AUC Score :
Short-term Tactic1 :
Dominant Strategy :
Time series to forecast n:
ML Model Testing : Modular Neural Network (CNN Layer)
Hypothesis Testing : Lasso Regression
Surveillance : Major exchange and OTC
1Short-term revised.
2Time series is updated based on short-term trends.
Key Points
The DJ Commodity Energy Index is anticipated to experience moderate volatility. Global economic growth, particularly in emerging markets, will exert upward pressure on energy demand, potentially supporting price appreciation. Simultaneously, geopolitical instability in key producing regions and supply chain disruptions could trigger price spikes. However, the continued adoption of renewable energy sources and increased energy efficiency measures pose a long-term risk to sustained price increases, especially as these alternatives become more cost-competitive.About DJ Commodity Energy Index
The Dow Jones Commodity Energy Index (DJCI Energy) is a benchmark designed to track the performance of the energy sector within the broader commodity market. It specifically focuses on a basket of energy-related futures contracts, reflecting the price movements of key commodities like crude oil, natural gas, heating oil, and gasoline. The index's construction employs a weighted methodology, where the allocation to each commodity is determined based on liquidity and production data. This structure ensures that the DJCI Energy effectively represents the market dynamics of the energy sector, providing investors with a valuable tool for assessing and monitoring the performance of energy commodities.
As a subset of the broader DJCI, the Energy index serves as a critical instrument for market analysis and investment decisions within the energy space. Its methodology and constituent weights are reviewed and rebalanced periodically to ensure that it remains representative of the evolving commodity landscape. Financial institutions and investment professionals often use the DJCI Energy to create investment products, benchmark energy sector performance, and gauge overall market sentiment. Investors frequently refer to it for insights into the global energy market and as a potential hedge against inflation or a component of diversified portfolios.
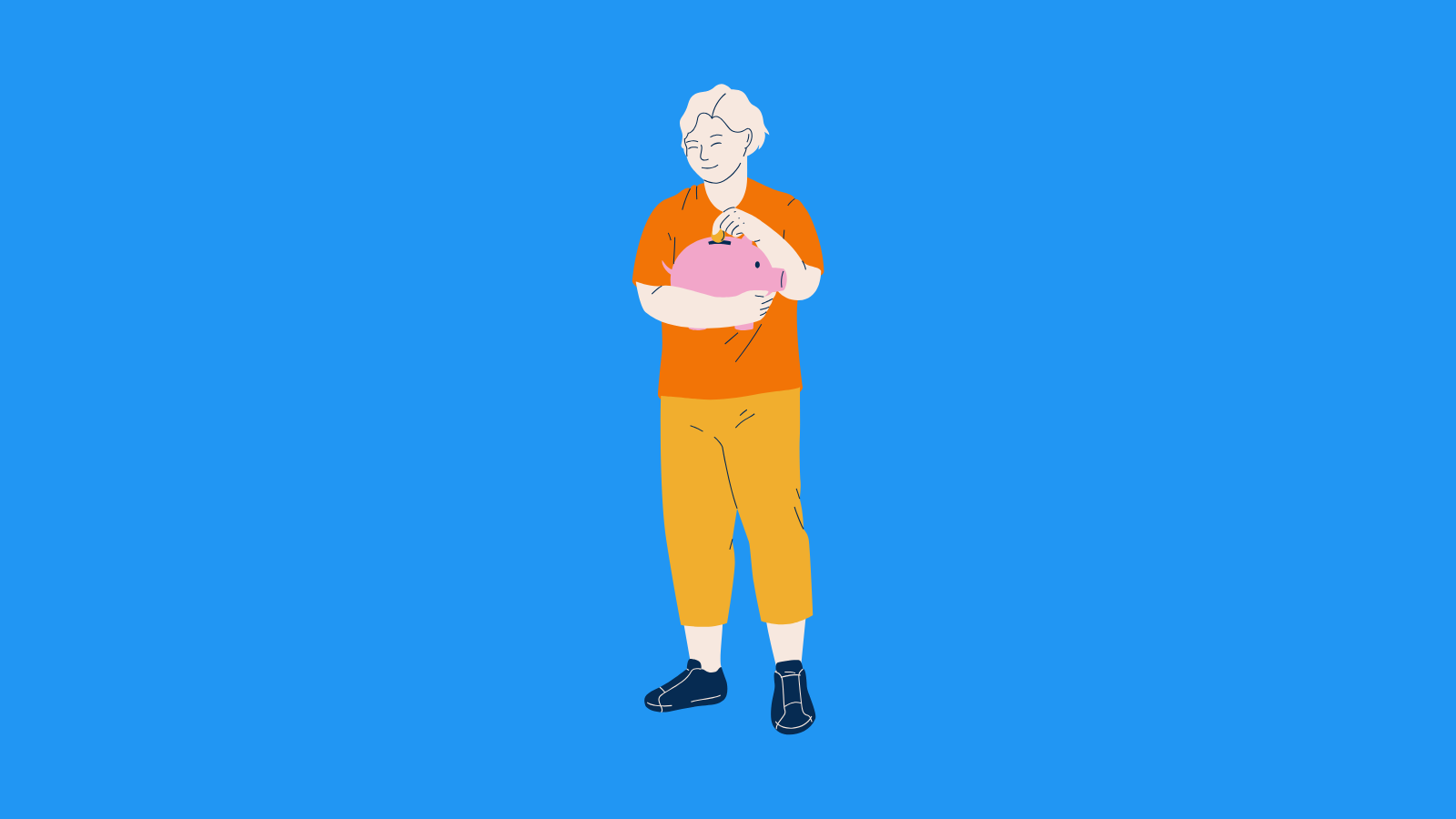
DJ Commodity Energy Index Forecasting Model
Our team of data scientists and economists has developed a robust machine learning model for forecasting the DJ Commodity Energy Index. The core of our model utilizes a time series analysis approach, leveraging historical price data, trading volume, and volatility measures as primary inputs. We integrate economic indicators, including global GDP growth rates, inflation rates, and oil supply and demand dynamics. These economic variables provide crucial contextual information that can influence the price of energy commodities. Feature engineering plays a significant role, with the creation of lagged variables, moving averages, and technical indicators to capture historical trends, seasonality, and short-term fluctuations. We implement a comprehensive data cleaning and preprocessing pipeline to handle missing values and outliers, ensuring the data's integrity and the model's reliability. We have employed several machine learning algorithms, like Recurrent Neural Networks (RNNs), particularly Long Short-Term Memory (LSTM) networks, owing to their capability to capture temporal dependencies. These models are trained on a substantial dataset, using a backtesting approach and cross-validation strategies to evaluate the model's performance and prevent overfitting.
The model's architecture includes multiple layers of LSTM cells, followed by fully connected layers and an output layer to predict future values of the DJ Commodity Energy Index. Model training incorporates techniques, such as dropout and regularization, to enhance generalization. A crucial aspect of our forecasting process is incorporating macroeconomic factors as external variables to the LSTM network. This includes global energy consumption, geopolitical events, and production forecasts. Our model also considers seasonal patterns inherent in energy markets and incorporates this knowledge in the modeling process. We constantly monitor the performance of our model, and we update the model periodically with the latest data and recalibrate its parameters based on changes in the market conditions, using a rolling window approach.
The final model provides both point forecasts and, importantly, confidence intervals, which give us a measure of uncertainty associated with the prediction. Model output is continuously validated against actual market movements, comparing forecasted values with observed values and analyzing residual errors to ensure that the model's predictions align with reality. We evaluate the model's predictive accuracy using metrics such as the Mean Absolute Error (MAE), Mean Squared Error (MSE), and Root Mean Squared Error (RMSE), alongside other domain-specific performance measures. The resulting forecasts are valuable for investment decisions, risk management, and strategic planning, with the caveats that these are forecasts and are not guaranteed to be correct due to inherent market complexities.
ML Model Testing
n:Time series to forecast
p:Price signals of DJ Commodity Energy index
j:Nash equilibria (Neural Network)
k:Dominated move of DJ Commodity Energy index holders
a:Best response for DJ Commodity Energy target price
For further technical information as per how our model work we invite you to visit the article below:
How do KappaSignal algorithms actually work?
DJ Commodity Energy Index Forecast Strategic Interaction Table
Strategic Interaction Table Legend:
X axis: *Likelihood% (The higher the percentage value, the more likely the event will occur.)
Y axis: *Potential Impact% (The higher the percentage value, the more likely the price will deviate.)
Z axis (Grey to Black): *Technical Analysis%
DJ Commodity Energy Index: Outlook and Forecast
The Dow Jones Commodity Energy Index, a key benchmark for the energy sector within the broader commodity market, presents a nuanced outlook shaped by a confluence of global factors. The primary drivers of the index's performance are the price movements of its constituent commodities, principally crude oil and natural gas. **Currently, these commodities are influenced by geopolitical tensions, especially in regions critical to energy supply**. Disruptions to production, transportation, or refining, stemming from conflicts or instability, can trigger significant price spikes. Simultaneously, the index's fortunes are intrinsically linked to the pace of global economic growth. **Stronger economic activity typically fuels higher energy demand**, while slower growth can dampen it. Furthermore, governmental policies, particularly those focused on climate change and the transition to renewable energy sources, exert considerable influence. Initiatives promoting renewable energy can depress the long-term demand for fossil fuels, potentially impacting the index negatively, although the pace of this transition varies across nations. The supply-demand balance is another critical factor: this balance can be affected by OPEC production decisions, the growth of shale production, and capacity utilization rates.
Looking at the factors, several specific trends are likely to affect the DJ Commodity Energy Index. **Supply-side dynamics are playing a crucial role**. OPEC's production quotas, and the adherence of member states to these quotas, will influence global oil supply. Any deviation from agreed-upon output levels could lead to supply shocks. Simultaneously, growth in US shale production has added more production and this affects the market. On the demand side, the economic health of major consuming nations, such as China, India, and the United States, is crucial. Stronger growth in these economies will likely support demand for energy commodities. **Environmental regulations and the push for cleaner energy sources present a headwind**. However, this is balanced by the continued reliance on fossil fuels for various sectors, including transportation and industrial production. Furthermore, the influence of global inventories should be considered, and these inventories influence market sentiment, influencing prices. For the long term, the trajectory of the energy transition will affect the demand.
Analyzing both near-term and long-term perspectives, a mixed outlook appears most probable for the DJ Commodity Energy Index. In the short term, the index may experience volatility. **Geopolitical events and sudden shifts in production capabilities can cause rapid price swings**. During periods of increased risk, the index may rise, while economic downturns could trigger declines. Over the medium term, a slower but more stable growth can be expected. Investment in new supply sources and the rising of economies could influence the stability of the index, but it may be offset by increased efficiency in energy use and developments of renewable energy. In the long term, the shift to renewable energy sources may lead to a lower long-term demand. This requires adjustment to the producers to take it in account.
Prediction and Risks: The DJ Commodity Energy Index is predicted to experience **moderate growth over the medium term, with greater volatility in the short term**. This forecast is based on a balance of conflicting trends: geopolitical instability, the economic outlook in major consuming economies, and the ongoing transition to cleaner energy sources. The most significant risks to this prediction include **escalation of geopolitical conflicts disrupting energy supply chains, and a faster-than-anticipated shift away from fossil fuels due to technological advancements or policy interventions**. Also, a global economic recession could significantly reduce energy demand and drag the index down. **A larger-than-expected increase in the production of low-cost energy supplies or a sudden technological breakthrough in energy storage or renewable energy generation could also depress the index**. However, if global economic growth strengthens, or if the shift to renewable energy is more gradual, the index has the possibility of exceeding its expected growth.
Rating | Short-Term | Long-Term Senior |
---|---|---|
Outlook | B3 | B1 |
Income Statement | Baa2 | Caa2 |
Balance Sheet | C | Caa2 |
Leverage Ratios | C | Baa2 |
Cash Flow | C | Baa2 |
Rates of Return and Profitability | B1 | C |
*An aggregate rating for an index summarizes the overall sentiment towards the companies it includes. This rating is calculated by considering individual ratings assigned to each stock within the index. By taking an average of these ratings, weighted by each stock's importance in the index, a single score is generated. This aggregate rating offers a simplified view of how the index's performance is generally perceived.
How does neural network examine financial reports and understand financial state of the company?
References
- J. Hu and M. P. Wellman. Nash q-learning for general-sum stochastic games. Journal of Machine Learning Research, 4:1039–1069, 2003.
- N. B ̈auerle and J. Ott. Markov decision processes with average-value-at-risk criteria. Mathematical Methods of Operations Research, 74(3):361–379, 2011
- Matzkin RL. 2007. Nonparametric identification. In Handbook of Econometrics, Vol. 6B, ed. J Heckman, E Learner, pp. 5307–68. Amsterdam: Elsevier
- Athey S, Mobius MM, Pál J. 2017c. The impact of aggregators on internet news consumption. Unpublished manuscript, Grad. School Bus., Stanford Univ., Stanford, CA
- Barkan O. 2016. Bayesian neural word embedding. arXiv:1603.06571 [math.ST]
- K. Boda and J. Filar. Time consistent dynamic risk measures. Mathematical Methods of Operations Research, 63(1):169–186, 2006
- Rumelhart DE, Hinton GE, Williams RJ. 1986. Learning representations by back-propagating errors. Nature 323:533–36