AUC Score :
Short-term Tactic1 :
Dominant Strategy :
Time series to forecast n:
ML Model Testing : Multi-Instance Learning (ML)
Hypothesis Testing : Lasso Regression
Surveillance : Major exchange and OTC
1Short-term revised.
2Time series is updated based on short-term trends.
Key Points
Genasys' future hinges on its ability to secure and effectively execute significant government contracts and expand its commercial customer base, particularly within the emergency communication and public safety sectors. Positive predictions include strong revenue growth if large-scale deployments of its systems occur, driven by increasing global demand for advanced communication technologies. Conversely, Genasys faces risks centered around intense competition from established players, the potential for delays or cancellations in government contracts, and the need for continued investment in research and development to maintain a competitive edge. Failure to innovate or meet evolving technological requirements could significantly diminish its market position. Economic downturns impacting government spending and commercial budgets could also hinder growth. The company's success is therefore dependent on its operational execution, strategic partnerships, and capacity to adapt to a rapidly changing technological landscape.About Genasys
Genasys Inc. (GNSS) is a provider of critical communications systems and solutions. The company specializes in developing and delivering advanced technologies for mass notification, emergency management, and public safety applications. These solutions are designed to enable organizations and individuals to rapidly disseminate crucial information during emergencies, natural disasters, and other critical events. The company's products often include sophisticated software platforms, hardware components, and communication networks.
GNSS primarily serves governmental agencies, public safety organizations, and commercial enterprises. Its focus lies in enhancing the safety and security of communities and infrastructure. The company offers a comprehensive suite of products and services tailored to address diverse communication needs across a variety of industries. Genasys strives to provide reliable and effective communication tools for various situations, from routine alerts to large-scale disaster response efforts.
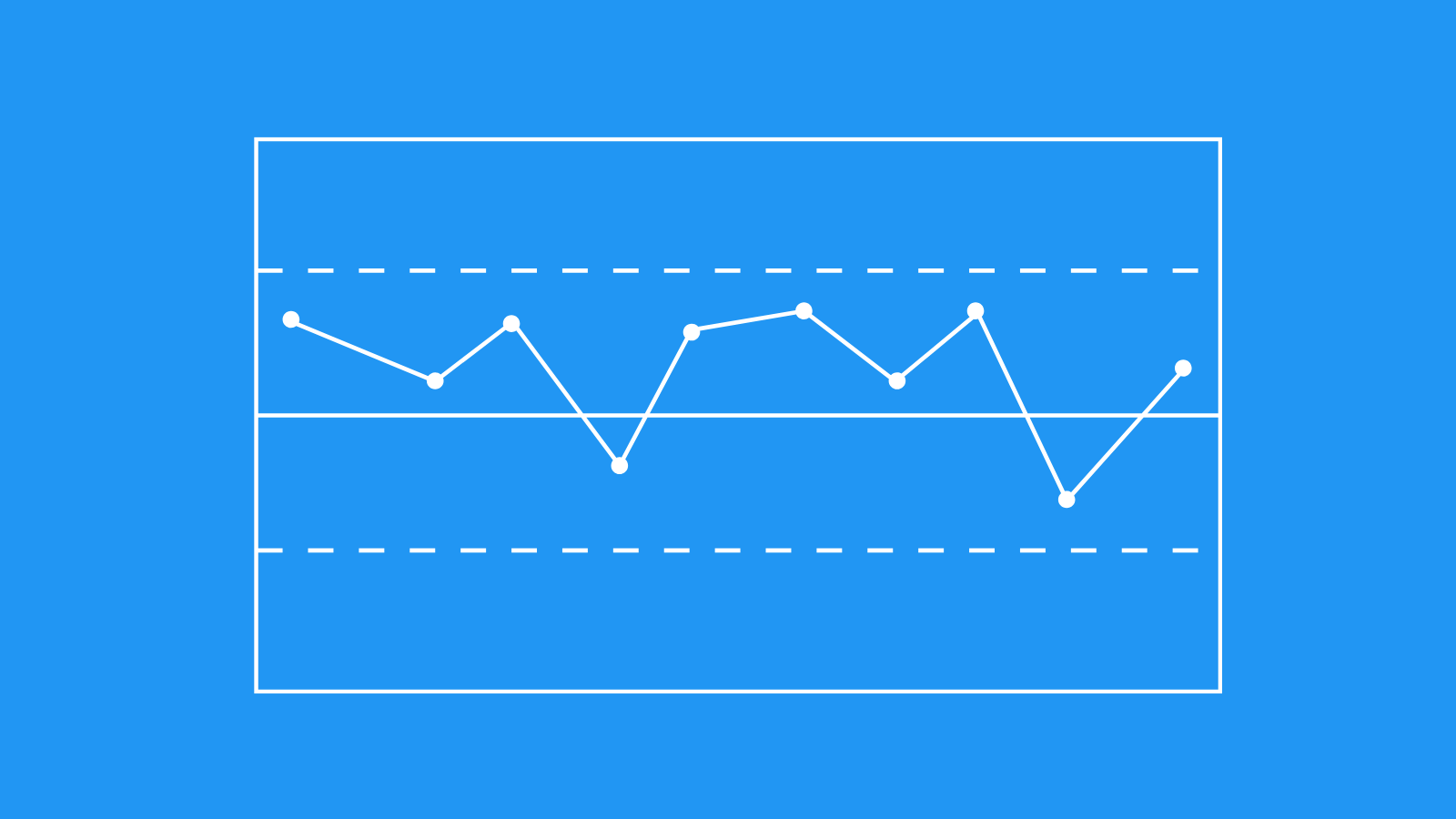
GNSS Stock Prediction Model
Our team proposes a comprehensive machine learning model for forecasting Genasys Inc. (GNSS) common stock performance. The model leverages a combination of techniques to capture both fundamental and technical indicators, aiming to provide accurate predictions. First, we will construct a feature set encompassing financial ratios such as price-to-earnings, debt-to-equity, and revenue growth, derived from the company's quarterly and annual reports. We will supplement this with macroeconomic data including inflation rates, interest rates, and sector-specific performance metrics. Finally, we incorporate technical indicators derived from historical price and volume data, including moving averages, Relative Strength Index (RSI), and trading volume anomalies. This rich dataset will be used to train and validate our model.
The core of our model will be a hybrid architecture combining a Recurrent Neural Network (RNN), specifically a Long Short-Term Memory (LSTM) network, with a Gradient Boosting Machine (GBM). The LSTM will be particularly effective at capturing temporal dependencies and patterns within the time series data of financial metrics and macroeconomic indicators. Simultaneously, the GBM will be used for incorporating categorical data and handling non-linear relationships within the financial ratios and technical indicators. Feature selection and dimensionality reduction will be performed using techniques such as principal component analysis (PCA) to minimize the complexity of the model and improve its generalization capabilities, mitigating overfitting. Regularization techniques will be incorporated to prevent overfitting, such as L1 and L2 regularization on the GBM and dropout on the LSTM layers.
The model's performance will be rigorously evaluated using a time-series cross-validation approach to simulate real-world forecasting scenarios. Key metrics will include Mean Absolute Error (MAE), Root Mean Squared Error (RMSE), and directional accuracy (the percentage of correctly predicted price direction changes). We will conduct sensitivity analysis to assess the impact of each feature on the final predictions, facilitating better model interpretability and risk management. Furthermore, we will regularly retrain the model with updated data to ensure its continued accuracy and adaptability to changing market conditions. The model will provide probabilistic output, offering confidence intervals around the forecasts to reflect the inherent uncertainty in financial markets. Our goal is to provide Genasys with a robust and reliable tool for informed investment decisions.
ML Model Testing
n:Time series to forecast
p:Price signals of Genasys stock
j:Nash equilibria (Neural Network)
k:Dominated move of Genasys stock holders
a:Best response for Genasys target price
For further technical information as per how our model work we invite you to visit the article below:
How do KappaSignal algorithms actually work?
Genasys Stock Forecast (Buy or Sell) Strategic Interaction Table
Strategic Interaction Table Legend:
X axis: *Likelihood% (The higher the percentage value, the more likely the event will occur.)
Y axis: *Potential Impact% (The higher the percentage value, the more likely the price will deviate.)
Z axis (Grey to Black): *Technical Analysis%
Genasys Inc. (GNSS) Financial Outlook and Forecast
GNSS, a company specializing in critical communications systems, currently demonstrates a mixed financial outlook. The company's recent performance reflects a combination of growth in certain areas alongside challenges within others. Revenues have experienced fluctuations, influenced by the timing and size of government contracts, which constitute a significant portion of GNSS's business. Increased demand for emergency alert systems driven by global instability and natural disasters provides a tailwind for the company, offering opportunities for sustained revenue growth. The company's expansion into new markets and product diversification initiatives also demonstrate the company's proactive strategy. Conversely, macroeconomic headwinds, including supply chain disruptions and inflationary pressures, have impacted operational costs and profitability. Despite these challenges, GNSS continues to invest in research and development, particularly in software-based solutions, which should lead to more recurring revenue streams and improved gross margins in the long term.
GNSS's profitability metrics warrant close attention. While the company has shown a strong revenue base, profit margins have varied over time. This variability is tied to project mix, the aforementioned supply chain issues, and investment spending. Cost management initiatives, including streamlining operations and optimizing resource allocation, are critical factors that should drive the company towards improved profitability. Management's focus on operational efficiency appears well-placed, and effective execution in these areas could lead to margin expansion. Furthermore, stronger customer relationships, especially within the government and public safety sectors, can translate into higher contract renewal rates and a more predictable revenue stream. This stability would, in turn, support improved financial forecasting and greater investor confidence. The company's liquidity position is satisfactory, providing resources for future investments and strategic acquisitions, if deemed necessary.
Looking ahead, a favorable scenario for GNSS involves accelerating revenue growth, driven by the increasing importance of its core communications technologies. Government spending on emergency preparedness and disaster response is likely to remain robust, providing a consistent demand driver. Success in attracting and retaining key government and commercial contracts will be crucial. Further, successful execution of product development and diversification efforts, including expansion into the cloud-based services, will enhance revenue stability and profitability. The company's ability to effectively manage its cost structure and improve margins will be a key differentiator. Strategic partnerships and acquisitions, if properly integrated, could offer accelerated growth, potentially leading to increased shareholder value.
Predicting the future for GNSS, the current trajectory suggests a moderately positive outlook. Sustained growth in revenue, driven by external demand and successful execution, is anticipated, although the pace may be uneven. The primary risk to this outlook is the company's reliance on government contracts, which are subject to political and budgetary shifts. Delays in contract awards or changes in government spending priorities could negatively impact revenues. Further risks include heightened competition in the communications sector, and potential technological obsolescence. GNSS must continually innovate and maintain a competitive edge to thrive in the evolving market landscape. In addition, any significant disruption in global supply chains could again impact operating costs and project timelines, thereby affecting financial results.
Rating | Short-Term | Long-Term Senior |
---|---|---|
Outlook | B1 | Ba1 |
Income Statement | Caa2 | Caa2 |
Balance Sheet | Ba2 | Baa2 |
Leverage Ratios | Baa2 | Baa2 |
Cash Flow | B2 | Baa2 |
Rates of Return and Profitability | B2 | B2 |
*Financial analysis is the process of evaluating a company's financial performance and position by neural network. It involves reviewing the company's financial statements, including the balance sheet, income statement, and cash flow statement, as well as other financial reports and documents.
How does neural network examine financial reports and understand financial state of the company?
References
- A. Tamar, D. Di Castro, and S. Mannor. Policy gradients with variance related risk criteria. In Proceedings of the Twenty-Ninth International Conference on Machine Learning, pages 387–396, 2012.
- Athey S, Bayati M, Doudchenko N, Imbens G, Khosravi K. 2017a. Matrix completion methods for causal panel data models. arXiv:1710.10251 [math.ST]
- Zubizarreta JR. 2015. Stable weights that balance covariates for estimation with incomplete outcome data. J. Am. Stat. Assoc. 110:910–22
- S. J. Russell and P. Norvig. Artificial Intelligence: A Modern Approach. Prentice Hall, Englewood Cliffs, NJ, 3nd edition, 2010
- Bengio Y, Ducharme R, Vincent P, Janvin C. 2003. A neural probabilistic language model. J. Mach. Learn. Res. 3:1137–55
- Jorgenson, D.W., Weitzman, M.L., ZXhang, Y.X., Haxo, Y.M. and Mat, Y.X., 2023. Can Neural Networks Predict Stock Market?. AC Investment Research Journal, 220(44).
- Bessler, D. A. T. Covey (1991), "Cointegration: Some results on U.S. cattle prices," Journal of Futures Markets, 11, 461–474.