AUC Score :
Short-term Tactic1 :
Dominant Strategy :
Time series to forecast n:
ML Model Testing : Modular Neural Network (Speculative Sentiment Analysis)
Hypothesis Testing : Statistical Hypothesis Testing
Surveillance : Major exchange and OTC
1Short-term revised.
2Time series is updated based on short-term trends.
Key Points
Hamilton Insurance Group's Class B shares are anticipated to experience moderate growth, driven by expansion in its specialty insurance lines and strategic acquisitions. This positive outlook hinges on maintaining underwriting discipline and effectively integrating any acquired entities. Risks include potential volatility in the reinsurance market, increased competition, and unforeseen losses from catastrophic events, which could significantly impact profitability. Further risks involve changes in interest rates that may affect investment income, as well as the capacity to successfully navigate regulatory changes.About Hamilton Insurance Group Ltd.
Hamilton Insurance Group Ltd. (HIGL) is a Bermuda-based holding company operating primarily in the property and casualty insurance and reinsurance sector. It focuses on underwriting specialty insurance and reinsurance risks globally. The company utilizes data analytics and technology to inform its underwriting decisions and improve operational efficiency. HIGL operates through a variety of subsidiaries and platforms, serving a diverse range of clients and brokers.
The company's strategy emphasizes disciplined underwriting, strategic partnerships, and innovation. It aims to achieve profitable growth and long-term value creation for its stakeholders. HIGL is committed to providing comprehensive insurance and reinsurance solutions, addressing the evolving needs of its clients in an increasingly complex risk landscape. The company's success depends on its ability to effectively manage risk, leverage data insights, and maintain strong relationships within the insurance industry.
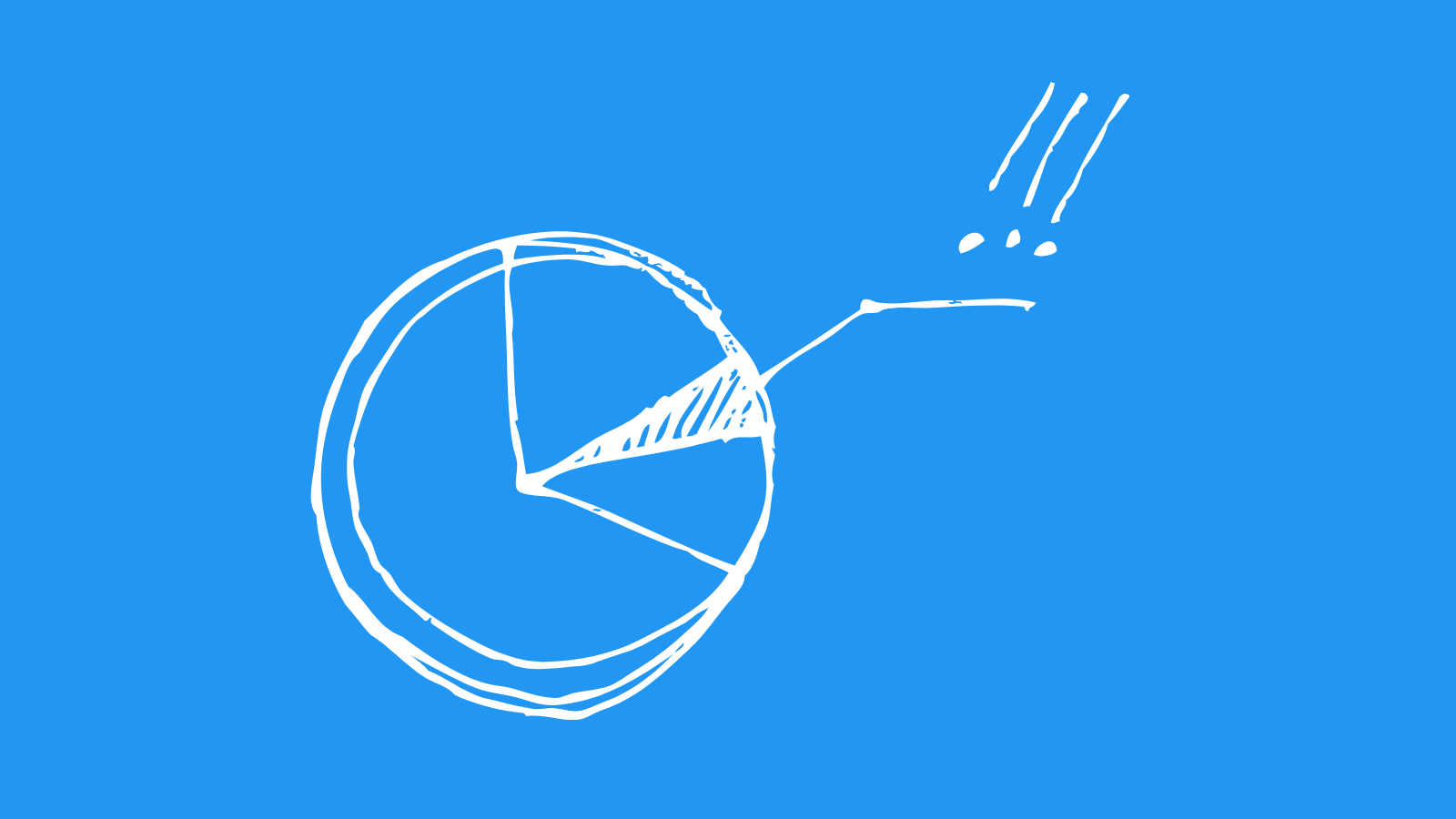
HG Stock Forecasting Model
Our team, comprising data scientists and economists, proposes a comprehensive machine learning model for forecasting the performance of Hamilton Insurance Group Ltd. Class B Common Shares (HG). The model will leverage a diverse range of input features categorized into macroeconomic indicators, financial statement analysis, and market sentiment data. Macroeconomic data will include GDP growth, inflation rates, interest rates, and unemployment figures, reflecting the overall economic environment's impact on the insurance industry. Financial statement data will encompass key metrics from HG's filings, such as revenue, net income, combined ratio, loss ratios, and expense ratios, providing insights into the company's financial health and operational efficiency. Market sentiment will be gauged using news sentiment analysis, social media trends, and analyst ratings to capture investor perceptions and market expectations.
The model will employ a hybrid approach, combining the strengths of multiple machine learning algorithms. Initially, time series models like ARIMA (Autoregressive Integrated Moving Average) and Exponential Smoothing will be utilized to capture the temporal dependencies and trends inherent in HG's historical stock performance. These models will establish a baseline forecast. Subsequently, we will implement more advanced algorithms such as Random Forests, Gradient Boosting Machines, and possibly a Recurrent Neural Network (RNN) variant like LSTM (Long Short-Term Memory) to capture non-linear relationships and complex interactions between the input features and stock returns. These algorithms are well-suited to handle the volume and complexity of the data.
Model performance will be rigorously evaluated using appropriate metrics such as Mean Absolute Error (MAE), Root Mean Squared Error (RMSE), and Sharpe Ratio, and will be assessed through backtesting to measure its predictive power and reliability. Regular model retraining will be conducted with the latest data to ensure the model stays current with evolving market dynamics and company-specific performance. Model interpretability will also be a focus, using feature importance techniques to provide insights into the key drivers of HG's stock performance, which helps in effective risk management and strategic decision-making. Our model provides a robust and adaptable framework for forecasting HG's stock performance.
ML Model Testing
n:Time series to forecast
p:Price signals of Hamilton Insurance Group Ltd. stock
j:Nash equilibria (Neural Network)
k:Dominated move of Hamilton Insurance Group Ltd. stock holders
a:Best response for Hamilton Insurance Group Ltd. target price
For further technical information as per how our model work we invite you to visit the article below:
How do KappaSignal algorithms actually work?
Hamilton Insurance Group Ltd. Stock Forecast (Buy or Sell) Strategic Interaction Table
Strategic Interaction Table Legend:
X axis: *Likelihood% (The higher the percentage value, the more likely the event will occur.)
Y axis: *Potential Impact% (The higher the percentage value, the more likely the price will deviate.)
Z axis (Grey to Black): *Technical Analysis%
Hamilton Insurance Group Ltd. Class B Common Shares: Financial Outlook and Forecast
The financial outlook for Hamilton is influenced by a confluence of factors inherent to the insurance and reinsurance industries, as well as the company's specific strategies and performance. The company's core business, encompassing insurance and reinsurance operations, is significantly affected by global economic conditions, natural catastrophe events, and evolving regulatory landscapes. Recent periods have seen elevated levels of inflation, influencing loss costs and investment returns, which in turn directly impacts Hamilton's profitability. Furthermore, the company's growth is tied to its ability to underwrite risks effectively and its success in developing and scaling its technology-driven underwriting platform. Another important thing is the company's expansion into new markets and the development of innovative insurance products, creating new revenue streams but also introducing complexities of execution and market acceptance. The firm's ability to capitalize on market opportunities and adapt to changes is also an important thing.
Hamilton's financial forecast can be understood by considering its existing operational strengths and strategic initiatives. Its technology-driven underwriting platform, which enhances efficiency and claims process management, is one important element. Investments in this technology are expected to continue, potentially leading to improved profitability and efficiency ratios compared to traditional insurance models. The company's ability to manage its investment portfolio, adapting it to higher interest rates, is an important point. Strategic partnerships and acquisitions could fuel further expansion, providing broader access to markets and greater diversification of risk. However, the forecast is subject to some important factors. The frequency and severity of natural disasters, the intensity of competition in the insurance sector, and the company's capacity to retain and attract talent are all pivotal.
Key metrics to watch include Hamilton's underwriting profitability, demonstrated by its combined ratio (claims and expenses relative to premiums earned), and its investment income performance. Growth in gross premiums written and net premiums earned would indicate successful market penetration and underwriting strategy execution. The performance of the company's technology investments, gauged by improved efficiency and customer satisfaction scores, also will be very important. The ability of Hamilton to consistently outperform industry averages in its operating ratios and to maintain a strong capital position are essential indicators of its financial health and ability to weather unforeseen events. Additionally, tracking the company's efforts to diversify its product offerings and geographic reach will provide insights into its long-term growth prospects.
Given the aforementioned conditions and projected developments, a moderately positive outlook is predicted for Hamilton. The company's commitment to leveraging technology and its strategic initiatives position it favorably to navigate the complexities of the insurance market. However, the outlook is not without risks. Natural catastrophes, macroeconomic volatility, and the competitive pressures in the insurance sector could severely impact performance. Moreover, the integration of acquisitions and the execution of its growth plans carry implementation risks. The company's ability to manage these risks, maintain a disciplined underwriting approach, and effectively deploy its capital will be crucial in determining its long-term success. Therefore, while the foundations for sustained growth are present, investors should remain vigilant and closely monitor the company's performance against these potential challenges.
```Rating | Short-Term | Long-Term Senior |
---|---|---|
Outlook | B3 | B1 |
Income Statement | Caa2 | Baa2 |
Balance Sheet | Baa2 | Baa2 |
Leverage Ratios | Baa2 | B2 |
Cash Flow | C | C |
Rates of Return and Profitability | C | C |
*Financial analysis is the process of evaluating a company's financial performance and position by neural network. It involves reviewing the company's financial statements, including the balance sheet, income statement, and cash flow statement, as well as other financial reports and documents.
How does neural network examine financial reports and understand financial state of the company?
References
- Canova, F. B. E. Hansen (1995), "Are seasonal patterns constant over time? A test for seasonal stability," Journal of Business and Economic Statistics, 13, 237–252.
- Abadie A, Imbens GW. 2011. Bias-corrected matching estimators for average treatment effects. J. Bus. Econ. Stat. 29:1–11
- Chernozhukov V, Chetverikov D, Demirer M, Duflo E, Hansen C, et al. 2016a. Double machine learning for treatment and causal parameters. Tech. Rep., Cent. Microdata Methods Pract., Inst. Fiscal Stud., London
- Chernozhukov V, Newey W, Robins J. 2018c. Double/de-biased machine learning using regularized Riesz representers. arXiv:1802.08667 [stat.ML]
- Imbens GW, Lemieux T. 2008. Regression discontinuity designs: a guide to practice. J. Econom. 142:615–35
- D. Bertsekas. Dynamic programming and optimal control. Athena Scientific, 1995.
- Chen X. 2007. Large sample sieve estimation of semi-nonparametric models. In Handbook of Econometrics, Vol. 6B, ed. JJ Heckman, EE Learner, pp. 5549–632. Amsterdam: Elsevier