AUC Score :
Short-term Tactic1 :
Dominant Strategy :
Time series to forecast n:
ML Model Testing : Modular Neural Network (Market Direction Analysis)
Hypothesis Testing : Sign Test
Surveillance : Major exchange and OTC
1Short-term revised.
2Time series is updated based on short-term trends.
Key Points
TR/CC CRB Coffee index is projected to experience volatile trading conditions. The index's price is anticipated to initially rise, driven by concerns regarding unfavorable weather patterns in key coffee-producing regions, possibly leading to a supply deficit. However, this upward trend may be short-lived as increased production from other regions could mitigate the supply shortages. Downward pressure could then take effect, potentially causing price correction. The main risk associated with this outlook includes unforeseen extreme weather events that could severely damage coffee crops, causing a substantial price increase. Geopolitical instability in key coffee-producing countries poses another risk, potentially disrupting trade and amplifying price volatility. On the other hand, a significant increase in global demand or positive economic data could also drive up prices, and therefore the inverse is also possible.About TR/CC CRB Coffee Index
The Thomson Reuters/CoreCommodity CRB (TR/CC CRB) index serves as a widely recognized benchmark for the overall commodity market. It is a futures-based index, meaning its value is derived from the prices of futures contracts on various commodities. The index aims to provide a comprehensive measure of commodity price movements, reflecting the aggregate performance of diverse raw materials that are vital to the global economy. It's a valuable tool for investors, analysts, and market participants looking to understand and track broader trends within the commodity sector.
The TR/CC CRB index is comprised of a basket of commodities, including energy products, precious metals, industrial metals, agricultural goods, and livestock. The weighting of each commodity within the index is determined by factors like trading volume and liquidity, ensuring a representation of the most actively traded and economically significant commodities. Rebalancing of the index happens regularly to maintain its relevance and accurately portray prevailing market conditions within the commodity markets, making it a key indicator for inflation and economic activity analysis.
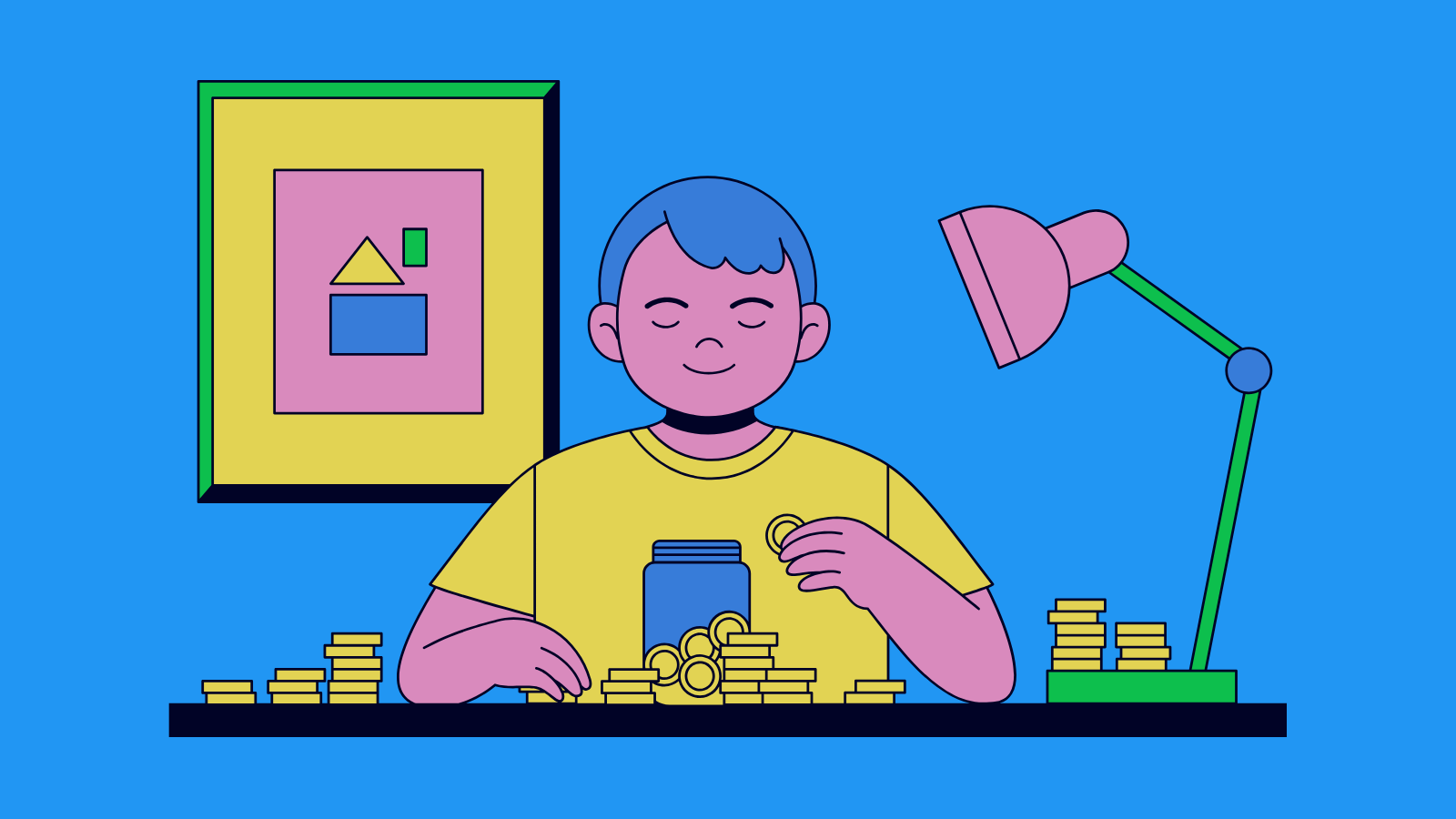
Machine Learning Model for TR/CC CRB Coffee Index Forecast
Our team of data scientists and economists has developed a machine learning model designed to forecast the TR/CC CRB Coffee index. The model utilizes a comprehensive dataset encompassing various economic and market indicators. These include but are not limited to: historical coffee futures prices, global production volumes (Brazil, Vietnam, Colombia, etc.), inventory levels across major exchanges, relevant weather patterns impacting coffee-growing regions (e.g., drought conditions), exchange rates (USD, BRL, VND, etc.), inflation rates within key coffee-producing and consuming nations, and global economic growth indicators such as Purchasing Managers' Index (PMI) and GDP growth. The data is preprocessed to handle missing values, outliers, and ensure data consistency. Feature engineering is performed to create relevant predictors, such as moving averages of historical prices and momentum indicators. This forms the foundation for our forecasting approach.
The core of our model employs an ensemble approach leveraging several machine learning algorithms. We've opted for a combination of time-series forecasting methods, specifically LSTM (Long Short-Term Memory) networks, alongside Gradient Boosting Machines (GBM) and Support Vector Regression (SVR). The choice of LSTM networks is particularly relevant due to their ability to capture long-term dependencies inherent in commodity price fluctuations. GBM and SVR models are incorporated to further refine the model's accuracy. Model training is conducted on a split dataset to ensure the unseen data is available to test the model, including hyperparameter tuning using techniques like cross-validation. The final forecast is generated by aggregating the individual model outputs, with weights assigned based on their past performance.
The model's output provides a forecasted value for the TR/CC CRB Coffee index for a predefined time horizon (e.g., monthly or quarterly). We evaluate the model's performance using standard metrics, namely Mean Absolute Error (MAE), Root Mean Squared Error (RMSE), and R-squared. The model is regularly updated and retrained with fresh data to maintain its accuracy. This enables continuous learning and adaptation to evolving market dynamics. In conclusion, our machine learning model offers a robust and data-driven approach to forecasting the TR/CC CRB Coffee index, providing valuable insights for market participants, including commodity traders and investors.
ML Model Testing
n:Time series to forecast
p:Price signals of TR/CC CRB Coffee index
j:Nash equilibria (Neural Network)
k:Dominated move of TR/CC CRB Coffee index holders
a:Best response for TR/CC CRB Coffee target price
For further technical information as per how our model work we invite you to visit the article below:
How do KappaSignal algorithms actually work?
TR/CC CRB Coffee Index Forecast Strategic Interaction Table
Strategic Interaction Table Legend:
X axis: *Likelihood% (The higher the percentage value, the more likely the event will occur.)
Y axis: *Potential Impact% (The higher the percentage value, the more likely the price will deviate.)
Z axis (Grey to Black): *Technical Analysis%
TR/CC CRB Coffee Index: Financial Outlook and Forecast
The TR/CC CRB Coffee Index, reflecting the price fluctuations of arabica and robusta coffee futures contracts, presents a complex financial outlook. The index's performance is heavily influenced by global supply and demand dynamics, weather patterns in major coffee-producing regions, and geopolitical events. Currently, the market faces several key drivers. Supply-side factors include the impact of La NiƱa on coffee production in Brazil, the world's largest coffee exporter, potentially leading to reduced yields and higher prices. Weather conditions in Vietnam, a significant robusta producer, also play a crucial role. Furthermore, disruptions in shipping and logistics, caused by international events or climate changes, can increase volatility. On the demand side, global consumption trends and economic growth in emerging markets, particularly China and India, are vital indicators. These countries' rising consumption habits could significantly impact global coffee demand. Currency fluctuations also play a pivotal role, as a stronger US dollar makes coffee more expensive for importers, while a weaker dollar could boost demand.
Analysing these complex factors, the index's near-term performance is likely to be subject to significant price volatility. The expectation is that the coffee index will be in the state of price fluctuation. Increased volatility is expected due to several simultaneous forces: the impacts of climate change and weather patterns in producing countries. For example, if there are issues with supply side factors such as drought or floods then there would be the potential for price inflation. The impact of any adverse weather event in Brazil and Vietnam would have a direct impact on coffee prices. Similarly, any change in consumption patterns or supply chain disruptions will cause variations in the index. Furthermore, the index is heavily influenced by the actions of institutional investors and commodity traders, who can exacerbate price swings based on their risk appetite and market speculation.
Mid-term prospects for the TR/CC CRB Coffee Index appear less certain, as long-term factors start playing a role in the market. The industry is also susceptible to the impacts of new sustainability initiatives. Growing consumer awareness of environmental and social concerns could change demand patterns and potentially shift the focus towards certified and sustainably produced coffee, influencing the price spread between different types of coffee. In addition, the emergence of innovative coffee production technologies, such as vertical farming and laboratory-grown coffee, could have a significant impact on supply and potentially reduce prices in the long term. The economic outlook of major coffee-consuming countries will also play a role in medium term. For example, any slowing economic growth may affect the demand for coffee, influencing the prices of the coffee index.
Overall, the outlook for the TR/CC CRB Coffee Index is cautiously optimistic, with periods of volatility anticipated. The prediction is that the index will experience fluctuations as the market navigates through both supply and demand side factors. However, there are risks that must be kept in mind. The most significant risk is unexpected weather events in major coffee-producing regions, which could significantly reduce production and drive up prices. Another risk is a global economic recession, which could decrease consumer demand. In addition, there is a risk of changes in the supply chain; for example, delays could impact the prices. It is recommended to closely monitor market developments, manage risks effectively, and adapt strategies based on the evolving dynamics of the coffee market.
Rating | Short-Term | Long-Term Senior |
---|---|---|
Outlook | B2 | Ba2 |
Income Statement | C | B1 |
Balance Sheet | Baa2 | Baa2 |
Leverage Ratios | Baa2 | Ba2 |
Cash Flow | Caa2 | Caa2 |
Rates of Return and Profitability | C | Baa2 |
*An aggregate rating for an index summarizes the overall sentiment towards the companies it includes. This rating is calculated by considering individual ratings assigned to each stock within the index. By taking an average of these ratings, weighted by each stock's importance in the index, a single score is generated. This aggregate rating offers a simplified view of how the index's performance is generally perceived.
How does neural network examine financial reports and understand financial state of the company?
References
- Chamberlain G. 2000. Econometrics and decision theory. J. Econom. 95:255–83
- Jorgenson, D.W., Weitzman, M.L., ZXhang, Y.X., Haxo, Y.M. and Mat, Y.X., 2023. S&P 500: Is the Bull Market Ready to Run Out of Steam?. AC Investment Research Journal, 220(44).
- F. A. Oliehoek, M. T. J. Spaan, and N. A. Vlassis. Optimal and approximate q-value functions for decentralized pomdps. J. Artif. Intell. Res. (JAIR), 32:289–353, 2008
- S. Bhatnagar, H. Prasad, and L. Prashanth. Stochastic recursive algorithms for optimization, volume 434. Springer, 2013
- L. Busoniu, R. Babuska, and B. D. Schutter. A comprehensive survey of multiagent reinforcement learning. IEEE Transactions of Systems, Man, and Cybernetics Part C: Applications and Reviews, 38(2), 2008.
- Abadie A, Diamond A, Hainmueller J. 2015. Comparative politics and the synthetic control method. Am. J. Political Sci. 59:495–510
- Chernozhukov V, Chetverikov D, Demirer M, Duflo E, Hansen C, et al. 2016a. Double machine learning for treatment and causal parameters. Tech. Rep., Cent. Microdata Methods Pract., Inst. Fiscal Stud., London