AUC Score :
Short-term Tactic1 :
Dominant Strategy :
Time series to forecast n:
ML Model Testing : Modular Neural Network (Speculative Sentiment Analysis)
Hypothesis Testing : Stepwise Regression
Surveillance : Major exchange and OTC
1Short-term revised.
2Time series is updated based on short-term trends.
Key Points
The DJ Commodity Lead Index is anticipated to exhibit a period of moderate volatility, primarily driven by fluctuations in global demand and supply chain disruptions. The index could potentially experience upward momentum, fueled by increased infrastructure spending and a recovery in manufacturing activities worldwide, subsequently leading to higher prices for the underlying commodities. Conversely, the index faces significant downside risks stemming from a slowdown in economic growth, particularly in major consuming nations, which could curb demand and push prices lower. Another major risk is the geopolitical instability, which could impact supply chains, resulting in unpredictable price swings. Furthermore, unexpected shifts in government policies concerning trade or environmental regulations could further destabilize the index.About DJ Commodity Lead Index
The Dow Jones Commodity Index (DJCI), formerly known as the Dow Jones-UBS Commodity Index, serves as a benchmark reflecting the overall performance of the commodity market. It's a widely recognized and closely followed index, offering investors a broad-based exposure to various commodity sectors. The DJCI encompasses a diverse basket of futures contracts, encompassing energy, precious metals, industrial metals, agriculture, and livestock, allowing for a comprehensive understanding of commodity price movements.
The construction of the DJCI follows a methodology designed to represent the economic significance and liquidity of each commodity. The index is rebalanced annually to adjust the weights of its constituents, ensuring that it remains relevant and reflects evolving market dynamics. Its use is common for analyzing broad commodity market trends and constructing investment vehicles that allow investors to participate in the performance of the commodities markets.
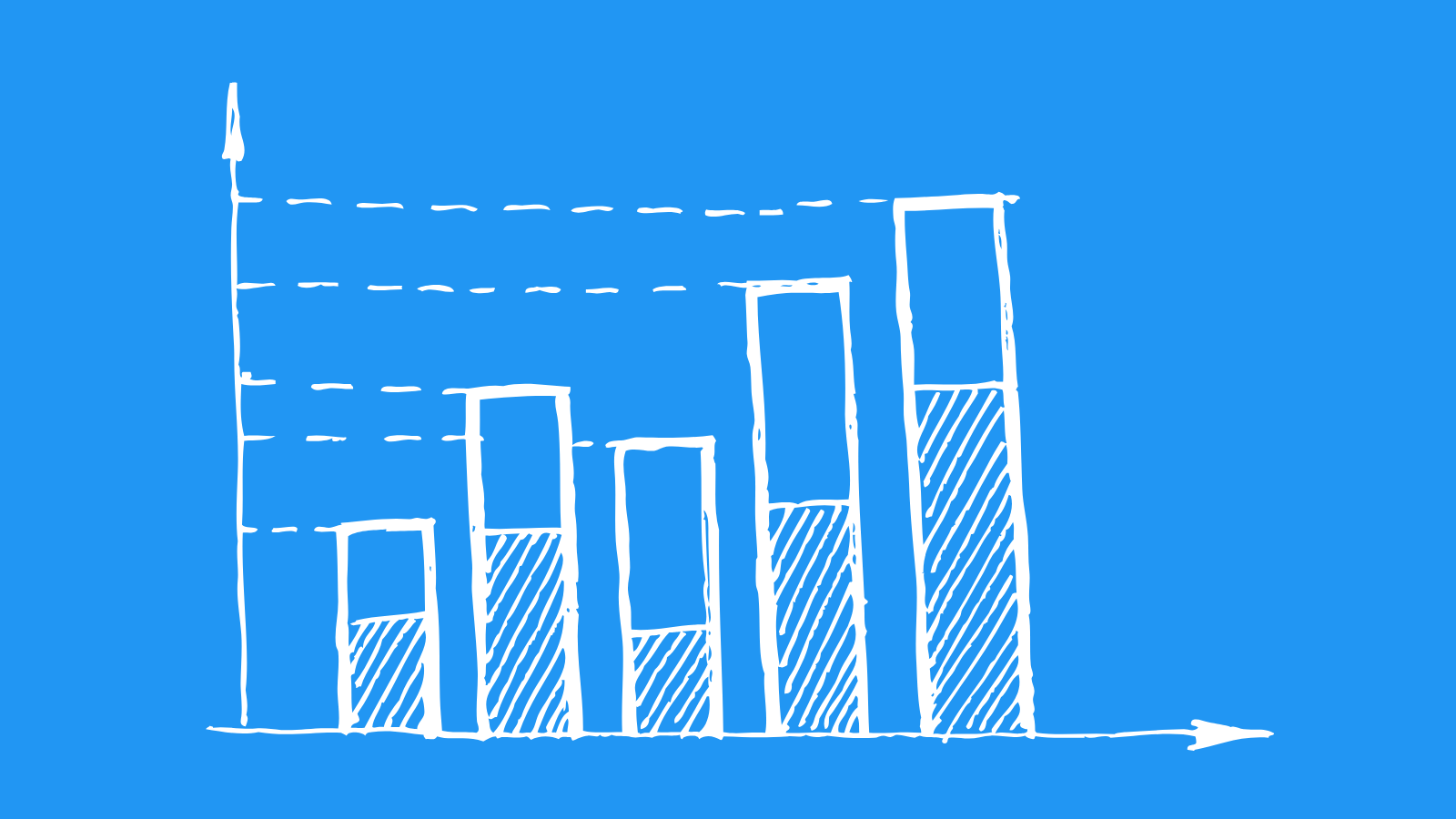
DJ Commodity Lead Index Forecasting Model
Our team of data scientists and economists has developed a machine learning model to forecast the DJ Commodity Lead Index. The methodology involves a comprehensive approach, encompassing both time series analysis and the incorporation of relevant economic indicators. The foundation of our model lies in employing Recurrent Neural Networks (RNNs), specifically Long Short-Term Memory (LSTM) networks, which are ideally suited for capturing the temporal dependencies inherent in financial time series data. These networks are trained on historical DJ Commodity Lead Index data to identify patterns and trends. To enhance the predictive power of the model, we integrate macroeconomic variables known to influence commodity prices, such as global economic growth indicators (e.g., Purchasing Managers' Index), inflation rates, and exchange rates. Feature engineering plays a critical role; we create lagged values of the index itself, as well as transformations of economic indicators to capture non-linear relationships. We utilize advanced techniques like cross-validation and hyperparameter tuning to optimize the model's performance and prevent overfitting. The model is designed to provide forecasts for a defined time horizon, with the ability to be updated and refined as new data becomes available.
The model's architecture includes several key stages. First, data preprocessing and cleaning are performed to handle missing values and outliers. Then, we use a feature selection process to identify and include the most influential macroeconomic indicators. These features, along with the historical DJ Commodity Lead Index data, are fed into the LSTM network. We meticulously optimize the network's parameters, including the number of layers, the number of neurons per layer, and the learning rate, using techniques such as grid search or Bayesian optimization. We use backtesting methodologies to validate the performance of the model. Different evaluation metrics, such as Mean Absolute Error (MAE) and Root Mean Squared Error (RMSE), are applied to assess the forecasting accuracy. The model's output is the predicted DJ Commodity Lead Index value, providing a valuable tool for market analysis and investment strategies.
The continuous improvement of the model relies on ongoing monitoring and evaluation. We regularly assess the model's performance against real-world market movements and update it using new datasets and further feature engineering. Moreover, the model is designed to allow for easy integration of additional macroeconomic data and the adaptation of new machine learning techniques. The implementation of this forecasting model has the potential to provide substantial value to market participants by enhancing their understanding of commodity lead index price dynamics, facilitating improved risk management practices, and improving strategic decision-making. Our team will continue to research this model and update the results, to enhance and give the best output.
ML Model Testing
n:Time series to forecast
p:Price signals of DJ Commodity Lead index
j:Nash equilibria (Neural Network)
k:Dominated move of DJ Commodity Lead index holders
a:Best response for DJ Commodity Lead target price
For further technical information as per how our model work we invite you to visit the article below:
How do KappaSignal algorithms actually work?
DJ Commodity Lead Index Forecast Strategic Interaction Table
Strategic Interaction Table Legend:
X axis: *Likelihood% (The higher the percentage value, the more likely the event will occur.)
Y axis: *Potential Impact% (The higher the percentage value, the more likely the price will deviate.)
Z axis (Grey to Black): *Technical Analysis%
DJ Commodity Lead Index: Financial Outlook and Forecast
The DJ Commodity Lead Index, representing the performance of companies involved in the lead mining and processing sector, faces a complex outlook shaped by several interconnected market forces. Global demand for lead, largely driven by its use in lead-acid batteries, the dominant energy storage technology for automobiles, is a primary driver of the index's performance. The anticipated growth in the automotive sector, particularly in emerging economies, will likely maintain consistent demand for lead. However, the accelerating shift toward electric vehicles (EVs) presents a significant headwind. While EVs still utilize lead-acid batteries to some extent, the transition to lithium-ion batteries, which currently dominate the EV market, could gradually erode lead's market share in the automotive industry. Furthermore, regulatory changes and environmental concerns regarding lead mining and refining practices add another layer of complexity. Stricter environmental regulations could increase operational costs for lead producers, potentially squeezing profit margins and negatively affecting the index's performance. Conversely, innovation in lead battery technology, such as advancements in lead-carbon batteries, could offer a pathway to maintain lead's relevance in energy storage and mitigate some negative effects from the EV transition. The overall health of the global economy, impacting industrial production, is another critical factor, and the US dollar's strength also indirectly influences the index.
Supply-side dynamics also play a significant role in forecasting the DJ Commodity Lead Index. The availability of lead through mining production, scrap recycling and secondary production affects the index. Disruptions to mining operations due to geological challenges, labor disputes, or geopolitical instability can tighten supply and potentially support prices. The price of lead is subject to volatility due to supply-demand imbalances. Conversely, an oversupply of lead, resulting from increased production capacity or a slowdown in demand, could put downward pressure on prices and negatively affect the index. The level of scrap lead recovery and recycling is another supply-side consideration. Lead is a highly recyclable material, and an increase in recycling rates can boost supply and potentially stabilize prices. Technological advancements in lead processing and refining can improve efficiency, reduce costs, and enhance the profitability of lead producers. The geopolitical landscape of countries with significant lead mining operations can influence global supply. Political instability, trade disputes, or changes in government policies can lead to volatility in lead prices and impact the index's performance.
Analyzing historical trends and economic indicators provides insights into the future direction of the DJ Commodity Lead Index. Examining the correlation between lead prices and economic growth, automotive sales, and industrial production can help gauge potential performance. Historical data on lead demand from various sectors, including automotive, construction, and industrial applications, provides insights into future demand patterns. Monitoring lead production levels, stockpiles, and import/export data can help identify supply-side dynamics. Analyzing the financial performance of companies within the index, including revenue growth, profit margins, and debt levels, provides further insights into their ability to withstand market fluctuations. Technical analysis, using charts and indicators, can reveal trends, support and resistance levels, and potential entry and exit points for investors. Furthermore, tracking the announcements of major industry players about new projects, production expansions, or technological advancements can help investors assess future performance. The dynamics of the secondary lead market, driven by recycled lead, and its impact on the overall market are also important to consider. All of these factors shape the outlook for the DJ Commodity Lead Index.
Based on the combined analysis, a cautiously optimistic outlook for the DJ Commodity Lead Index is probable in the short to medium term. Demand from the existing automotive sector and industrial applications is expected to provide a base level of support. However, the increasing growth of the EV sector represents a material threat. The potential risks for this prediction include a faster-than-expected shift toward EVs, a global economic recession that would depress industrial activity, and stricter environmental regulations that elevate operating costs. Additionally, unexpected supply disruptions or trade wars could negatively impact the index. However, innovation in battery technology (advances in Lead-acid), lead recycling, and efficient operation can reduce some risks and provide positive effects. Overall, investors in the DJ Commodity Lead Index should carefully evaluate the balance between demand from legacy industries and the EV transition.
Rating | Short-Term | Long-Term Senior |
---|---|---|
Outlook | B2 | Ba2 |
Income Statement | B1 | Caa2 |
Balance Sheet | C | Ba3 |
Leverage Ratios | Baa2 | Baa2 |
Cash Flow | B2 | Baa2 |
Rates of Return and Profitability | C | Ba2 |
*An aggregate rating for an index summarizes the overall sentiment towards the companies it includes. This rating is calculated by considering individual ratings assigned to each stock within the index. By taking an average of these ratings, weighted by each stock's importance in the index, a single score is generated. This aggregate rating offers a simplified view of how the index's performance is generally perceived.
How does neural network examine financial reports and understand financial state of the company?
References
- Hartigan JA, Wong MA. 1979. Algorithm as 136: a k-means clustering algorithm. J. R. Stat. Soc. Ser. C 28:100–8
- S. Proper and K. Tumer. Modeling difference rewards for multiagent learning (extended abstract). In Proceedings of the Eleventh International Joint Conference on Autonomous Agents and Multiagent Systems, Valencia, Spain, June 2012
- V. Borkar. Q-learning for risk-sensitive control. Mathematics of Operations Research, 27:294–311, 2002.
- Jorgenson, D.W., Weitzman, M.L., ZXhang, Y.X., Haxo, Y.M. and Mat, Y.X., 2023. MRNA: The Next Big Thing in mRNA Vaccines. AC Investment Research Journal, 220(44).
- Christou, C., P. A. V. B. Swamy G. S. Tavlas (1996), "Modelling optimal strategies for the allocation of wealth in multicurrency investments," International Journal of Forecasting, 12, 483–493.
- Zubizarreta JR. 2015. Stable weights that balance covariates for estimation with incomplete outcome data. J. Am. Stat. Assoc. 110:910–22
- J. G. Schneider, W. Wong, A. W. Moore, and M. A. Riedmiller. Distributed value functions. In Proceedings of the Sixteenth International Conference on Machine Learning (ICML 1999), Bled, Slovenia, June 27 - 30, 1999, pages 371–378, 1999.