AUC Score :
Short-term Tactic1 :
Dominant Strategy :
Time series to forecast n:
ML Model Testing : Multi-Instance Learning (ML)
Hypothesis Testing : Stepwise Regression
Surveillance : Major exchange and OTC
1Short-term revised.
2Time series is updated based on short-term trends.
Key Points
ENSG is predicted to experience consistent revenue growth driven by its expansion strategy and acquisitions within the healthcare sector. Profitability is also expected to improve, supported by effective cost management and a strong occupancy rate. The primary risk lies in increased competition from larger healthcare providers and potential regulatory changes impacting reimbursement rates. Additional risks include integration challenges associated with future acquisitions, along with the potential for increased labor costs.About The Ensign Group
The Ensign Group (ENSG) is a holding company specializing in healthcare services. The company operates through a network of independently operated subsidiaries providing skilled nursing, assisted living, and other related healthcare services. Ensign's business model centers around acquiring and operating these facilities with a focus on localized management and operational expertise. Their subsidiaries are spread across multiple states, offering a range of post-acute care options. The company has a history of growth through both acquisitions and organic expansion.
ENSG's operational strategy emphasizes decentralization, empowering local management teams to make decisions. This approach aims to foster a strong sense of ownership and accountability. Ensign emphasizes quality of care, employee training, and responsiveness to the needs of patients and residents. Their financial performance is driven by factors such as occupancy rates, reimbursement policies within the healthcare industry, and their ability to successfully integrate acquired facilities. The company is focused on long-term growth in the post-acute care sector.
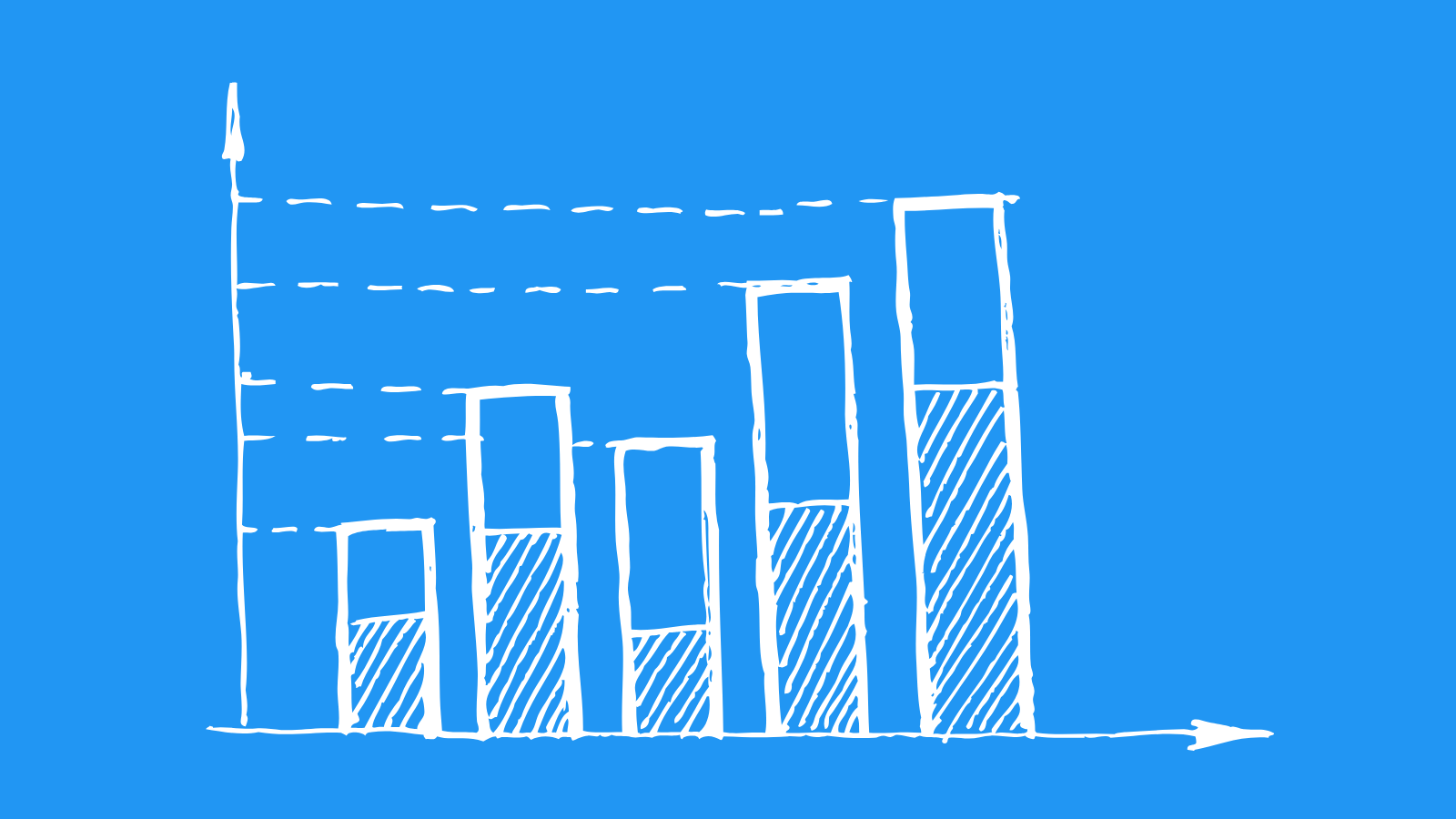
ENSG Stock Price Forecasting Machine Learning Model
Our team of data scientists and economists has developed a machine learning model designed to forecast the future performance of The Ensign Group Inc. (ENSG) stock. This model leverages a comprehensive dataset encompassing various financial and economic indicators. These include, but are not limited to, ENSG's quarterly and annual financial statements, including revenue, profit margins, and debt levels; industry-specific data such as occupancy rates in skilled nursing facilities and changes in healthcare regulations; and broader macroeconomic factors like interest rates, inflation, and overall market sentiment as reflected in indices like the S&P 500. We employ a hybrid approach, integrating time-series analysis techniques like ARIMA and Exponential Smoothing to capture temporal dependencies, alongside supervised learning algorithms such as Random Forests and Gradient Boosting to model complex non-linear relationships. Feature engineering is a critical component, where we create new variables, such as moving averages, volatility measures, and growth rates, to provide more informative inputs for the model.
The model's architecture is built upon a rigorous cross-validation framework to ensure robustness and prevent overfitting. We have established a training dataset that includes historical data from several years, split into distinct training, validation, and testing sets. The model's performance is evaluated using metrics appropriate for time series forecasting, including Mean Absolute Error (MAE), Root Mean Squared Error (RMSE), and Mean Absolute Percentage Error (MAPE). Regular model retraining is a key feature of our approach; we plan to incorporate the most recent data to maintain predictive accuracy, mitigating any potential impact of evolving market conditions. Furthermore, the model's output is accompanied by an assessment of forecast confidence intervals to provide a range of potential outcomes, accounting for the inherent uncertainty in financial markets. Scenario analysis is also planned by incorporating potential future data and trends.
The ultimate deliverable will be a user-friendly interface that presents the ENSG stock forecast, along with key contributing factors and associated risk assessments. The forecast will provide insight into the direction of the stock's price movement over a specified time horizon. Our team is continuously working to enhance the model, including the incorporation of alternative datasets, like ESG scores and social media sentiment analysis, to create a more holistic understanding of the drivers influencing the stock's future performance. The model is designed to act as a supplementary tool for investment decision-making, not a replacement for thorough fundamental analysis. Finally, a rigorous backtesting process will be used to ensure the model's performance and make adjustments if needed, and to avoid any bias.
ML Model Testing
n:Time series to forecast
p:Price signals of The Ensign Group stock
j:Nash equilibria (Neural Network)
k:Dominated move of The Ensign Group stock holders
a:Best response for The Ensign Group target price
For further technical information as per how our model work we invite you to visit the article below:
How do KappaSignal algorithms actually work?
The Ensign Group Stock Forecast (Buy or Sell) Strategic Interaction Table
Strategic Interaction Table Legend:
X axis: *Likelihood% (The higher the percentage value, the more likely the event will occur.)
Y axis: *Potential Impact% (The higher the percentage value, the more likely the price will deviate.)
Z axis (Grey to Black): *Technical Analysis%
ENSG Financial Outlook and Forecast
ENSG, a prominent operator of healthcare services, exhibits a generally positive financial outlook, driven by several key factors. Demographic trends, particularly the aging population in developed countries, fuel consistent demand for the company's core services, including skilled nursing facilities, assisted living facilities, and independent living facilities. Furthermore, ENSG's strategic approach to acquiring and integrating facilities, often involving operational improvements and enhanced efficiency, allows for organic revenue and profitability growth. The company's focus on local market expertise and decentralized management structure contributes to agility and responsiveness to regional healthcare needs. ENSG's strong balance sheet, demonstrated by manageable debt levels and healthy cash flow generation, provides a solid foundation for continued investment in acquisitions and capital expenditures, further supporting its long-term growth trajectory. The organization consistently demonstrates its adaptability through its focus on high-quality care and its ability to address diverse patient needs. This strategic positioning positions ENSG favorably within the healthcare landscape.
The company's forecast suggests continued revenue growth, driven by both organic increases in occupancy and revenue per patient and through strategic acquisitions. ENSG's management has a proven track record of identifying and integrating acquisitions successfully. This includes operational improvements and cost synergies. The forecast incorporates expectations of rising demand for specialized services, such as rehabilitation and memory care, which offer higher revenue and profitability. Furthermore, the ongoing transition towards value-based care models may offer favorable opportunities for ENSG, as the company's focus on quality and patient outcomes aligns well with the goals of these payment systems. The company's consistent execution and financial prudence supports the expectation of solid earnings growth. Additionally, the company's demonstrated ability to navigate industry regulations and changes in reimbursement models suggests a resilience to the challenges inherent in the healthcare sector.
Several operational and market considerations could impact ENSG's financial performance. Healthcare reimbursement rates, primarily from government and managed care programs, are subject to change, and any downward pressure on these rates could negatively affect revenue and margins. Competition within the healthcare sector is intense, and increased competition from other skilled nursing operators, hospitals, and home healthcare providers could erode market share and potentially constrain pricing power. Changes in regulations related to staffing, quality of care, and licensing can increase operating costs. These operational risks could affect the financial performance of ENSG, potentially impacting its revenue, margins, and overall profitability. The ongoing labor shortages in the healthcare industry and rising labor costs represent a significant operational challenge. Also, potential risks are involved in integrating acquired facilities, including operational disruptions and unexpected costs.
The forecast for ENSG is generally positive, anticipating continued growth driven by favorable demographic trends, strategic acquisitions, and operational improvements. The company's proven ability to navigate the healthcare landscape and its strong financial foundation support this optimistic view. However, the company faces inherent risks associated with reimbursement rate changes, competitive pressures, and evolving regulations. A prolonged downturn in reimbursement rates or a significant increase in labor costs could significantly impact ENSG's profitability, potentially leading to a negative short-term outlook. Careful monitoring of industry trends, continued focus on operational efficiency, and strategic adaptation to market changes will be crucial for ENSG to achieve its financial goals.
Rating | Short-Term | Long-Term Senior |
---|---|---|
Outlook | Ba1 | B1 |
Income Statement | Baa2 | Ba1 |
Balance Sheet | Baa2 | C |
Leverage Ratios | Ba2 | Baa2 |
Cash Flow | Baa2 | Baa2 |
Rates of Return and Profitability | Ba3 | C |
*Financial analysis is the process of evaluating a company's financial performance and position by neural network. It involves reviewing the company's financial statements, including the balance sheet, income statement, and cash flow statement, as well as other financial reports and documents.
How does neural network examine financial reports and understand financial state of the company?
References
- Kitagawa T, Tetenov A. 2015. Who should be treated? Empirical welfare maximization methods for treatment choice. Tech. Rep., Cent. Microdata Methods Pract., Inst. Fiscal Stud., London
- A. Tamar and S. Mannor. Variance adjusted actor critic algorithms. arXiv preprint arXiv:1310.3697, 2013.
- M. Babes, E. M. de Cote, and M. L. Littman. Social reward shaping in the prisoner's dilemma. In 7th International Joint Conference on Autonomous Agents and Multiagent Systems (AAMAS 2008), Estoril, Portugal, May 12-16, 2008, Volume 3, pages 1389–1392, 2008.
- C. Claus and C. Boutilier. The dynamics of reinforcement learning in cooperative multiagent systems. In Proceedings of the Fifteenth National Conference on Artificial Intelligence and Tenth Innovative Applications of Artificial Intelligence Conference, AAAI 98, IAAI 98, July 26-30, 1998, Madison, Wisconsin, USA., pages 746–752, 1998.
- Imbens GW, Rubin DB. 2015. Causal Inference in Statistics, Social, and Biomedical Sciences. Cambridge, UK: Cambridge Univ. Press
- R. Sutton and A. Barto. Introduction to reinforcement learning. MIT Press, 1998
- S. Proper and K. Tumer. Modeling difference rewards for multiagent learning (extended abstract). In Proceedings of the Eleventh International Joint Conference on Autonomous Agents and Multiagent Systems, Valencia, Spain, June 2012