AUC Score :
Short-term Tactic1 :
Dominant Strategy :
Time series to forecast n:
ML Model Testing : Modular Neural Network (Market News Sentiment Analysis)
Hypothesis Testing : Chi-Square
Surveillance : Major exchange and OTC
1Short-term revised.
2Time series is updated based on short-term trends.
Key Points
GXO's future performance is projected to experience continued expansion in the e-commerce and supply chain sectors, fueled by its robust infrastructure and global presence, leading to organic revenue growth. Potential risks include increased competition from established players and emerging logistics firms that could compress margins. Economic downturns or shifts in consumer spending patterns might negatively affect volume and demand for logistics services. Furthermore, disruptions to global supply chains, possibly stemming from geopolitical instability or unforeseen events, could impact GXO's operational efficiency and profitability. Integration challenges from ongoing acquisitions, along with labor market fluctuations, including wage pressures and scarcity of skilled workers, pose further potential headwinds for GXO's sustained success.About GXO Logistics
GXO Logistics, Inc. is a prominent player in the global contract logistics market. Spun off from XPO Logistics in 2021, the company specializes in providing a wide array of supply chain solutions. These encompass warehousing, distribution, e-commerce fulfillment, and reverse logistics services for diverse industries. GXO leverages advanced technology, including automation and robotics, to optimize its operations and enhance efficiency for its clients. It operates a vast network of facilities and offers services across multiple regions, including North America and Europe.
GXO's strategy emphasizes growth through expanding its customer base, developing innovative solutions, and pursuing strategic acquisitions. The company focuses on long-term contracts and building enduring partnerships with its clients. Furthermore, it is committed to sustainability and reducing the environmental impact of its operations. GXO strives to adapt to evolving market demands, particularly those driven by the ongoing growth of e-commerce and the need for efficient supply chain management.
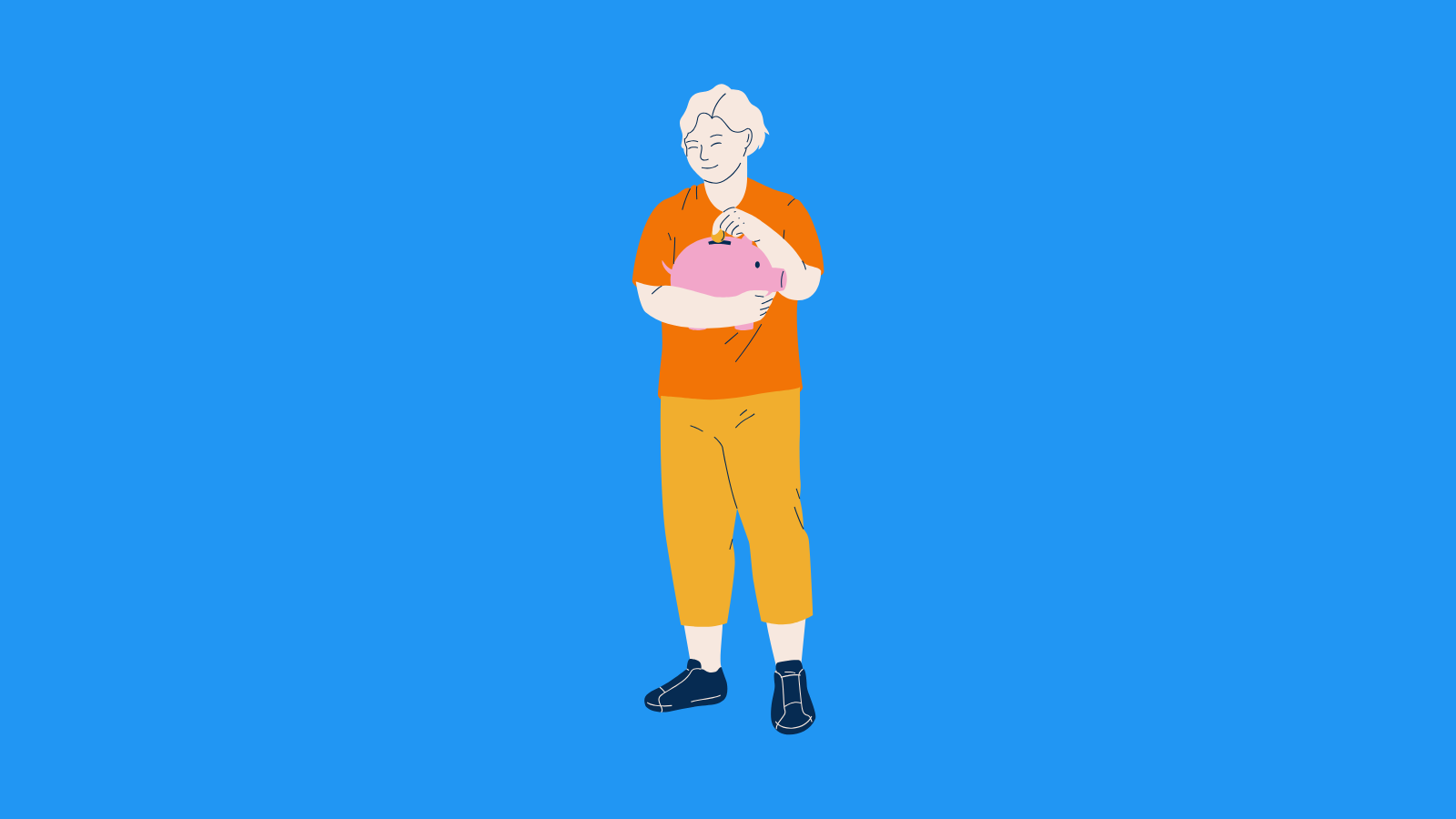
GXO Stock Forecast Model
Our team of data scientists and economists has developed a machine learning model to forecast the performance of GXO Logistics Inc. (GXO) common stock. The model leverages a comprehensive set of features categorized into three primary groups: market indicators, company-specific fundamentals, and sentiment analysis. Market indicators include macroeconomic variables such as GDP growth, inflation rates, and interest rate trends, as these factors significantly impact overall market sentiment and investor behavior. Company-specific fundamentals encompass financial ratios like revenue growth, profit margins, debt levels, and cash flow, derived from GXO's quarterly and annual reports. Sentiment analysis incorporates the processing of news articles, social media data, and analyst reports to gauge public perception and investor expectations regarding GXO. The model employs a time-series approach and utilizes a combination of algorithms, including Recurrent Neural Networks (RNNs), specifically Long Short-Term Memory (LSTM) networks, known for their ability to capture long-term dependencies in sequential data, and gradient boosting algorithms such as XGBoost and LightGBM to improve prediction accuracy.
The model's architecture includes several key steps. First, we perform rigorous data cleaning and preprocessing to handle missing values, outliers, and ensure data consistency across all feature sets. Feature engineering is then implemented to create new variables or transform existing ones to enhance predictive power, such as calculating moving averages or deriving ratios. The time-series data is split into training, validation, and test sets, with cross-validation techniques to mitigate overfitting. The training set is used to train and optimize the model parameters using a variety of hyperparameter tuning methods. Performance is assessed using relevant metrics such as Mean Absolute Error (MAE), Root Mean Squared Error (RMSE), and R-squared. The validation set is used to further refine parameters and evaluate the model's generalization ability. Finally, we test the model's performance on the unseen test set, representing a forward-looking prediction.
Our model's output is a forecast of the future direction of GXO's stock, incorporating the predicted likelihood of upward, downward, or stable trends. The model's outputs will be accompanied by confidence intervals to provide a measure of the uncertainty associated with the predictions. Further, we plan to continuously refine the model by incorporating the latest economic data, GXO's performance results, and updated sentiment analysis. Model performance is carefully monitored, and frequent retraining is scheduled to maintain prediction accuracy over time. We also anticipate using ensemble methods to combine the predictions of different models for further accuracy improvements. This model is designed to offer guidance, and it must be understood that no model can guarantee future market outcomes.
ML Model Testing
n:Time series to forecast
p:Price signals of GXO Logistics stock
j:Nash equilibria (Neural Network)
k:Dominated move of GXO Logistics stock holders
a:Best response for GXO Logistics target price
For further technical information as per how our model work we invite you to visit the article below:
How do KappaSignal algorithms actually work?
GXO Logistics Stock Forecast (Buy or Sell) Strategic Interaction Table
Strategic Interaction Table Legend:
X axis: *Likelihood% (The higher the percentage value, the more likely the event will occur.)
Y axis: *Potential Impact% (The higher the percentage value, the more likely the price will deviate.)
Z axis (Grey to Black): *Technical Analysis%
GXO Logistics Inc. Common Stock Financial Outlook and Forecast
GXO's financial outlook appears promising, driven by its strategic focus on technology-driven logistics solutions and a strong position within the rapidly expanding e-commerce and omnichannel fulfillment markets. The company has demonstrated robust revenue growth, driven by both organic expansion and strategic acquisitions. These acquisitions have allowed GXO to broaden its service offerings and enter new geographical markets. The company is expected to continue to benefit from increased outsourcing of logistics functions by businesses seeking to optimize their supply chains and reduce costs. Furthermore, GXO's investment in automation and other advanced technologies is anticipated to enhance operational efficiency, improve margins, and support its capacity to manage increasingly complex logistics needs. GXO's focus on specialized services, such as reverse logistics and cold chain management, positions it well to capture growth in niche markets.
Forecasts anticipate continued revenue growth for GXO in the coming years. Increased e-commerce penetration and growing demand for sophisticated supply chain solutions are expected to be key drivers. The company's investments in warehouse automation and robotics should contribute to margin expansion and increased profitability. Industry analysts project that GXO can sustain a rate of revenue growth that is higher than the overall industry average. GXO's leadership team has demonstrated effective operational execution and a disciplined approach to capital allocation, factors that are expected to support its financial performance. The company is also likely to benefit from global supply chain disruptions as customers seek to diversify their logistics providers and improve resilience. Continued focus on cost management and operational efficiency is expected to further improve the company's profitability.
GXO's financial performance is highly dependent on macroeconomic conditions. Factors such as fluctuations in economic growth, inflation, and consumer spending can impact demand for logistics services. The company's exposure to specific geographic regions, particularly North America and Europe, means that economic challenges in those markets can pose risks. The ability to successfully integrate acquisitions and realize projected synergies is crucial for sustained growth and profitability. Competition from other large logistics providers and smaller, niche players presents a constant challenge. Furthermore, GXO is subject to risks related to labor costs, fuel prices, and supply chain disruptions. Potential regulatory changes, particularly those impacting transportation and warehousing, could also affect its operations and profitability. Cybersecurity threats pose a risk, given the company's reliance on information technology systems.
Overall, the outlook for GXO is positive. The company is well-positioned to capitalize on the growth of the logistics market. However, the projected growth is subject to various risks, including macroeconomic volatility, intense competition, and potential supply chain disruptions. The prediction is that GXO will experience continued revenue and profit growth, though maintaining this momentum will require successful execution of strategic initiatives, effective management of costs, and the ability to mitigate potential risks. The biggest risks to this positive outlook are an economic downturn in key markets, failure to efficiently integrate future acquisitions, and inability to adapt to technological changes. The company's performance hinges on managing these risks effectively while capitalizing on the opportunities presented by a dynamic and evolving logistics landscape.
Rating | Short-Term | Long-Term Senior |
---|---|---|
Outlook | Ba3 | Ba1 |
Income Statement | Ba1 | B2 |
Balance Sheet | B3 | Baa2 |
Leverage Ratios | Baa2 | Baa2 |
Cash Flow | Baa2 | Baa2 |
Rates of Return and Profitability | C | B1 |
*Financial analysis is the process of evaluating a company's financial performance and position by neural network. It involves reviewing the company's financial statements, including the balance sheet, income statement, and cash flow statement, as well as other financial reports and documents.
How does neural network examine financial reports and understand financial state of the company?
References
- Chen X. 2007. Large sample sieve estimation of semi-nonparametric models. In Handbook of Econometrics, Vol. 6B, ed. JJ Heckman, EE Learner, pp. 5549–632. Amsterdam: Elsevier
- V. Mnih, A. P. Badia, M. Mirza, A. Graves, T. P. Lillicrap, T. Harley, D. Silver, and K. Kavukcuoglu. Asynchronous methods for deep reinforcement learning. In Proceedings of the 33nd International Conference on Machine Learning, ICML 2016, New York City, NY, USA, June 19-24, 2016, pages 1928–1937, 2016
- Challen, D. W. A. J. Hagger (1983), Macroeconomic Systems: Construction, Validation and Applications. New York: St. Martin's Press.
- Jorgenson, D.W., Weitzman, M.L., ZXhang, Y.X., Haxo, Y.M. and Mat, Y.X., 2023. Can Neural Networks Predict Stock Market?. AC Investment Research Journal, 220(44).
- Bottou L. 1998. Online learning and stochastic approximations. In On-Line Learning in Neural Networks, ed. D Saad, pp. 9–42. New York: ACM
- E. Altman, K. Avrachenkov, and R. N ́u ̃nez-Queija. Perturbation analysis for denumerable Markov chains with application to queueing models. Advances in Applied Probability, pages 839–853, 2004
- M. J. Hausknecht. Cooperation and Communication in Multiagent Deep Reinforcement Learning. PhD thesis, The University of Texas at Austin, 2016