AUC Score :
Short-term Tactic1 :
Dominant Strategy :
Time series to forecast n:
ML Model Testing : Transductive Learning (ML)
Hypothesis Testing : Chi-Square
Surveillance : Major exchange and OTC
1Short-term revised.
2Time series is updated based on short-term trends.
Key Points
The TR/CC CRB Cotton index is projected to exhibit moderate volatility. Global supply chain disruptions and fluctuating weather patterns in key cotton-producing regions will likely contribute to price instability. Demand is anticipated to remain steady, supported by the textile industry, but potential economic slowdowns could temper consumption growth. A significant risk factor is the impact of geopolitical tensions on trade routes and commodity prices, potentially leading to supply constraints and price spikes. Conversely, technological advancements in cotton farming and increased yields could stabilize prices or exert downward pressure.About TR/CC CRB Cotton Index
The TR/CC CRB Cotton Index is a commodity index that tracks the price fluctuations of cotton futures contracts. It serves as a benchmark for cotton prices, providing insights into market trends and volatility within the cotton sector. This index is calculated and maintained by Thompson Reuters and the Commodity Research Bureau (CRB). The index is widely used by investors, traders, and analysts to monitor and analyze the performance of the cotton market. Furthermore, it helps in understanding the factors influencing cotton prices, such as weather patterns, global demand, and production levels.
The TR/CC CRB Cotton Index can be utilized as a tool for various purposes. It helps in assessing market risk, formulating trading strategies, and evaluating the performance of cotton-related investments. The index is based on the nearby futures contract. Market participants use this information to hedge against price risks. It offers a standardized way to evaluate the overall market direction and the relative value of cotton in comparison to other commodities. By tracking cotton's performance, the index plays a vital role in price discovery and overall market efficiency in the cotton trade.
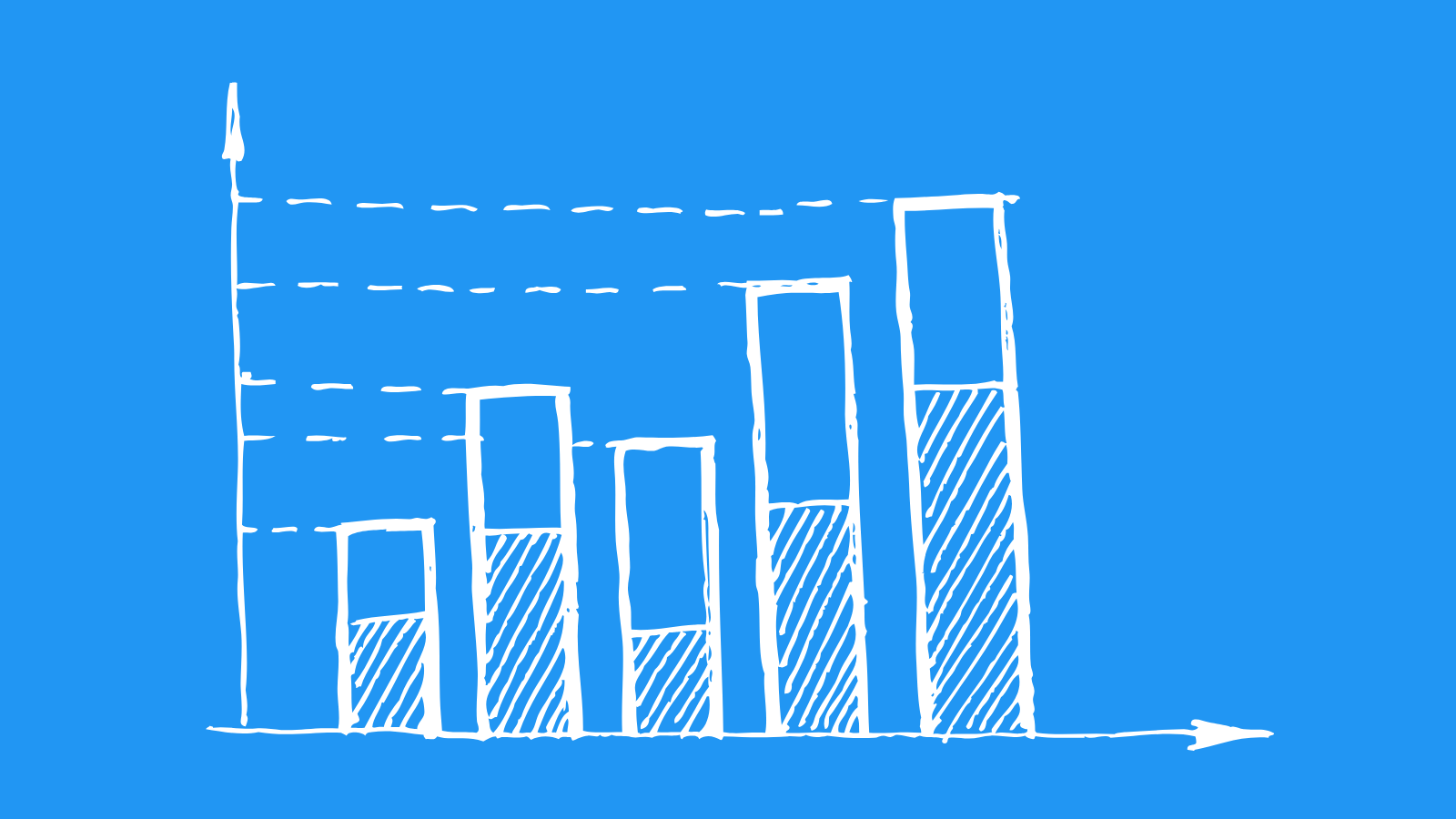
TR/CC CRB Cotton Index Forecasting Model
Our team of data scientists and economists has developed a comprehensive machine learning model designed to forecast the TR/CC CRB Cotton index. The model employs a multi-faceted approach, leveraging a combination of time series analysis, economic indicators, and market sentiment data. The core of our model utilizes a recurrent neural network (RNN), specifically a Long Short-Term Memory (LSTM) network, which is well-suited for capturing complex temporal dependencies inherent in commodity prices. Historical TR/CC CRB Cotton index data, encompassing price fluctuations over several years, forms the primary training dataset. Additionally, we incorporate macroeconomic variables known to influence cotton prices, such as global GDP growth, exchange rates (USD/other currencies), and inflation rates. Finally, we integrate market-specific data, including supply and demand dynamics, inventory levels, and futures contract data. This comprehensive approach aims to capture the multifaceted nature of the cotton market and provide accurate forecasts.
Feature engineering plays a critical role in enhancing the model's predictive power. We carefully preprocess the raw data, handling missing values and outliers through imputation techniques and robust statistical methods. Furthermore, we create new features derived from existing data, such as rolling averages and volatility measures, to provide additional insights into market trends. Economic indicators are preprocessed to remove seasonality and trends, ensuring the model focuses on relevant short-term and medium-term impacts. Model training utilizes a carefully curated dataset, split into training, validation, and testing sets, ensuring proper model performance evaluation. We employ techniques like hyperparameter tuning, cross-validation, and regularization to optimize model parameters, prevent overfitting, and enhance generalization capabilities. The validation set enables model optimization while the testing set assesses its performance on unseen data, providing a robust measure of forecast accuracy.
The final model output provides forecasts for the TR/CC CRB Cotton index over various time horizons. The model provides both point estimates (single-value predictions) and probabilistic forecasts that quantify the uncertainty in the predictions. Model performance is continuously monitored using key metrics such as Mean Absolute Error (MAE), Root Mean Squared Error (RMSE), and Mean Absolute Percentage Error (MAPE). The model is regularly updated with fresh data and retrained to maintain its predictive accuracy, especially considering the dynamic nature of the cotton market. The insights gained from this model enable informed decision-making for various stakeholders, including cotton producers, textile manufacturers, and commodity traders. Furthermore, the model can be used to analyze scenario-based simulations and risk assessment.
ML Model Testing
n:Time series to forecast
p:Price signals of TR/CC CRB Cotton index
j:Nash equilibria (Neural Network)
k:Dominated move of TR/CC CRB Cotton index holders
a:Best response for TR/CC CRB Cotton target price
For further technical information as per how our model work we invite you to visit the article below:
How do KappaSignal algorithms actually work?
TR/CC CRB Cotton Index Forecast Strategic Interaction Table
Strategic Interaction Table Legend:
X axis: *Likelihood% (The higher the percentage value, the more likely the event will occur.)
Y axis: *Potential Impact% (The higher the percentage value, the more likely the price will deviate.)
Z axis (Grey to Black): *Technical Analysis%
TR/CC CRB Cotton Index Financial Outlook and Forecast
The TR/CC CRB Cotton Index, a benchmark reflecting the price movements of cotton futures contracts, offers a valuable perspective on the overall health and future trajectory of the global cotton market. This index is a crucial indicator for stakeholders involved in cotton production, processing, and trading, as well as for investors seeking exposure to the agricultural commodity sector. Currently, analysis of various economic indicators and market dynamics suggests a period of moderate growth and stability for the cotton market. Demand is anticipated to remain relatively consistent, driven by underlying consumer preferences for natural fibers and the textile industry's ongoing needs. Supply-side dynamics, including factors such as weather patterns impacting cotton-growing regions, technological advancements in agriculture, and geopolitical events influencing trade flows, will play a pivotal role in shaping the index's performance. Understanding these multifaceted elements is essential for accurately assessing the future financial outlook of the TR/CC CRB Cotton Index.
Several key factors are expected to influence the financial outlook for the TR/CC CRB Cotton Index in the coming periods. First, the continued strength in global textile demand, particularly from emerging markets where consumer spending is projected to grow, is expected to provide a solid foundation for cotton prices. Second, the influence of government agricultural policies and trade agreements, which can significantly impact cotton production and export volumes, remains crucial. Third, fluctuations in currency exchange rates, especially the strength of the U.S. dollar (as cotton is priced in USD), can affect international cotton prices and demand. Finally, the impact of supply-chain disruptions, labor shortages, and rising input costs (such as fertilizers and energy) will also have a bearing on production costs and, in turn, cotton prices. Each of these elements needs diligent monitoring and considered integration into any assessment of the index's forward-looking prospects.
Analyzing these different elements indicates that the TR/CC CRB Cotton Index is likely to experience a period of relative stability, with modest price fluctuations. The global textile industry's steady demand, particularly from growing emerging economies, should provide underlying support. However, the expected continued volatility from factors such as weather events (e.g., droughts, floods) and logistical challenges is likely to limit any substantial gains. Moreover, the current economic conditions globally, marked by varying degrees of inflation and monetary policy tightening, could potentially dampen consumer spending and slow demand growth. Producers and consumers, alike, need to stay informed regarding developments in key cotton-producing regions, such as the United States, China, and India, as crop yields and supply availability are paramount factors. Additionally, geopolitical events and shifts in global trade patterns can introduce uncertainty and influence price dynamics.
Overall, a cautious but generally positive outlook can be projected for the TR/CC CRB Cotton Index. The anticipated continued demand from the textile sector and stable supply, as suggested by current forecasts, points towards a positive trajectory. However, there are associated risks to this prediction. The most significant risk remains the unpredictability of weather patterns, which could lead to substantial crop losses and significant price volatility. Economic downturns in major consumer markets could diminish cotton demand, creating downward pressure on prices. Finally, changing trade regulations and geopolitical tensions could disrupt supply chains and international trade, impacting cotton production and distribution. Constant monitoring of all market factors and risk mitigation strategies will be crucial for those invested or linked to the TR/CC CRB Cotton Index.
Rating | Short-Term | Long-Term Senior |
---|---|---|
Outlook | B1 | B1 |
Income Statement | B3 | C |
Balance Sheet | Ba1 | Baa2 |
Leverage Ratios | Caa2 | Ba3 |
Cash Flow | Caa2 | B2 |
Rates of Return and Profitability | Baa2 | B2 |
*An aggregate rating for an index summarizes the overall sentiment towards the companies it includes. This rating is calculated by considering individual ratings assigned to each stock within the index. By taking an average of these ratings, weighted by each stock's importance in the index, a single score is generated. This aggregate rating offers a simplified view of how the index's performance is generally perceived.
How does neural network examine financial reports and understand financial state of the company?
References
- V. Borkar and R. Jain. Risk-constrained Markov decision processes. IEEE Transaction on Automatic Control, 2014
- Chamberlain G. 2000. Econometrics and decision theory. J. Econom. 95:255–83
- Athey S, Tibshirani J, Wager S. 2016b. Generalized random forests. arXiv:1610.01271 [stat.ME]
- E. van der Pol and F. A. Oliehoek. Coordinated deep reinforcement learners for traffic light control. NIPS Workshop on Learning, Inference and Control of Multi-Agent Systems, 2016.
- E. Collins. Using Markov decision processes to optimize a nonlinear functional of the final distribution, with manufacturing applications. In Stochastic Modelling in Innovative Manufacturing, pages 30–45. Springer, 1997
- Chernozhukov V, Chetverikov D, Demirer M, Duflo E, Hansen C, Newey W. 2017. Double/debiased/ Neyman machine learning of treatment effects. Am. Econ. Rev. 107:261–65
- Dietterich TG. 2000. Ensemble methods in machine learning. In Multiple Classifier Systems: First International Workshop, Cagliari, Italy, June 21–23, pp. 1–15. Berlin: Springer