AUC Score :
Short-term Tactic1 :
Dominant Strategy :
Time series to forecast n:
ML Model Testing : Transfer Learning (ML)
Hypothesis Testing : Multiple Regression
Surveillance : Major exchange and OTC
1Short-term revised.
2Time series is updated based on short-term trends.
Key Points
FR has a mixed outlook. Revenue growth is anticipated due to expansion projects and increased gaming activity, particularly in emerging markets. Profitability should improve, driven by operational efficiencies and potentially higher margins in established casinos. However, risks persist, including economic downturns that could reduce consumer spending on discretionary activities like gaming and leisure. Increased competition from new casino openings and online gaming platforms presents additional challenges. Any regulatory changes, especially those related to gaming taxes or licensing, could significantly impact financial performance. The company is also exposed to potential disruptions like natural disasters or labor disputes, affecting its operational stability.About Full House Resorts
Full House Resorts is a publicly traded company focused on the development, ownership, and operation of casinos and related hospitality facilities. The company's strategy emphasizes establishing properties in markets with limited existing competition, targeting locations that offer potential for significant growth. FLL has a diversified portfolio, including casinos in various states, often emphasizing unique themes or attractions to appeal to local and regional markets.
FLL's operations typically involve offering a range of gaming options, including slot machines, table games, and sports betting, alongside dining and entertainment venues. Management prioritizes maintaining strong relationships with local communities and regulatory bodies. Expansion often involves strategic acquisitions and development projects, aimed at capitalizing on emerging market opportunities and enhancing the company's overall value proposition. The company's focus is on offering differentiated gaming and entertainment experiences.
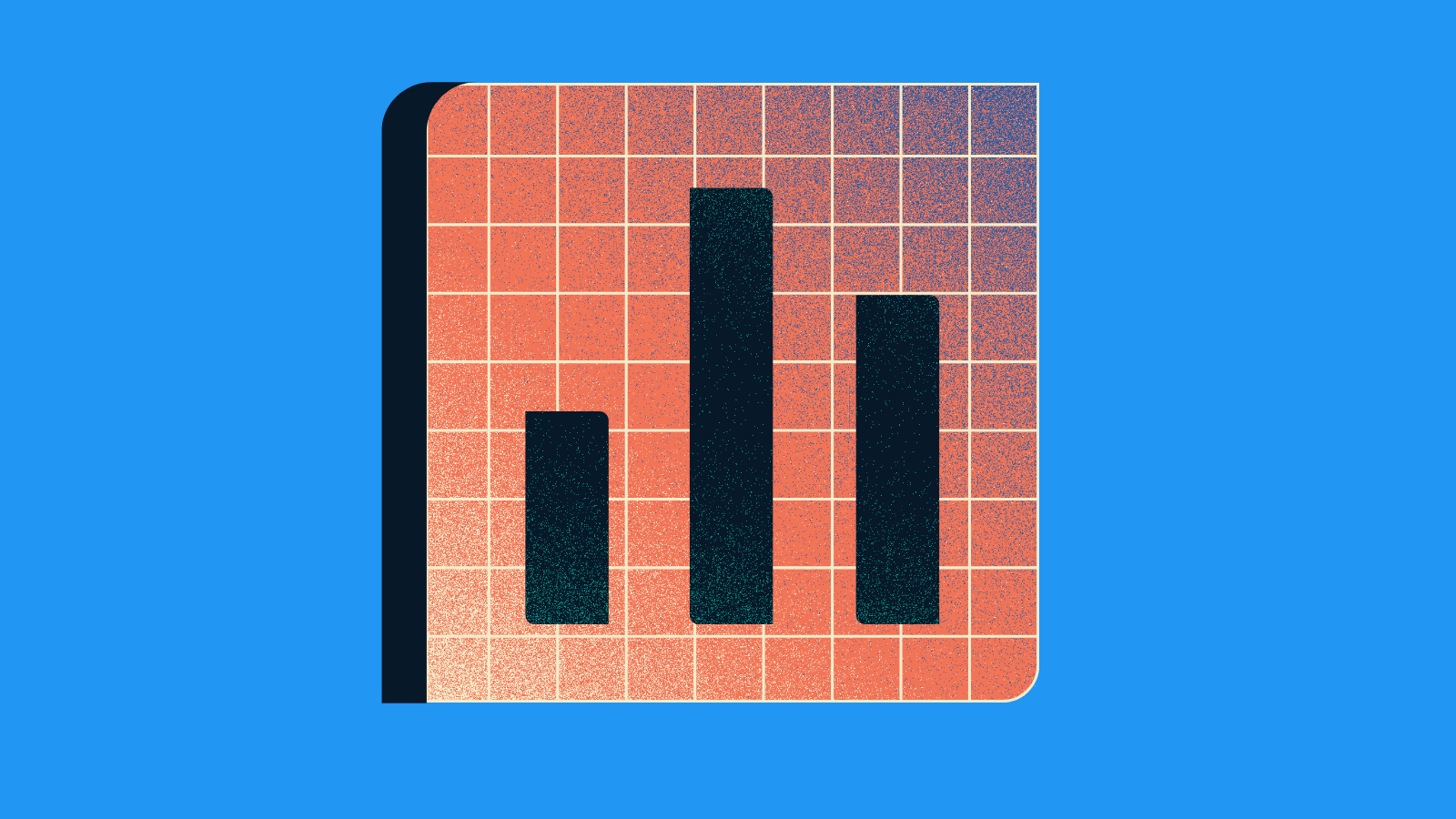
FLL Stock Forecast Model
Our team proposes a machine learning model to forecast the performance of Full House Resorts, Inc. (FLL) common stock. This model will leverage a diverse set of economic indicators and company-specific data to provide a comprehensive and data-driven outlook. We will employ a time-series forecasting methodology, incorporating both past stock performance and external factors. The model's foundation includes historical trading data (e.g., volume, intraday price movements, and volatility) and financial metrics like revenue, earnings per share (EPS), debt-to-equity ratio, and operating margins. These internal metrics will be critically assessed to understand the financial health and growth potential of the firm. Furthermore, we will integrate macroeconomic variables such as GDP growth, inflation rates, consumer confidence indices, interest rates, and employment data. The goal is to capture how the broader economic environment influences the company's performance.
To build a robust and accurate forecasting model, we will utilize a combination of machine learning algorithms. Initially, we will explore the performance of ARIMA (Autoregressive Integrated Moving Average) models and their extensions, which are widely used for time series analysis. We anticipate examining the suitability of more complex techniques, such as Recurrent Neural Networks (RNNs), particularly Long Short-Term Memory (LSTM) networks, due to their capacity for identifying long-term dependencies and complex patterns in time series data. Furthermore, we will evaluate the applicability of ensemble methods, such as Random Forests and Gradient Boosting, to leverage the predictive strength of multiple models. The model's performance will be rigorously evaluated using metrics such as Mean Absolute Error (MAE), Root Mean Squared Error (RMSE), and R-squared to ensure accuracy and reliability. We will perform backtesting using historical data, and regular model retraining will be incorporated as well to account for the dynamic nature of the markets.
The final model will provide probabilistic forecasts for the future stock performance of FLL. The output will incorporate a range of possible scenarios, providing insight into the potential upside and downside risks. The forecasts will be accompanied by clear interpretations and caveats, highlighting the limitations of the model and the uncertainty inherent in stock market predictions. Regular updates and model refinement are crucial for maintaining the forecast's accuracy, which will be based on the latest available data and market developments. We intend to publish forecasts with varying time horizons (e.g., weekly, monthly, and quarterly), allowing for adaptability to investors' varying investment strategies. The goal is to offer investors and stakeholders with a sophisticated tool for informed decision-making regarding FLL stock.
ML Model Testing
n:Time series to forecast
p:Price signals of Full House Resorts stock
j:Nash equilibria (Neural Network)
k:Dominated move of Full House Resorts stock holders
a:Best response for Full House Resorts target price
For further technical information as per how our model work we invite you to visit the article below:
How do KappaSignal algorithms actually work?
Full House Resorts Stock Forecast (Buy or Sell) Strategic Interaction Table
Strategic Interaction Table Legend:
X axis: *Likelihood% (The higher the percentage value, the more likely the event will occur.)
Y axis: *Potential Impact% (The higher the percentage value, the more likely the price will deviate.)
Z axis (Grey to Black): *Technical Analysis%
Full House Resorts Inc. (FLL) Financial Outlook and Forecast
The financial outlook for FLL presents a mixed picture, shaped by its strategic positioning in the gaming and entertainment sector, particularly its focus on regional casinos. Recent performance indicates positive trends in revenue generation, driven by a recovery in leisure travel and pent-up demand for entertainment experiences. The company's diversified portfolio, encompassing properties in multiple states, provides a degree of insulation against regional economic downturns or regulatory changes. Furthermore, FLL's management has demonstrated an ability to effectively manage operational costs, enhancing profitability. Capital investments in property improvements and expansions are expected to contribute to future revenue growth by attracting a broader customer base and offering enhanced gaming and entertainment options. However, the company's outlook is also influenced by the broader economic environment and the competitive landscape within the gaming industry.
The company's revenue forecast for the next 2-3 years anticipates continued growth, fueled by improvements in occupancy rates and increased customer spending at its properties. This growth will be particularly prominent in the areas of online sports betting and iGaming where the company's efforts are expected to bear fruit in the future. FLL's strategic acquisitions and partnerships are expected to support its expansion plans. Management's commitment to shareholder value, demonstrated through efficient capital allocation and disciplined financial management, further contributes to the positive outlook. Furthermore, the company's relatively low debt levels provide financial flexibility, which it can use to pursue further opportunities. The company's expansion plans, however, will depend on their ability to navigate the permitting and regulatory hurdles in each jurisdiction.
Key factors influencing FLL's financial performance include consumer spending patterns, the competitive intensity of the gaming market, and the impact of evolving regulations on the gaming industry. The company's success will depend on its capacity to adapt to shifting consumer preferences, technological advancements, and its ability to differentiate itself from its competitors through high-quality experiences and offerings. The company is dependent on the economic conditions in the markets it serves. The company's ability to manage labor costs and supply chain disruptions will be important to its financial success. In addition, the overall health of the economy and any changes in the regulatory environment regarding gaming activities, including the introduction of new taxes or restrictions, could affect the company's profitability.
Based on current trends and strategic initiatives, a positive outlook for FLL's financial performance is predicted for the next several years. The company is expected to benefit from increased revenue generation and enhanced profitability. However, this prediction is subject to the inherent risks associated with the gaming industry, including economic downturns, changing consumer preferences, intense competition, and fluctuations in the regulatory environment. Unforeseen events, such as significant economic recessions or negative changes in state and federal regulations could substantially impair its outlook. In addition, FLL's ability to manage its debt and maintain strong financial controls will be critical to realizing its full potential.
Rating | Short-Term | Long-Term Senior |
---|---|---|
Outlook | Baa2 | Ba3 |
Income Statement | B1 | B2 |
Balance Sheet | Baa2 | B2 |
Leverage Ratios | Ba1 | Baa2 |
Cash Flow | Baa2 | B3 |
Rates of Return and Profitability | Ba3 | Baa2 |
*Financial analysis is the process of evaluating a company's financial performance and position by neural network. It involves reviewing the company's financial statements, including the balance sheet, income statement, and cash flow statement, as well as other financial reports and documents.
How does neural network examine financial reports and understand financial state of the company?
References
- J. Z. Leibo, V. Zambaldi, M. Lanctot, J. Marecki, and T. Graepel. Multi-agent Reinforcement Learning in Sequential Social Dilemmas. In Proceedings of the 16th International Conference on Autonomous Agents and Multiagent Systems (AAMAS 2017), Sao Paulo, Brazil, 2017
- Y. Chow and M. Ghavamzadeh. Algorithms for CVaR optimization in MDPs. In Advances in Neural Infor- mation Processing Systems, pages 3509–3517, 2014.
- Hastie T, Tibshirani R, Friedman J. 2009. The Elements of Statistical Learning. Berlin: Springer
- Abadie A, Diamond A, Hainmueller J. 2015. Comparative politics and the synthetic control method. Am. J. Political Sci. 59:495–510
- Holland PW. 1986. Statistics and causal inference. J. Am. Stat. Assoc. 81:945–60
- N. B ̈auerle and J. Ott. Markov decision processes with average-value-at-risk criteria. Mathematical Methods of Operations Research, 74(3):361–379, 2011
- K. Boda and J. Filar. Time consistent dynamic risk measures. Mathematical Methods of Operations Research, 63(1):169–186, 2006