and feature engineering, will be executed. The dataset will be divided into training, validation, and testing sets to ensure the model's robustness. The model will be evaluated using standard metrics, such as Mean Squared Error (MSE), Root Mean Squared Error (RMSE), and Mean Absolute Percentage Error (MAPE), to assess its predictive accuracy across different time horizons.
The final model will be deployed using a robust and scalable system, enabling real-time forecasting. The performance of the model will be continually monitored and its accuracy re-evaluated. Periodic retraining with fresh data will maintain the model's accuracy and account for changes in market conditions. Moreover, a sensitivity analysis will be carried out to identify the features which have a significant impact on the index forecast, providing valuable insight for investors and market analysts. The output of the model will be a forecast of the index, along with a measure of prediction uncertainty. This allows users to make informed investment decisions based on a comprehensive understanding of potential risks and rewards associated with the index. The ability to integrate new data and adapt to changing economic conditions is a key advantage of our machine learning approach.
ML Model Testing
n:Time series to forecast
p:Price signals of Dow Jones U.S. Select Home Construction index
j:Nash equilibria (Neural Network)
k:Dominated move of Dow Jones U.S. Select Home Construction index holders
a:Best response for Dow Jones U.S. Select Home Construction target price
For further technical information as per how our model work we invite you to visit the article below:
How do KappaSignal algorithms actually work?
Dow Jones U.S. Select Home Construction Index Forecast Strategic Interaction Table
Strategic Interaction Table Legend:
X axis: *Likelihood% (The higher the percentage value, the more likely the event will occur.)
Y axis: *Potential Impact% (The higher the percentage value, the more likely the price will deviate.)
Z axis (Grey to Black): *Technical Analysis%
Dow Jones U.S. Select Home Construction Index: Financial Outlook and Forecast
The Dow Jones U.S. Select Home Construction Index offers a comprehensive view of the financial health and future prospects of the U.S. homebuilding industry. Comprising publicly traded companies principally involved in the construction, design, and sale of residential homes, the index serves as a critical barometer for economic activity, consumer confidence, and the overall housing market. Several key factors influence this index, including interest rates, which directly affect mortgage affordability and demand; raw material costs, such as lumber and steel, which impact construction expenses and profit margins; and government policies, like tax incentives or regulatory changes that can stimulate or hinder development. Furthermore, demographic shifts, including population growth, household formation rates, and migration patterns, significantly shape the long-term outlook for the index. The index's performance is also sensitive to broader economic trends, such as inflation, unemployment rates, and consumer spending, which collectively influence the ability and willingness of individuals and families to invest in homeownership.
Current trends suggest a complex and dynamic landscape for the home construction sector. While the demand for housing remains relatively strong due to persistent demographic pressures and a historical undersupply in many markets, challenges persist. Rising interest rates, designed to combat inflation, are increasing mortgage rates, which can make homeownership less affordable and potentially cool down demand. Concurrently, high construction costs due to supply chain disruptions and labor shortages continue to squeeze builders' profitability. Furthermore, uncertainties surrounding the overall economic climate, including the possibility of a recession, could lead to a decline in consumer confidence and a slowdown in housing demand. However, certain positive factors, such as ongoing government initiatives aimed at boosting housing supply and a robust labor market, could partially offset these negative influences. Additionally, there is a growing trend toward sustainable and energy-efficient home construction, potentially leading to higher demand for companies that embrace these innovations.
The index's future trajectory will likely be determined by the interplay of these contrasting forces. The rate at which inflation is tamed and interest rates stabilize will be a crucial determinant. A sustained period of high inflation could lead to further rate hikes, dampening housing demand, and negatively impacting the index. Conversely, a successful effort to bring inflation under control could lead to a moderation in interest rates, thus providing support to the homebuilding sector. The continued impact of supply chain disruptions and the resolution of labor shortages are also critical factors. A return to more stable supply chains and a readily available workforce could lower construction costs and improve builders' profit margins. Moreover, the degree to which consumer confidence is maintained amidst broader economic uncertainty will influence the appetite for home purchases. The index's performance will also be dependent on policy changes, such as subsidies, tax credits, and zoning regulations. Ultimately, the strength of the housing market, and by extension, the index, will be a function of these factors interacting and shaping the overall economic environment.
Prediction: The Dow Jones U.S. Select Home Construction Index faces a period of moderate growth. While demographic trends and a persistent housing shortage should provide underlying support, the challenges of rising interest rates, increased construction costs, and overall economic uncertainty will likely limit the index's gains. Risks: The primary risks to this prediction include a more severe or prolonged economic recession, which could lead to a substantial decline in housing demand and a sharp downturn in the index. Unexpected spikes in raw material costs or prolonged labor shortages could also negatively affect the index's performance. Conversely, stronger-than-expected economic growth, a faster decline in inflation, or robust government support for homeownership could lead to stronger-than-anticipated gains. Furthermore, policy changes related to environmental regulations, such as increased requirements for energy-efficient construction, may provide a tailwind for companies that adopt green building practices.
Rating | Short-Term | Long-Term Senior |
---|---|---|
Outlook | B1 | B1 |
Income Statement | B3 | B1 |
Balance Sheet | Ba1 | Ba2 |
Leverage Ratios | Baa2 | Baa2 |
Cash Flow | B3 | B2 |
Rates of Return and Profitability | Caa2 | C |
*An aggregate rating for an index summarizes the overall sentiment towards the companies it includes. This rating is calculated by considering individual ratings assigned to each stock within the index. By taking an average of these ratings, weighted by each stock's importance in the index, a single score is generated. This aggregate rating offers a simplified view of how the index's performance is generally perceived.
How does neural network examine financial reports and understand financial state of the company?
References
- Jorgenson, D.W., Weitzman, M.L., ZXhang, Y.X., Haxo, Y.M. and Mat, Y.X., 2023. MRNA: The Next Big Thing in mRNA Vaccines. AC Investment Research Journal, 220(44).
- G. Theocharous and A. Hallak. Lifetime value marketing using reinforcement learning. RLDM 2013, page 19, 2013
- Belloni A, Chernozhukov V, Hansen C. 2014. High-dimensional methods and inference on structural and treatment effects. J. Econ. Perspect. 28:29–50
- White H. 1992. Artificial Neural Networks: Approximation and Learning Theory. Oxford, UK: Blackwell
- K. Tuyls and G. Weiss. Multiagent learning: Basics, challenges, and prospects. AI Magazine, 33(3): 41–52, 2012
- Belsley, D. A. (1988), "Modelling and forecast reliability," International Journal of Forecasting, 4, 427–447.
- Blei DM, Lafferty JD. 2009. Topic models. In Text Mining: Classification, Clustering, and Applications, ed. A Srivastava, M Sahami, pp. 101–24. Boca Raton, FL: CRC Press