AUC Score :
Short-term Tactic1 :
Dominant Strategy :
Time series to forecast n:
ML Model Testing : Transfer Learning (ML)
Hypothesis Testing : Wilcoxon Sign-Rank Test
Surveillance : Major exchange and OTC
1Short-term revised.
2Time series is updated based on short-term trends.
Key Points
EPRT's future appears cautiously optimistic, driven by its focus on necessity-based real estate. Predictions suggest continued, albeit moderate, revenue and earnings growth, supported by lease renewals and strategic property acquisitions. The company's dividend is expected to remain a key attraction for investors, though potential interest rate hikes could pose a challenge by increasing borrowing costs and impacting overall real estate valuations. Risks include the ability to effectively manage its portfolio amidst shifting economic conditions and attract quality tenants, as well as the susceptibility of certain property types within its portfolio to changing consumer preferences. While EPRT's focus on essential properties provides a degree of insulation, any significant economic downturn or sector-specific disruption could negatively affect its performance.About Essential Properties Realty Trust
Essential Properties Realty Trust (ESS) is a real estate investment trust (REIT) specializing in the acquisition, ownership, and management of single-tenant net-lease properties. The company focuses on properties leased to tenants operating in service-oriented and necessity-based businesses. ESS primarily acquires properties across a range of industries, including restaurants, auto service, medical services, and convenience stores, among others.
ESS aims to provide consistent and growing cash flows from its diversified portfolio of net-leased properties. Their business model centers around long-term leases with established tenants, which reduces risk and provides a stable income stream. The company emphasizes disciplined capital allocation and focuses on investments in high-quality, well-located properties with strong tenant credit profiles.
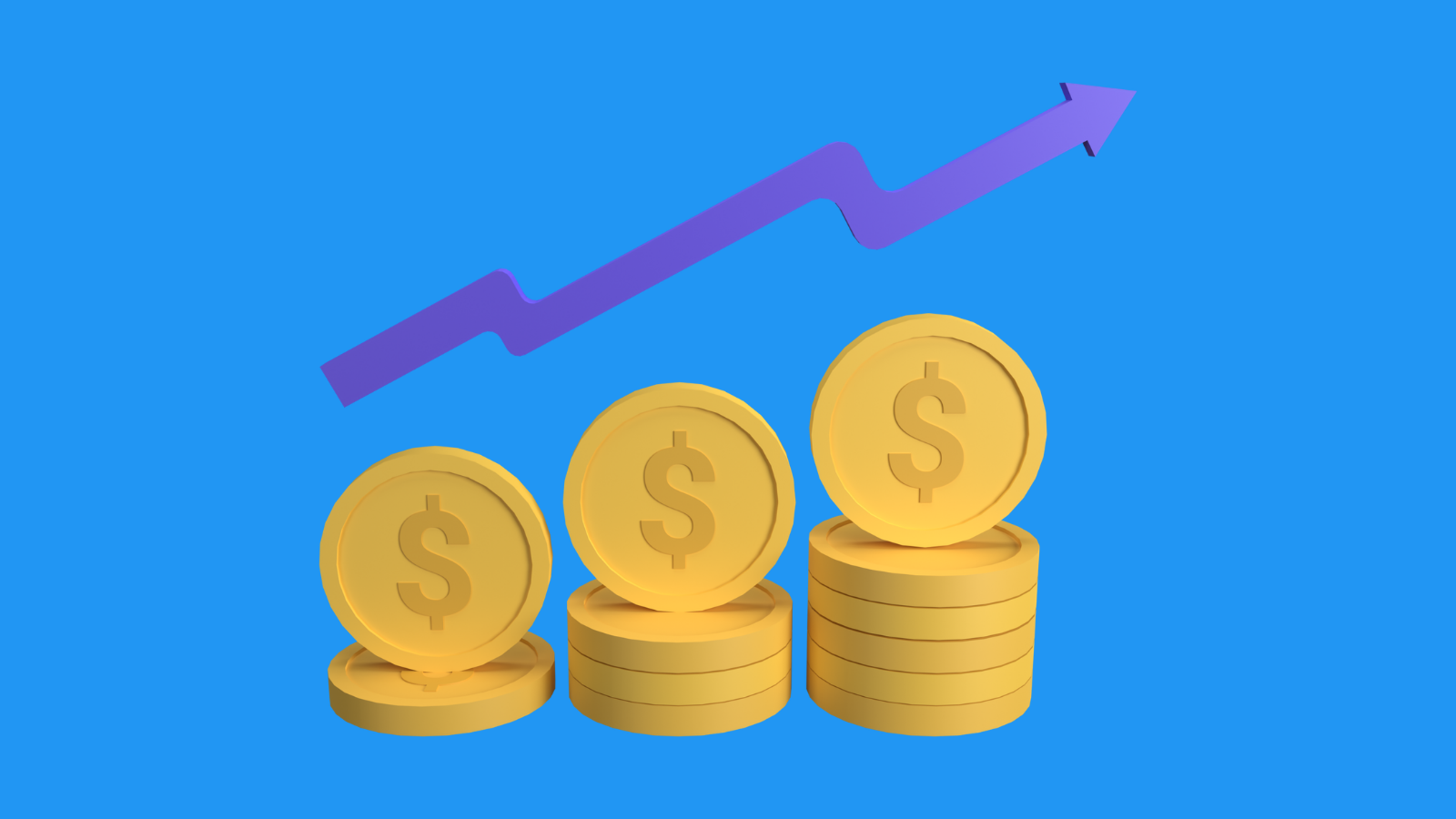
EPRT Stock Forecast Model
Our team of data scientists and economists has developed a comprehensive machine learning model to forecast the performance of Essential Properties Realty Trust Inc. (EPRT) common stock. This model integrates a diverse range of financial and economic indicators to provide robust predictions. The core of the model is a **hybrid architecture** leveraging both time series analysis and machine learning techniques. We utilize historical EPRT stock data, incorporating technical indicators such as moving averages, Relative Strength Index (RSI), and volume analysis. Concurrently, we incorporate fundamental data points, including EPRT's quarterly earnings reports, revenue growth, occupancy rates, and debt levels. Economic factors such as interest rates, inflation, and overall real estate market trends are also included as crucial inputs. We employ a sophisticated feature engineering process to optimize the predictive power of the variables, transforming raw data into features suitable for our machine learning algorithms.
For the machine learning component, we've implemented an ensemble approach, combining the strengths of various algorithms. This includes Recurrent Neural Networks (RNNs), specifically Long Short-Term Memory (LSTM) networks, which excel in capturing temporal dependencies in the stock's behavior. We also use Gradient Boosting Machines (GBMs) to identify and exploit complex relationships between the input variables and stock performance. The model is trained on a large dataset of historical data, and undergoes rigorous validation using techniques like k-fold cross-validation to ensure the robustness and generalizability of its predictions. This multi-faceted approach allows us to mitigate the risks associated with any single algorithm and provide more reliable forecasts. The model's output includes both point predictions (e.g., predicted value at a specific point in time) and probabilistic forecasts, quantifying the uncertainty around the predictions.
The final output of our model is designed to inform strategic decision-making, helping investors and analysts understand the potential future performance of EPRT. The model provides insights into the factors that are most likely to impact the stock's behavior, highlighting key opportunities and potential risks. The model's predictions will be continuously monitored and updated as new data becomes available. The ongoing validation and evaluation process ensures that the model remains accurate and effective. The model is intended to provide a valuable tool for evaluating EPRT, but should be used in conjunction with other sources of information and professional financial advice. The model will also incorporate macroeconomic forecasts and expert industry opinions to offer a holistic perspective on the stock's prospects.
ML Model Testing
n:Time series to forecast
p:Price signals of Essential Properties Realty Trust stock
j:Nash equilibria (Neural Network)
k:Dominated move of Essential Properties Realty Trust stock holders
a:Best response for Essential Properties Realty Trust target price
For further technical information as per how our model work we invite you to visit the article below:
How do KappaSignal algorithms actually work?
Essential Properties Realty Trust Stock Forecast (Buy or Sell) Strategic Interaction Table
Strategic Interaction Table Legend:
X axis: *Likelihood% (The higher the percentage value, the more likely the event will occur.)
Y axis: *Potential Impact% (The higher the percentage value, the more likely the price will deviate.)
Z axis (Grey to Black): *Technical Analysis%
Essential Properties Realty Trust Inc. (ESS) Financial Outlook and Forecast
The outlook for Essential Properties Realty Trust (ESS) appears cautiously optimistic, driven by the company's stable net lease portfolio. ESS primarily focuses on acquiring, owning, and managing single-tenant net-leased properties, primarily in the essential retail and service sectors. This strategy provides a degree of resilience during economic downturns, as the tenants occupying these properties offer essential services and products. The company benefits from long-term lease agreements that generally provide predictable cash flows. Further, ESS has demonstrated a disciplined approach to acquisitions, focusing on properties with attractive yields and strong tenant credit profiles. The company's continued expansion, both through acquisitions and organic growth, is expected to support its financial performance, however, a slowdown in acquisition activity or rising interest rates could pose challenges.
ESS's financial performance is expected to remain solid, characterized by steady revenue growth and a manageable debt profile. The company's ability to maintain a high occupancy rate is crucial to its success. This is largely influenced by the quality of its tenant base and the essential nature of the services offered. The company's Net Operating Income (NOI) should increase due to a combination of rent escalations within its existing portfolio and contributions from new acquisitions. ESS has generally shown a good track record for managing its financial leverage, utilizing a combination of debt and equity to fund its growth initiatives. Maintaining a healthy balance sheet will be critical to ensuring its long-term stability and the ability to pursue further acquisitions and growth prospects. This will be particularly important in an environment of potentially rising interest rates, which could increase borrowing costs.
ESS is expected to continue to increase dividends to shareholders in line with consistent earnings. The company's focus on net lease properties, which often have less volatility than other types of real estate, supports its dividend-paying capability. Furthermore, ESS is expected to continue to be an attractive investment for income-seeking investors. The company's ability to generate consistent cash flow and its history of returning capital to shareholders in the form of dividends are key attractions. The valuation of ESS will also be impacted by overall market conditions, including investor sentiment and the prevailing interest rate environment. Furthermore, the company's success hinges upon its ability to maintain its relationships with its tenants and adapt to evolving consumer preferences and needs.
Overall, ESS's financial outlook is positive, with continued growth anticipated, driven by a solid business model and the potential for further expansion. The company's focus on essential services and tenant credit quality should provide some insulation from economic downturns, however, this positive outlook is subject to some risks. Rising interest rates could increase borrowing costs and potentially slow down acquisition activity, thereby impacting growth. Moreover, any economic downturn could negatively affect the company's tenants and therefore rental income. Furthermore, any unexpected problems with tenants could impact occupancy rates and thus influence financial performance. Despite these risks, ESS's fundamentals appear sound, suggesting that it is well-positioned to capitalize on opportunities for long-term success.
```
Rating | Short-Term | Long-Term Senior |
---|---|---|
Outlook | B3 | B1 |
Income Statement | Ba2 | Caa2 |
Balance Sheet | C | B1 |
Leverage Ratios | Caa2 | B3 |
Cash Flow | Baa2 | Baa2 |
Rates of Return and Profitability | C | Caa2 |
*Financial analysis is the process of evaluating a company's financial performance and position by neural network. It involves reviewing the company's financial statements, including the balance sheet, income statement, and cash flow statement, as well as other financial reports and documents.
How does neural network examine financial reports and understand financial state of the company?
References
- G. Konidaris, S. Osentoski, and P. Thomas. Value function approximation in reinforcement learning using the Fourier basis. In AAAI, 2011
- Mikolov T, Chen K, Corrado GS, Dean J. 2013a. Efficient estimation of word representations in vector space. arXiv:1301.3781 [cs.CL]
- J. Filar, L. Kallenberg, and H. Lee. Variance-penalized Markov decision processes. Mathematics of Opera- tions Research, 14(1):147–161, 1989
- J. Harb and D. Precup. Investigating recurrence and eligibility traces in deep Q-networks. In Deep Reinforcement Learning Workshop, NIPS 2016, Barcelona, Spain, 2016.
- C. Wu and Y. Lin. Minimizing risk models in Markov decision processes with policies depending on target values. Journal of Mathematical Analysis and Applications, 231(1):47–67, 1999
- F. A. Oliehoek and C. Amato. A Concise Introduction to Decentralized POMDPs. SpringerBriefs in Intelligent Systems. Springer, 2016
- Meinshausen N. 2007. Relaxed lasso. Comput. Stat. Data Anal. 52:374–93