AUC Score :
Short-term Tactic1 :
Dominant Strategy :
Time series to forecast n:
ML Model Testing : Multi-Instance Learning (ML)
Hypothesis Testing : Wilcoxon Sign-Rank Test
Surveillance : Major exchange and OTC
1Short-term revised.
2Time series is updated based on short-term trends.
Key Points
Cardiff Oncology is projected to experience significant volatility due to its reliance on single-asset clinical trials targeting cancer. Success in its lead program, onvansertib, could lead to substantial share price appreciation, especially if it receives regulatory approval for its focus indications. However, there's a considerable risk of failure in clinical trials, which would likely result in a drastic decline in value. The company's financial health is another area of concern. Any delays in fundraising or adverse clinical results could impair its ability to fund further research and operations. Furthermore, the competitive environment within oncology presents challenges, with other biopharmaceutical companies also seeking to develop effective cancer treatments.About Cardiff Oncology
Cardiff Oncology (CRDF) is a clinical-stage biotechnology company focused on developing and commercializing novel therapies for the treatment of cancer. The company's primary focus is on onvansertib, a Polo-like Kinase 1 (PLK1) inhibitor. Onvansertib is being investigated in multiple clinical trials across various solid tumor types, including metastatic pancreatic cancer and KRAS-mutated colorectal cancer. Cardiff Oncology aims to improve patient outcomes through targeted therapies that address the underlying mechanisms of cancer growth and progression, especially in indications with limited treatment options.
The company's research and development efforts are centered around the potential of onvansertib to enhance the efficacy of existing chemotherapies and targeted therapies. Cardiff Oncology has received Fast Track designation from the FDA for onvansertib in combination with FOLFIRI and bevacizumab for second-line treatment of metastatic colorectal cancer. They are working to understand the potential of onvansertib across different cancer types and treatment combinations, with a goal of addressing unmet needs in oncology.
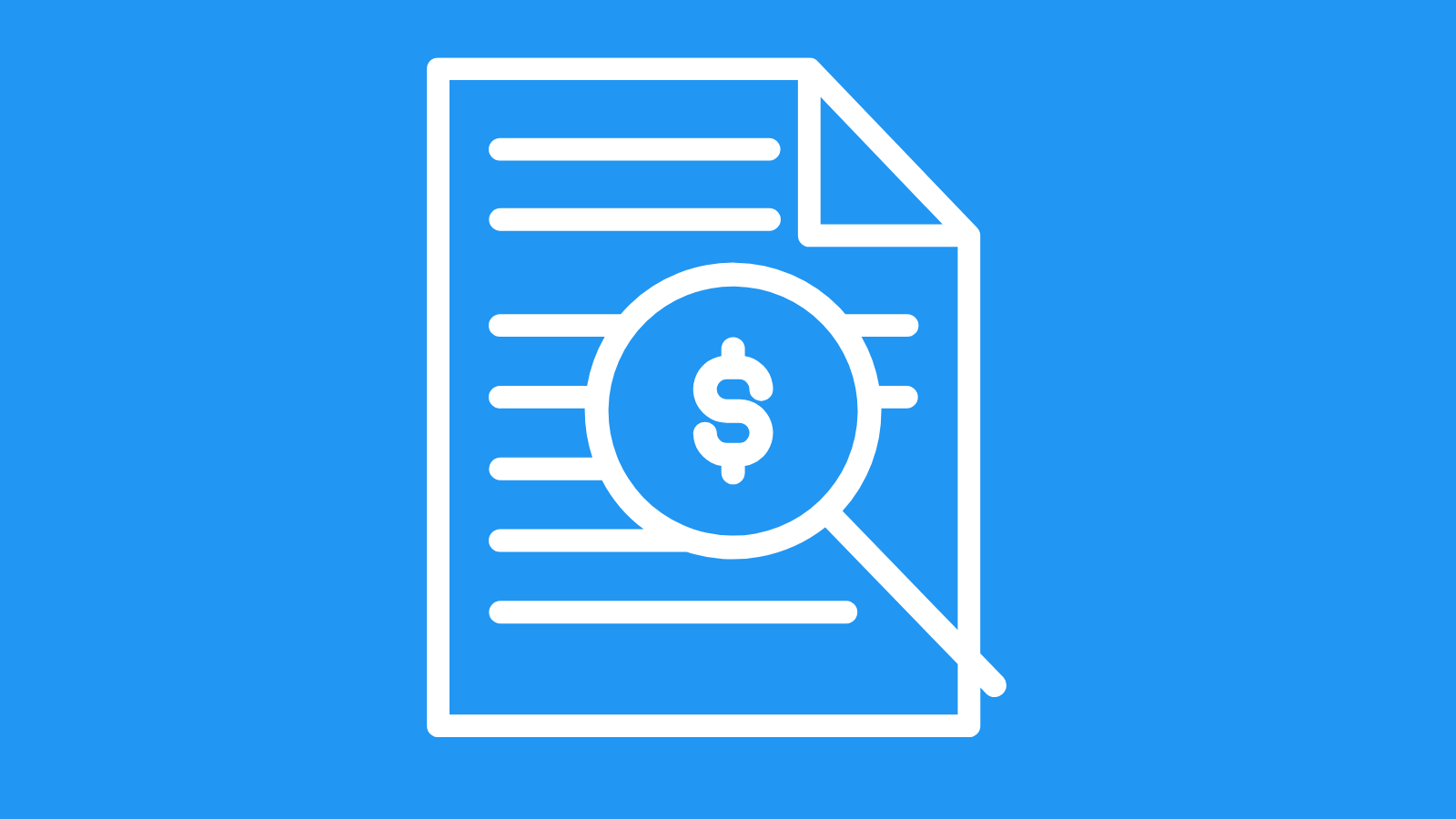
CRDF Stock Forecast Machine Learning Model
Our team, comprised of data scientists and economists, has developed a machine learning model to forecast the performance of Cardiff Oncology Inc. Common Stock (CRDF). The model leverages a comprehensive dataset encompassing both financial and non-financial indicators. Financial data incorporates quarterly and annual reports, including revenue, earnings per share (EPS), debt levels, and cash flow. We also incorporate market capitalization, price-to-earnings ratio (P/E), and other relevant valuation metrics. Non-financial factors, which are critical in the biotechnology sector, encompass clinical trial data, regulatory approvals, pipeline progress, and competitive landscape analysis. External factors, such as macroeconomic indicators (interest rates, inflation), sector-specific news, and overall market sentiment, are also incorporated to provide a holistic view.
We employ a hybrid approach, combining various machine learning algorithms for robustness and accuracy. These include time-series models (e.g., ARIMA, Prophet) to capture historical trends and seasonality, regression models (e.g., Random Forest, Gradient Boosting) to establish relationships between the independent variables and stock performance, and potentially, deep learning techniques (e.g., LSTMs) for advanced pattern recognition. Feature engineering plays a crucial role in enhancing model performance. This involves transforming raw data into meaningful features, such as calculating moving averages, generating lag variables, and incorporating sentiment scores derived from news articles and social media. The model's performance is evaluated using rigorous backtesting and validation techniques, employing metrics such as mean absolute error (MAE), root mean squared error (RMSE), and R-squared to assess forecast accuracy. Regular model retraining and recalibration are performed to adapt to changing market conditions and data availability.
The output of our model is a probabilistic forecast of CRDF's stock performance over specified time horizons (e.g., 3 months, 6 months, 1 year). The forecasts include predicted direction (up, down, or neutral) and confidence intervals, providing a measure of uncertainty. We are focused on providing actionable insights for informed decision-making. Furthermore, the model provides the ability to conduct scenario analysis, allowing users to simulate the impact of various events (e.g., clinical trial results, regulatory decisions) on the stock's potential performance. Our team regularly reviews and refines the model, incorporating feedback, new data sources, and emerging methodologies to maintain its relevance and predictive power. The model is a dynamic tool intended to support investment strategy and risk management regarding CRDF stock.
ML Model Testing
n:Time series to forecast
p:Price signals of Cardiff Oncology stock
j:Nash equilibria (Neural Network)
k:Dominated move of Cardiff Oncology stock holders
a:Best response for Cardiff Oncology target price
For further technical information as per how our model work we invite you to visit the article below:
How do KappaSignal algorithms actually work?
Cardiff Oncology Stock Forecast (Buy or Sell) Strategic Interaction Table
Strategic Interaction Table Legend:
X axis: *Likelihood% (The higher the percentage value, the more likely the event will occur.)
Y axis: *Potential Impact% (The higher the percentage value, the more likely the price will deviate.)
Z axis (Grey to Black): *Technical Analysis%
Cardiff Oncology: Financial Outlook and Forecast
Cardiff Oncology (CRDF) is a clinical-stage biotechnology company focused on the development of onvansertib, a Polo-like Kinase 1 (PLK1) inhibitor, for the treatment of cancer. CRDF's financial outlook hinges significantly on the successful clinical development and regulatory approval of onvansertib in various cancer indications. The company's primary value driver is its pipeline, particularly onvansertib, and its ability to demonstrate efficacy and safety in its ongoing and planned clinical trials. Market perception of CRDF is therefore tightly linked to clinical trial results and advancements. Financial performance is currently characterized by operating losses, typical of a pre-revenue biotechnology company, funded by capital raised through equity offerings. Revenue generation will primarily come from product sales following regulatory approval, which is still several years away. Furthermore, the company's financial health is dependent on its capacity to secure sufficient funding through public or private offerings, partnerships, and potential collaborations to support its extensive clinical development programs. Management's strategic decisions regarding capital allocation, clinical trial design, and potential partnerships will play a critical role in determining the company's long-term financial viability.
The forecast for CRDF's financial performance over the next several years projects continued operational losses. These losses will be primarily driven by research and development expenses, which are anticipated to remain high as clinical trials progress and potentially expand. Sales and marketing expenses will also increase as the company prepares for potential commercialization of onvansertib, should the clinical data support regulatory approval. The company's cash burn rate is a critical metric to monitor, as it indicates how quickly CRDF is utilizing its cash reserves to fund its operations. Projections for the company's cash runway will fluctuate depending on the success of future fundraising efforts and the pace of clinical trial spending. The company is at an inflection point, where the potential for positive clinical data to emerge could significantly influence investor sentiment and the ability to raise further capital. In order to survive and keep up with current expenses CRDF might consider cutting staff or other methods to manage expenses.
Key factors that will shape CRDF's future include the clinical outcomes of the ongoing trials of onvansertib in several cancer types such as KRAS-mutated metastatic colorectal cancer and metastatic pancreatic cancer. The company must show positive and statistically significant clinical results to achieve regulatory approval. The progress of these trials, including timelines, data readouts, and the ability to recruit and enroll patients, will be highly influential. Furthermore, the competitive landscape, including other companies developing oncology therapies, represents a significant external factor. Success depends on the speed of progress and the ability to stand out and produce better therapies. Strategic partnerships or collaborations with larger pharmaceutical companies could provide CRDF with additional resources, expertise, and potential revenue streams through milestone payments and royalties. The ability to successfully negotiate such partnerships will significantly influence the financial outlook. Additionally, the regulatory environment, including the speed and requirements for obtaining regulatory approvals, and the level of reimbursement from insurance companies will also play a critical role in the long-term financial success of CRDF.
The prediction for CRDF's financial outlook is cautiously optimistic. Positive clinical trial results of onvansertib and successful capital raising efforts should drive investor confidence, leading to long-term growth. However, this outlook is highly dependent on the clinical trials. The primary risk to this forecast is the failure of onvansertib to demonstrate compelling efficacy or safety, which could lead to a significant decline in the company's market value and difficulty in securing further funding. Regulatory setbacks and the increasing competition within the oncology space also represent substantial risks. Furthermore, the company's ability to effectively manage its cash burn rate and maintain adequate capital reserves is crucial for its survival and continued operations. Overall, the company has strong potential, but faces high uncertainty until further data are available.
Rating | Short-Term | Long-Term Senior |
---|---|---|
Outlook | B1 | B3 |
Income Statement | B1 | B3 |
Balance Sheet | Ba3 | C |
Leverage Ratios | C | Caa2 |
Cash Flow | B3 | B1 |
Rates of Return and Profitability | Baa2 | Caa2 |
*Financial analysis is the process of evaluating a company's financial performance and position by neural network. It involves reviewing the company's financial statements, including the balance sheet, income statement, and cash flow statement, as well as other financial reports and documents.
How does neural network examine financial reports and understand financial state of the company?
References
- Bengio Y, Schwenk H, SenĂ©cal JS, Morin F, Gauvain JL. 2006. Neural probabilistic language models. In Innovations in Machine Learning: Theory and Applications, ed. DE Holmes, pp. 137–86. Berlin: Springer
- Artis, M. J. W. Zhang (1990), "BVAR forecasts for the G-7," International Journal of Forecasting, 6, 349–362.
- D. Bertsekas. Dynamic programming and optimal control. Athena Scientific, 1995.
- B. Derfer, N. Goodyear, K. Hung, C. Matthews, G. Paoni, K. Rollins, R. Rose, M. Seaman, and J. Wiles. Online marketing platform, August 17 2007. US Patent App. 11/893,765
- Jorgenson, D.W., Weitzman, M.L., ZXhang, Y.X., Haxo, Y.M. and Mat, Y.X., 2023. Can Neural Networks Predict Stock Market?. AC Investment Research Journal, 220(44).
- Chen, C. L. Liu (1993), "Joint estimation of model parameters and outlier effects in time series," Journal of the American Statistical Association, 88, 284–297.
- K. Boda and J. Filar. Time consistent dynamic risk measures. Mathematical Methods of Operations Research, 63(1):169–186, 2006