AUC Score :
Short-term Tactic1 :
Dominant Strategy :
Time series to forecast n:
ML Model Testing : Modular Neural Network (Market Direction Analysis)
Hypothesis Testing : Linear Regression
Surveillance : Major exchange and OTC
1Short-term revised.
2Time series is updated based on short-term trends.
Key Points
The TR/CC CRB Cotton index is anticipated to experience a period of moderate price volatility. The index is likely to show a gradual increase, reflecting steady demand and potentially constrained global supplies. However, this trajectory carries risks, including unfavorable weather conditions impacting cotton yields in key producing regions, shifts in global trade policies, and fluctuations in consumer demand which could lead to price corrections and declines. Furthermore, economic downturns could soften demand and push the index downwards.About TR/CC CRB Cotton Index
The TR/CC CRB Cotton Index serves as a benchmark reflecting the price movements of cotton futures contracts. It's designed to offer a broad measure of the performance of the cotton market, a crucial commodity in the textile industry and global trade. This index is often used by investors and analysts to gauge market sentiment, assess the economic health of the cotton sector, and make informed decisions about investment strategies related to cotton and associated derivatives. It reflects the daily price fluctuations of actively traded cotton futures contracts, providing a readily available reference point for market participants.
The composition and methodology of the TR/CC CRB Cotton Index typically involve selecting a specific futures contract series that represent the benchmark and determining the weighting methodology for each contract within the index. This can involve equal weighting or a system that gives higher weight to contracts with greater trading volume or open interest. Regularly, the index is rebalanced to maintain accuracy as contracts expire and new ones become active. The Index is a tool for market participants to assess the cotton markets.
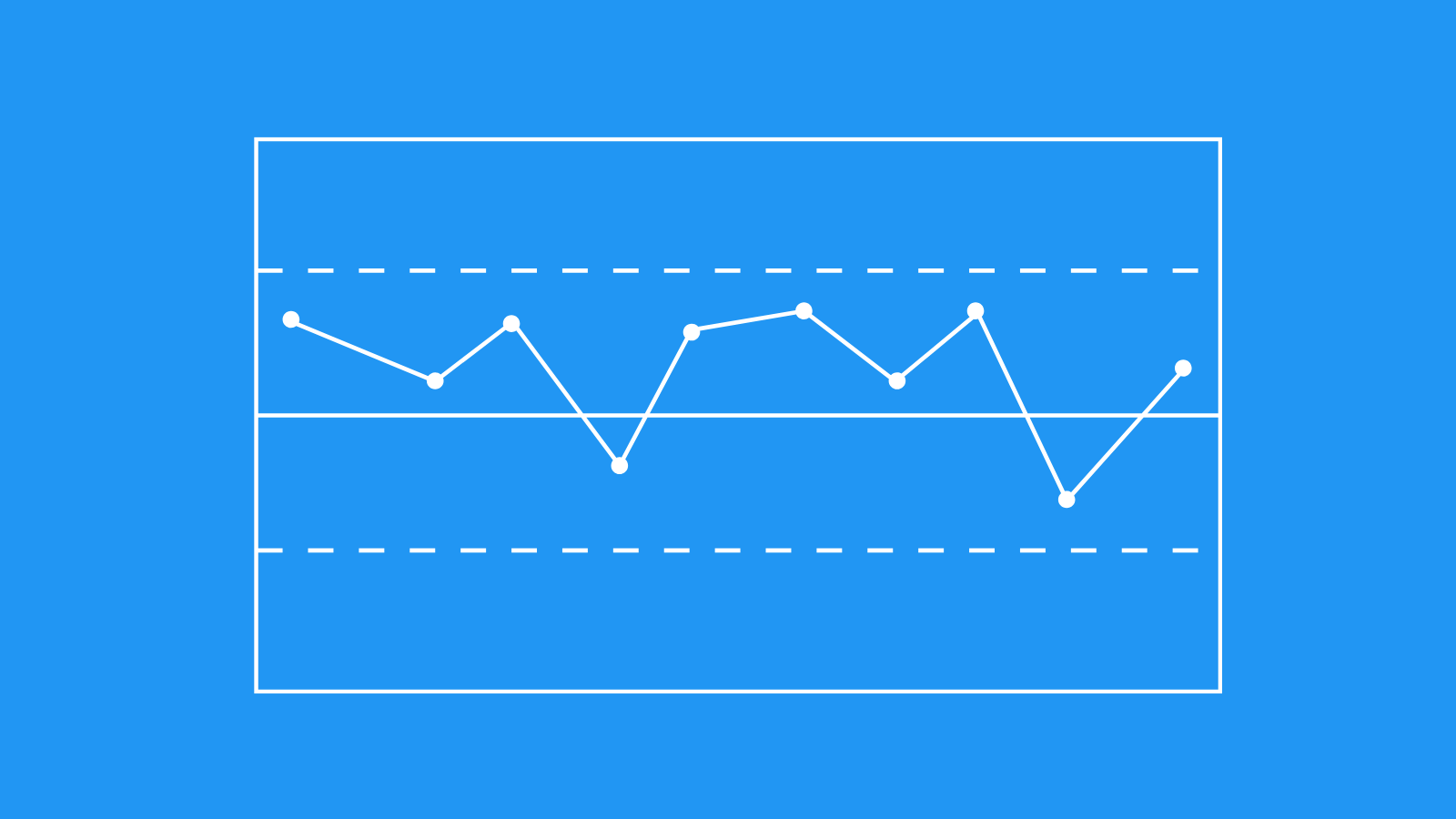
Machine Learning Model for TR/CC CRB Cotton Index Forecast
Our team of data scientists and economists proposes a robust machine learning model for forecasting the TR/CC CRB Cotton Index. The foundation of our model rests on a comprehensive understanding of the intricate factors influencing cotton prices. We will utilize a hybrid approach, integrating both time-series analysis and economic indicator analysis. For the time-series component, we will employ Recurrent Neural Networks (RNNs), specifically Long Short-Term Memory (LSTM) networks, known for their proficiency in capturing temporal dependencies and handling sequential data. We will also incorporate historical price data and trading volume. For the economic indicators, we will consider factors such as global cotton production levels, supply chain disruptions, demand from key textile manufacturing countries, global economic growth (GDP), currency exchange rates, and government policies like subsidies and trade tariffs. These indicators will provide crucial contextual information. We will also take into account of climate conditions like droughts. We believe this method can provide better forecasting capability.
The model development process will involve several key steps. First, data acquisition and preprocessing will be conducted. This includes gathering historical price data, relevant economic indicators, and any available qualitative information. Data cleaning, handling missing values, and feature engineering will be performed to ensure data quality. Subsequently, the dataset will be split into training, validation, and testing sets. We will use the training set to train our model, the validation set for hyperparameter tuning and model selection, and the testing set to evaluate the model's performance on unseen data. Hyperparameter tuning will be performed to optimize the performance of the LSTM networks, adjusting parameters such as the number of layers, the number of neurons per layer, and the learning rate. We will use a combination of techniques such as grid search, random search, and Bayesian optimization. The model's performance will be evaluated using metrics such as Mean Squared Error (MSE), Root Mean Squared Error (RMSE), and Mean Absolute Percentage Error (MAPE).
Finally, model deployment and monitoring are critical. Upon achieving satisfactory performance, the trained model will be deployed for real-time forecasting. We will establish an automated data pipeline to ingest new data and update the model periodically. Continuous monitoring of the model's performance is necessary to identify any degradation in accuracy. Regular model retraining using the latest data will be performed to adapt to evolving market dynamics and incorporate new information. Furthermore, we will conduct a sensitivity analysis to understand the impact of different economic indicators on the forecasts. This will enable us to identify the most critical factors driving cotton price movements, providing actionable insights for risk management and strategic decision-making. The model will be designed to provide probabilistic forecasts, including confidence intervals, to quantify the uncertainty associated with the predictions.
```
ML Model Testing
n:Time series to forecast
p:Price signals of TR/CC CRB Cotton index
j:Nash equilibria (Neural Network)
k:Dominated move of TR/CC CRB Cotton index holders
a:Best response for TR/CC CRB Cotton target price
For further technical information as per how our model work we invite you to visit the article below:
How do KappaSignal algorithms actually work?
TR/CC CRB Cotton Index Forecast Strategic Interaction Table
Strategic Interaction Table Legend:
X axis: *Likelihood% (The higher the percentage value, the more likely the event will occur.)
Y axis: *Potential Impact% (The higher the percentage value, the more likely the price will deviate.)
Z axis (Grey to Black): *Technical Analysis%
TR/CC CRB Cotton Index: Financial Outlook and Forecast
The TR/CC CRB Cotton Index, a benchmark reflecting the price movements of cotton futures contracts, is influenced by a complex interplay of global supply and demand dynamics. Examining the index's financial outlook requires careful consideration of factors such as global cotton production, consumption patterns, and geopolitical events that could impact trade flows. The index's performance is often linked to agricultural policy decisions in major cotton-producing nations like the United States, China, India, and Brazil. Shifts in these policies, including export subsidies, import tariffs, and acreage allocation, can significantly affect the index's trajectory. Additionally, weather patterns, including droughts, floods, and temperature fluctuations in key cotton-growing regions, represent critical supply-side risks. Understanding these elements provides a foundation for assessing the index's potential performance.
Analyzing the financial outlook also necessitates considering consumption trends. Demand from textile industries, especially in emerging markets like Southeast Asia, directly affects cotton prices. Any shifts in consumer preferences towards synthetic fibers or disruptions in global trade, such as port closures or supply chain bottlenecks, can impact demand and, consequently, the index. Furthermore, the index is sensitive to macroeconomic indicators, including economic growth rates, inflation, and currency exchange rates. Strong economic expansion often leads to increased textile demand and higher cotton prices. Conversely, economic slowdowns or currency devaluations in key import markets can restrain demand and pressure the index downwards. Therefore, a comprehensive analysis must incorporate a broad understanding of global economic conditions.
Recent trends indicate a complex environment for the TR/CC CRB Cotton Index. Factors like climate change and water scarcity in cotton-producing regions are exerting persistent pressure on supply. Meanwhile, evolving consumer preferences and the development of alternative materials continue to challenge cotton's market dominance. The ongoing situation in key cotton-producing countries, including political instability and changes to trade policy, adds an extra layer of uncertainty to the index. Inventory levels, both globally and in specific regions, need to be monitored as they influence immediate price movements. Any unexpected supply disruptions, perhaps caused by pest infestations or adverse weather events, would likely drive the index up. Conversely, a surge in production or a weakening of demand would put downward pressure on prices.
Based on current data and analysis, the outlook for the TR/CC CRB Cotton Index is projected to be volatile. While strong demand from emerging markets will offer some support, supply constraints and geopolitical uncertainties pose significant challenges. The forecast suggests a cautious positive trajectory, predicated on the ongoing demand with potential price increases, given adequate weather conditions and the absence of major trade disruptions. Key risks to this prediction include severe weather events in major growing regions, the resurgence of synthetic fiber competition, and policy changes. Any significant economic downturn or changes to trade agreements could also dramatically alter the forecast. Investors must remain vigilant, closely monitor these factors, and consider risk mitigation strategies, such as diversification, to effectively navigate the potential fluctuations of the TR/CC CRB Cotton Index.
Rating | Short-Term | Long-Term Senior |
---|---|---|
Outlook | Baa2 | Ba3 |
Income Statement | Baa2 | Baa2 |
Balance Sheet | Ba3 | C |
Leverage Ratios | Baa2 | B2 |
Cash Flow | B3 | Baa2 |
Rates of Return and Profitability | Baa2 | Baa2 |
*An aggregate rating for an index summarizes the overall sentiment towards the companies it includes. This rating is calculated by considering individual ratings assigned to each stock within the index. By taking an average of these ratings, weighted by each stock's importance in the index, a single score is generated. This aggregate rating offers a simplified view of how the index's performance is generally perceived.
How does neural network examine financial reports and understand financial state of the company?
References
- Barkan O. 2016. Bayesian neural word embedding. arXiv:1603.06571 [math.ST]
- Li L, Chu W, Langford J, Moon T, Wang X. 2012. An unbiased offline evaluation of contextual bandit algo- rithms with generalized linear models. In Proceedings of 4th ACM International Conference on Web Search and Data Mining, pp. 297–306. New York: ACM
- Mikolov T, Chen K, Corrado GS, Dean J. 2013a. Efficient estimation of word representations in vector space. arXiv:1301.3781 [cs.CL]
- G. Shani, R. Brafman, and D. Heckerman. An MDP-based recommender system. In Proceedings of the Eigh- teenth conference on Uncertainty in artificial intelligence, pages 453–460. Morgan Kaufmann Publishers Inc., 2002
- Tibshirani R, Hastie T. 1987. Local likelihood estimation. J. Am. Stat. Assoc. 82:559–67
- Hastie T, Tibshirani R, Friedman J. 2009. The Elements of Statistical Learning. Berlin: Springer
- M. Colby, T. Duchow-Pressley, J. J. Chung, and K. Tumer. Local approximation of difference evaluation functions. In Proceedings of the Fifteenth International Joint Conference on Autonomous Agents and Multiagent Systems, Singapore, May 2016