AUC Score :
Short-term Tactic1 :
Dominant Strategy :
Time series to forecast n:
ML Model Testing : Modular Neural Network (DNN Layer)
Hypothesis Testing : Linear Regression
Surveillance : Major exchange and OTC
1Short-term revised.
2Time series is updated based on short-term trends.
Key Points
The DJ Commodity index is anticipated to experience moderate growth, fueled by sustained demand in energy and agricultural sectors, though increased volatility is expected. Base metals may see modest gains, constrained by supply chain bottlenecks and macroeconomic uncertainties, but the agricultural sector is likely to show resilience, driven by strong consumption patterns and potentially adverse weather conditions in key growing regions. Risks include potential downturns due to rising interest rates, geopolitical tensions impacting energy supplies, and the possibility of economic slowdowns in major consumer markets. Further potential threats include unexpected shifts in global trade policies and the emergence of alternative technologies that affect commodity demand.About DJ Commodity Index
The Dow Jones Commodity Index (DJCI) is a broad, diversified index designed to reflect the performance of the commodity market. It provides exposure to a wide array of commodities, encompassing various sectors like energy, agriculture, precious metals, and industrial metals. This diversified approach aims to offer investors a comprehensive view of the commodity asset class, mitigating the concentration risk often associated with single-commodity investments. The DJCI serves as a benchmark for commodity market performance and is used as a basis for investment products, allowing investors to gain exposure to this important asset class.
The index is constructed using a rules-based methodology, ensuring transparency and replicability. The weighting of individual commodities within the DJCI is determined based on their liquidity and economic significance, with no single commodity dominating the index. The index is rebalanced periodically to reflect changes in the commodity markets and to maintain its diversification across different commodity sectors. This dynamic rebalancing ensures that the DJCI remains a relevant and reliable measure of commodity market performance over time.
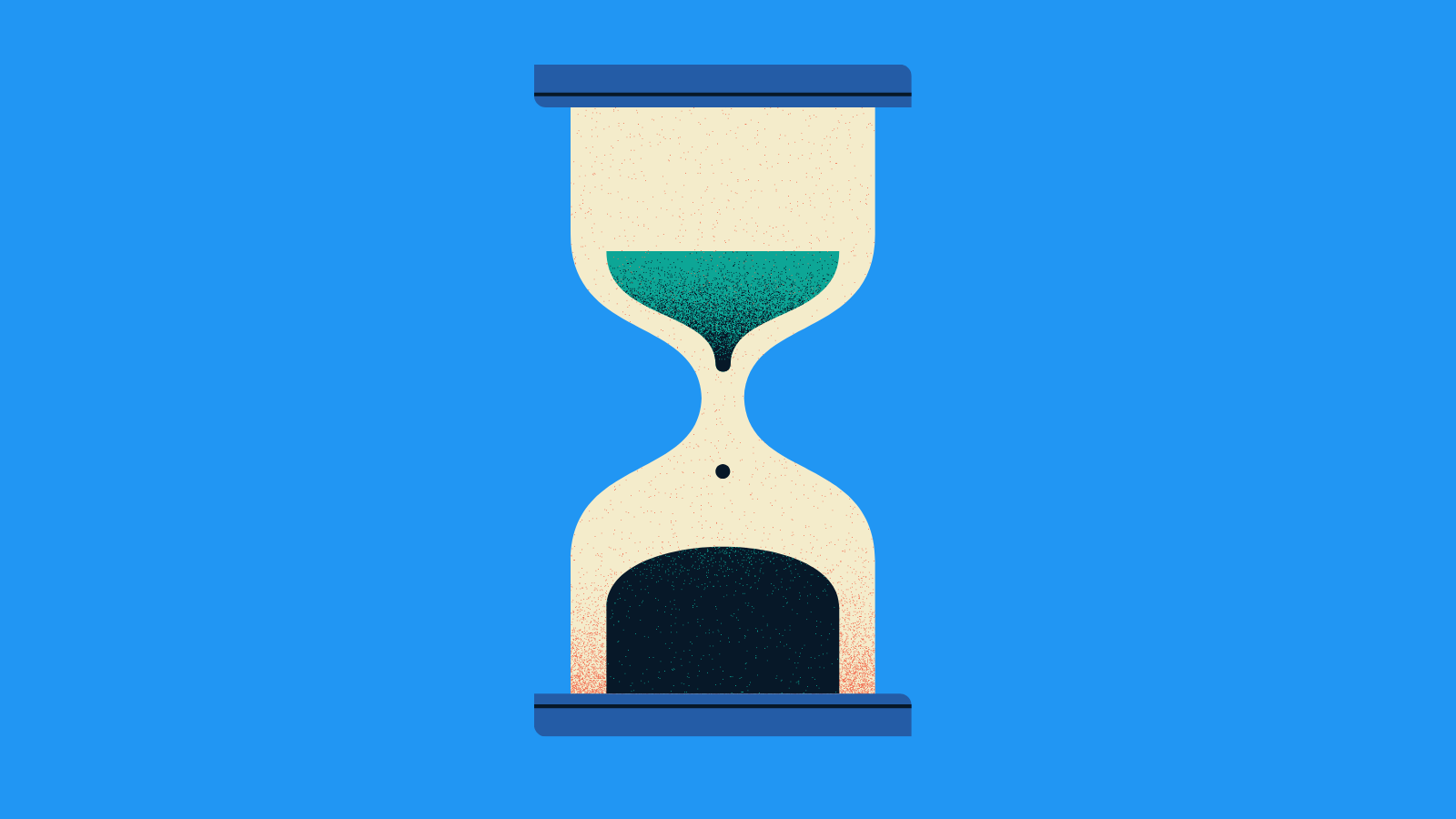
DJ Commodity Index Forecasting Machine Learning Model
The development of a robust forecasting model for the Dow Jones Commodity Index (DJCI) necessitates a multi-faceted approach that integrates both economic principles and advanced machine learning techniques. Our team proposes a model that leverages a combination of time series analysis, macroeconomic indicators, and external market data. The core of the model will be a Recurrent Neural Network (RNN) variant, specifically a Long Short-Term Memory (LSTM) network, due to its proven ability to capture long-term dependencies and non-linear relationships inherent in commodity price movements. Inputs to the LSTM will include the historical DJCI values, along with leading economic indicators such as the Purchasing Managers' Index (PMI), consumer price index (CPI), producer price index (PPI), and industrial production data. Furthermore, we will incorporate data on global demand and supply factors for key commodities within the DJCI, like crude oil, metals, and agricultural products.
Feature engineering will be crucial for optimizing model performance. This includes creating lagged variables of the DJCI and economic indicators to account for delayed impacts. We will also explore feature combinations and transformations, such as calculating moving averages, exponential smoothing, and creating ratios between different economic variables to reveal deeper insights. Prior to model training, we will employ data preprocessing techniques, including data cleaning, handling missing values, and standardizing all input variables. The model will be trained using a time-series cross-validation approach to assess the model's performance on unseen data and prevent overfitting. The performance will be assessed by using Mean Absolute Error (MAE), Mean Squared Error (MSE), and R-squared metrics on a holdout set.
To enhance the model's reliability and provide valuable insights, we will integrate economic interpretation into the machine learning predictions. This will involve analyzing the weights and biases learned by the LSTM to understand the influence of different input features on the DJCI forecasts. Scenario analysis will be performed by changing the values of input economic indicators to investigate how they may change DJCI index. Furthermore, we will constantly monitor the model's performance and retrain it regularly, incorporating new data and adapting to evolving market conditions. The model's output will be presented in a user-friendly dashboard allowing financial analysts to easily visualize the DJCI forecasts, key influencing factors, and confidence intervals. We anticipate that this sophisticated model will provide accurate and timely forecasts for the DJCI, enabling informed decision-making for investors and stakeholders.
ML Model Testing
n:Time series to forecast
p:Price signals of DJ Commodity index
j:Nash equilibria (Neural Network)
k:Dominated move of DJ Commodity index holders
a:Best response for DJ Commodity target price
For further technical information as per how our model work we invite you to visit the article below:
How do KappaSignal algorithms actually work?
DJ Commodity Index Forecast Strategic Interaction Table
Strategic Interaction Table Legend:
X axis: *Likelihood% (The higher the percentage value, the more likely the event will occur.)
Y axis: *Potential Impact% (The higher the percentage value, the more likely the price will deviate.)
Z axis (Grey to Black): *Technical Analysis%
DJ Commodity Index: Financial Outlook and Forecast
The DJ Commodity Index, a widely-regarded benchmark for the performance of a diversified basket of commodity futures contracts, currently presents a complex and evolving financial outlook. Several key factors are shaping its trajectory. Primarily, global economic growth projections are pivotal. Strong economic expansion, particularly in emerging markets, typically fuels increased demand for raw materials, leading to higher commodity prices and potentially boosting the index. Conversely, economic slowdowns or recessions, particularly in major consumer nations like China, can dampen demand, exerting downward pressure. Furthermore, the index's performance is significantly impacted by geopolitical events. Conflicts, trade disputes, and political instability can disrupt supply chains, causing price volatility and influencing the index's composition and overall value. Investor sentiment, influenced by factors such as inflation expectations, currency fluctuations, and interest rate policies, also plays a crucial role, impacting the flow of investment capital into and out of commodity markets. The overall supply and demand dynamics for individual commodities within the index, including energy, metals, and agricultural products, contribute to the index's performance as well.
Several critical elements are expected to have substantial impacts on the DJ Commodity Index's forecast in the near to medium term. Inflation rates globally, and the responses from central banks, are expected to create potential volatility. Higher inflation could support commodity prices as a hedge against rising costs. However, aggressive interest rate hikes, designed to curb inflation, might simultaneously slow economic growth, which could counterbalance this positive effect. Furthermore, the energy transition presents a significant and perhaps disruptive influence. Increasing demand for commodities needed for renewable energy infrastructure (e.g., lithium, copper) is expected to provide a tailwind, while potentially reducing the long-term demand for traditional fossil fuels, with an impact on commodities like oil and natural gas. Technological advancements, such as those in energy efficiency and resource extraction, will continue to impact both supply and demand. The evolution of these dynamics requires an investor to monitor these advancements and adapt to changing trends. In addition, climate change will be a consideration, influencing agricultural production through factors like droughts and floods, thus affecting the index's agricultural components.
Considering the prevailing conditions, the DJ Commodity Index is expected to exhibit moderate volatility over the forecast period. Increased supply-side constraints for particular commodities, such as critical minerals, could trigger periods of rapid price escalation. However, macroeconomic factors, such as potentially slowing global growth and increased interest rates, may limit overall index gains. Some commodities are predicted to face structural demand challenges due to the energy transition, potentially lowering their contribution to the overall index performance. Geopolitical uncertainties and disruptions could exacerbate volatility, particularly in energy and agricultural commodities. The impact of government policies, including trade policies, resource regulations, and sustainability initiatives, could also affect the index components significantly. The overall index movement will also be determined by the interactions of supply and demand for each of its components, which fluctuate in response to global trends. The forecast indicates a period of considerable uncertainty, with the index's trajectory largely dependent on the interplay of these dynamic influences.
In conclusion, the DJ Commodity Index's financial outlook is predicted to be moderately positive, with an overall forecast for modest gains over the next year. This prediction is predicated on the continued economic growth in developing economies alongside strategic diversification into sustainable industries. However, significant risks exist. A global economic slowdown, intensified geopolitical instability, or a faster-than-anticipated transition to sustainable energy could negatively impact the index. Conversely, unexpected supply disruptions or greater-than-expected demand, especially for critical minerals and green energy resources, could generate unexpected positive returns. Moreover, unforeseen policy changes, such as commodity export restrictions, could disrupt supply chains, impacting price volatility. Investors should therefore monitor the key factors and take actions that reflect their assessment of the risks and opportunities associated with each commodity group within the index and be prepared to adjust their strategies to reflect these developments.
Rating | Short-Term | Long-Term Senior |
---|---|---|
Outlook | Ba3 | B1 |
Income Statement | Caa2 | B3 |
Balance Sheet | B1 | B2 |
Leverage Ratios | Baa2 | Ba3 |
Cash Flow | Caa2 | C |
Rates of Return and Profitability | Baa2 | Baa2 |
*An aggregate rating for an index summarizes the overall sentiment towards the companies it includes. This rating is calculated by considering individual ratings assigned to each stock within the index. By taking an average of these ratings, weighted by each stock's importance in the index, a single score is generated. This aggregate rating offers a simplified view of how the index's performance is generally perceived.
How does neural network examine financial reports and understand financial state of the company?
References
- Zubizarreta JR. 2015. Stable weights that balance covariates for estimation with incomplete outcome data. J. Am. Stat. Assoc. 110:910–22
- Tibshirani R, Hastie T. 1987. Local likelihood estimation. J. Am. Stat. Assoc. 82:559–67
- E. Altman, K. Avrachenkov, and R. N ́u ̃nez-Queija. Perturbation analysis for denumerable Markov chains with application to queueing models. Advances in Applied Probability, pages 839–853, 2004
- Bai J, Ng S. 2002. Determining the number of factors in approximate factor models. Econometrica 70:191–221
- Vapnik V. 2013. The Nature of Statistical Learning Theory. Berlin: Springer
- L. Prashanth and M. Ghavamzadeh. Actor-critic algorithms for risk-sensitive MDPs. In Proceedings of Advances in Neural Information Processing Systems 26, pages 252–260, 2013.
- M. J. Hausknecht. Cooperation and Communication in Multiagent Deep Reinforcement Learning. PhD thesis, The University of Texas at Austin, 2016