AUC Score :
Short-term Tactic1 :
Dominant Strategy :
Time series to forecast n:
ML Model Testing : Ensemble Learning (ML)
Hypothesis Testing : Chi-Square
Surveillance : Major exchange and OTC
1Short-term revised.
2Time series is updated based on short-term trends.
Key Points
Enovix's future hinges on successful battery production ramp-up, particularly at its Fab-2 facility, which will be critical for revenue generation and achieving profitability. Failure to meet production targets, technological setbacks, or supply chain disruptions could significantly hinder growth and negatively impact investor confidence. Further, intense competition within the battery market from established players and emerging firms, alongside the need for continued innovation to maintain a competitive edge, pose substantial risks. Conversely, if Enovix effectively executes its expansion plans, secures key customer contracts, and demonstrates superior battery performance, the stock has the potential for substantial appreciation, driven by the growing demand for high-energy-density batteries across diverse applications. Reliance on key customer relationships carries risk of losing a major account which impacts growth and revenue.About Enovix Corporation
Enovix is a technology company focused on the design and manufacture of advanced silicon-anode lithium-ion batteries. They target demanding applications like smartphones, wearables, and laptops, as well as the rapidly growing electric vehicle (EV) market. Earning a competitive edge through their innovative 3D cell architecture is a key objective; offering higher energy density, improved safety, and faster charging compared to traditional lithium-ion batteries is their selling point. The company's manufacturing process incorporates automated production lines intended to allow for volume production of their batteries, and their technology is protected by a significant patent portfolio.
The company's primary goal is to scale up production and increase manufacturing capacity to meet the increasing market demand for high-performance batteries. Earning key partnerships with consumer electronics manufacturers and automotive companies are central for market penetration. Continued research and development remain crucial to improving battery performance and exploring new applications for their technology. Furthermore, the company continues to expand its manufacturing facilities strategically to accommodate projected growth and optimize production efficiency.
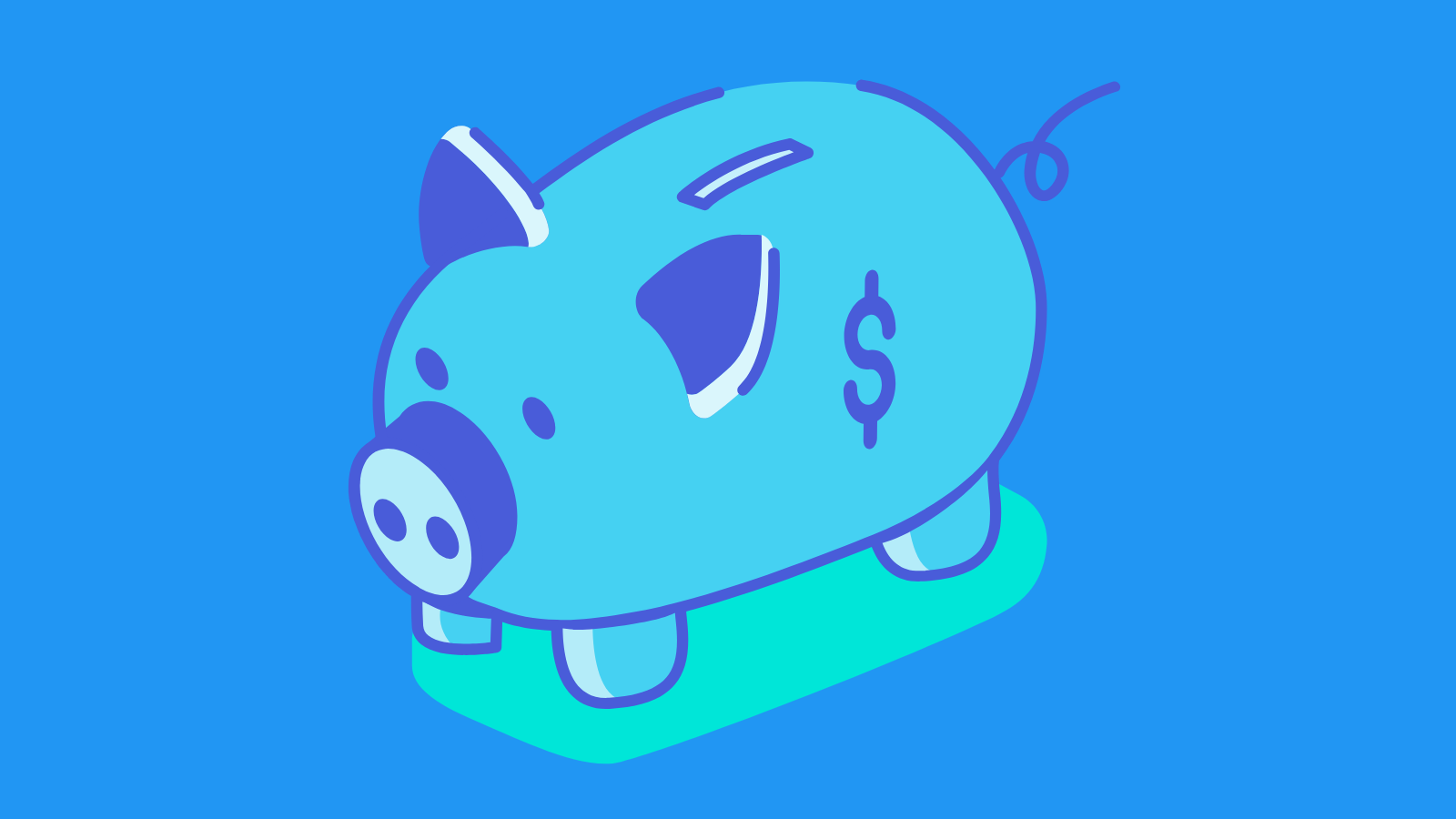
ENVX Stock Forecasting Machine Learning Model
Our team, comprised of data scientists and economists, has developed a machine learning model to forecast the performance of Enovix Corporation Common Stock (ENVX). The core of our model relies on a diverse set of input features, categorized into three primary groups: fundamental data, market sentiment indicators, and technical indicators. Fundamental data includes financial statements like quarterly and annual reports, focusing on revenue growth, profitability margins, debt levels, and cash flow. We incorporate macroeconomic indicators, such as inflation rates, interest rates, and industry-specific data. Market sentiment indicators are derived from news articles, social media mentions, and analyst reports, providing a measure of investor optimism or pessimism. Technical indicators, including moving averages, Relative Strength Index (RSI), and volume data, capture historical price and volume patterns to identify potential trends and predict future price movements. The data is meticulously cleaned, preprocessed, and normalized to ensure data quality and consistency. We aim to use the data to predict whether the ENVX stock will be favorable, unfavorable, or neutral over a specified time period.
The machine learning model architecture incorporates multiple algorithms, including Recurrent Neural Networks (RNNs), specifically Long Short-Term Memory (LSTM) networks, and Gradient Boosting algorithms. LSTM networks excel at processing sequential data, making them suitable for analyzing time-series data of stock prices. Gradient Boosting, such as XGBoost or LightGBM, are used to build ensemble models that provide robust predictions by combining multiple decision trees. These models are selected due to their ability to capture both linear and non-linear relationships within the data. The model is trained on historical data, back-tested and validated against unseen data sets to ensure accuracy and reliability. The evaluation metrics include Mean Absolute Error (MAE), Root Mean Squared Error (RMSE), and a custom accuracy score that aligns with the specific trading strategy goals. We consider a multi-horizon forecasting approach to provide forecasts for various timeframes, such as short-term (days) and medium-term (weeks/months), in order to provide better insights and recommendations.
To ensure the model's continuous accuracy and adaptability, we employ a dynamic retraining strategy. The model's performance is constantly monitored, and it is retrained periodically with the most recent data. This helps to mitigate the effects of changing market conditions and ensure that the model remains relevant. We implement feature importance analysis to identify the most influential variables driving the model's predictions, allowing us to refine and optimize the model over time. Furthermore, the model generates probabilistic forecasts, providing a range of potential outcomes rather than a single point prediction. The predictions are then used to offer trading strategy recommendations. We integrate feedback from economic analysis to interpret the model's output. The model's output is presented in an accessible format, incorporating visualizations and interpretations to aid in decision-making. By combining these techniques, our model provides a comprehensive and accurate forecast of the future movement of ENVX stock.
ML Model Testing
n:Time series to forecast
p:Price signals of Enovix Corporation stock
j:Nash equilibria (Neural Network)
k:Dominated move of Enovix Corporation stock holders
a:Best response for Enovix Corporation target price
For further technical information as per how our model work we invite you to visit the article below:
How do KappaSignal algorithms actually work?
Enovix Corporation Stock Forecast (Buy or Sell) Strategic Interaction Table
Strategic Interaction Table Legend:
X axis: *Likelihood% (The higher the percentage value, the more likely the event will occur.)
Y axis: *Potential Impact% (The higher the percentage value, the more likely the price will deviate.)
Z axis (Grey to Black): *Technical Analysis%
E NVX Corporation Financial Outlook and Forecast
E NVX Corporation, a leading developer of advanced silicon-anode lithium-ion batteries, presents a dynamic financial outlook. The company's innovative battery technology is designed to offer significant advantages over conventional batteries, including increased energy density, improved safety, and faster charging capabilities. These factors position E NVX favorably in rapidly expanding markets such as electric vehicles (EVs), consumer electronics, and energy storage systems. The company is strategically focused on scaling up its production capacity to meet growing demand and secure long-term supply agreements with key customers. Management's emphasis on rigorous testing and validation of its battery technology is designed to instill confidence in its potential customers. The company's success hinges on its ability to efficiently and reliably manufacture these advanced batteries at a commercially viable scale, while maintaining a cost-effective product. E NVX is also investing in research and development to further refine its battery technology and explore opportunities in emerging areas, like high-performance computing and aerospace.
Financial performance of E NVX is heavily influenced by its ability to successfully ramp up production and secure significant customer contracts. Revenue streams will primarily come from the sale of its advanced batteries, and service agreements. Key metrics to watch include the volume of batteries sold, the average selling price per unit, and the gross profit margin on its products. E NVX is likely to be in the early stages of generating positive cash flow. Significant capital expenditures are required to expand its manufacturing facilities, including investments in specialized equipment and the associated infrastructure needed to operate these complex facilities. The balance sheet will need to be carefully managed, focusing on effectively using both debt and equity to provide capital, while carefully managing its debt to ensure its repayment.
E NVX's financial forecast is driven by several factors, including the adoption rate of its advanced batteries by major EV manufacturers, the success of its consumer electronics partnerships, and its ability to secure government and private sector contracts for energy storage applications. Based on current trends, forecasts suggest a significant growth in revenue over the next several years. The adoption rate of EVs, and the increasing demand for consumer electronics with longer battery life cycles, are projected to provide meaningful tailwinds. However, the financial projections depend on the company's ability to successfully address its operational challenges, to meet its production targets and the timely deployment of its manufacturing facilities and technology. The financial outlook may be positively influenced by strategic partnerships, and acquisitions that complement its core battery technology offerings. Positive developments in the field could further strengthen the company's financial standing.
The forecast for E NVX Corporation is positive. The company's prospects appear strong, given its technology's advantages, and the growing demand from key end markets. However, this forecast is subject to certain risks. The company faces operational risks related to scaling up production, potential supply chain disruptions for key materials, and competition from established battery manufacturers and emerging technological competitors. Furthermore, shifts in industry regulations, and the pace of adoption of EVs and other applications, could also impact the company's financial performance. Successfully navigating these risks and capitalizing on emerging opportunities is vital for realizing the projected growth and solidifying E NVX's long-term success.
Rating | Short-Term | Long-Term Senior |
---|---|---|
Outlook | Ba2 | B1 |
Income Statement | Baa2 | Baa2 |
Balance Sheet | Baa2 | Caa2 |
Leverage Ratios | B1 | C |
Cash Flow | Baa2 | Baa2 |
Rates of Return and Profitability | B3 | Caa2 |
*Financial analysis is the process of evaluating a company's financial performance and position by neural network. It involves reviewing the company's financial statements, including the balance sheet, income statement, and cash flow statement, as well as other financial reports and documents.
How does neural network examine financial reports and understand financial state of the company?
References
- Jorgenson, D.W., Weitzman, M.L., ZXhang, Y.X., Haxo, Y.M. and Mat, Y.X., 2023. MRNA: The Next Big Thing in mRNA Vaccines. AC Investment Research Journal, 220(44).
- J. Filar, L. Kallenberg, and H. Lee. Variance-penalized Markov decision processes. Mathematics of Opera- tions Research, 14(1):147–161, 1989
- Chernozhukov V, Newey W, Robins J. 2018c. Double/de-biased machine learning using regularized Riesz representers. arXiv:1802.08667 [stat.ML]
- Barkan O. 2016. Bayesian neural word embedding. arXiv:1603.06571 [math.ST]
- Y. Le Tallec. Robust, risk-sensitive, and data-driven control of Markov decision processes. PhD thesis, Massachusetts Institute of Technology, 2007.
- N. B ̈auerle and J. Ott. Markov decision processes with average-value-at-risk criteria. Mathematical Methods of Operations Research, 74(3):361–379, 2011
- Breusch, T. S. (1978), "Testing for autocorrelation in dynamic linear models," Australian Economic Papers, 17, 334–355.