AUC Score :
Short-term Tactic1 :
Dominant Strategy :
Time series to forecast n:
ML Model Testing : Multi-Instance Learning (ML)
Hypothesis Testing : Logistic Regression
Surveillance : Major exchange and OTC
1Short-term revised.
2Time series is updated based on short-term trends.
Key Points
Artelo's stock presents a high-risk, high-reward scenario. Predictions center on clinical trial outcomes for its cannabinoid-based therapies, particularly in cancer and anorexia, and positive results could trigger significant stock appreciation. Success depends on the successful completion of ongoing trials and subsequent regulatory approvals. Risks include clinical trial failures, regulatory setbacks, and potential competition from other pharmaceutical companies with similar drug candidates, which would severely depress the stock value. Further risks involve the company's ability to secure additional funding to support its operations and clinical programs.About Artelo Biosciences
Artelo Biosciences (ARTL) is a clinical-stage biopharmaceutical company focused on the development and commercialization of therapeutics that modulate the endocannabinoid system (ECS). The ECS plays a crucial role in regulating various physiological processes, including appetite, pain, inflammation, and mood. ARTL leverages its deep understanding of this system to create innovative medicines targeting unmet medical needs in oncology, pain management, and other therapeutic areas. The company's pipeline includes various drug candidates designed to interact with specific ECS receptors, with the aim of achieving therapeutic effects with potentially reduced side effects.
The company's strategy involves developing and advancing its drug candidates through clinical trials, aiming to demonstrate safety and efficacy. ARTL actively seeks to identify and address opportunities in areas where ECS modulation may offer therapeutic benefits. Furthermore, the company often collaborates with other biotechnology and pharmaceutical organizations to leverage their expertise and resources. ARTL's ongoing research and development efforts reflect its commitment to providing innovative solutions for patients suffering from debilitating diseases.
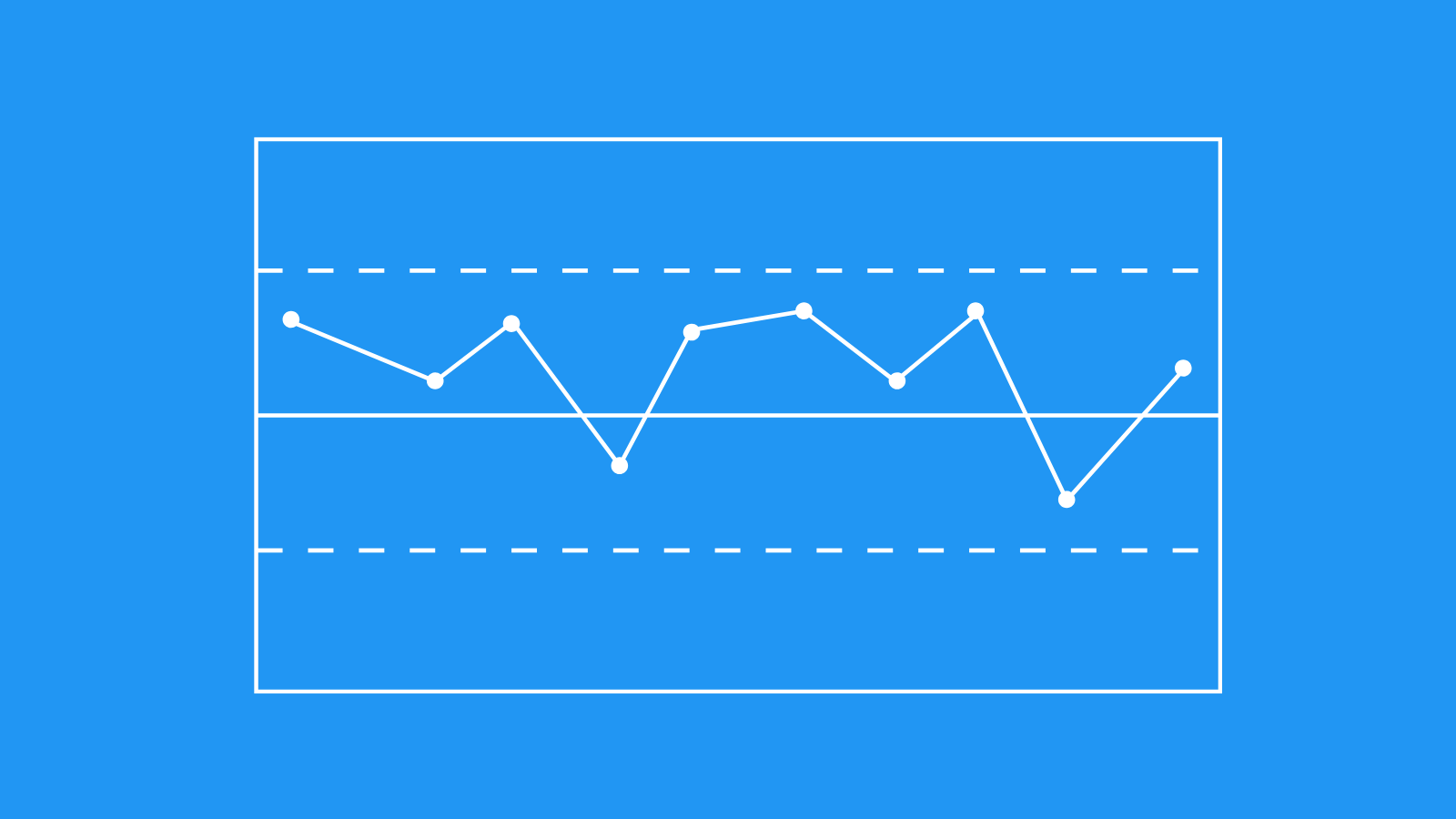
ARTL Stock Prediction Model
Our team proposes a comprehensive machine learning model to forecast the performance of Artelo Biosciences Inc. (ARTL) stock. The model will leverage a multi-faceted approach incorporating both fundamental and technical analysis. Fundamental analysis will involve extracting and processing data from Artelo's financial statements, including revenue, expenses, net income, and cash flow. We will also incorporate data related to Artelo's research and development pipeline, focusing on clinical trial progress, regulatory approvals, and the competitive landscape within the cannabinoid-based therapeutic market. Key economic indicators, such as interest rates, inflation, and market sentiment, which may impact the biotech industry, will also be integrated. These fundamental factors will provide insights into the intrinsic value and long-term growth potential of the company.
Technical analysis will be employed to identify patterns and trends in historical trading data. This will include utilizing time-series analysis techniques to analyze past price movements and trading volumes to anticipate future price fluctuations. We will consider various technical indicators, such as moving averages, Relative Strength Index (RSI), and Bollinger Bands, to detect potential buy and sell signals. Furthermore, we plan to incorporate natural language processing (NLP) to analyze news articles, social media sentiment, and analyst reports related to Artelo and the broader biotech sector. This will provide a real-time assessment of market perception and its impact on the stock price. This approach will help to predict short-term price volatility and market reactions to news events.
The machine learning model will employ a hybrid approach, combining the strengths of several algorithms. Initially, we will use regression models, such as Support Vector Regression (SVR) and Random Forest Regression, to establish relationships between fundamental and technical indicators with future stock performance. For time-series prediction, Recurrent Neural Networks (RNNs), particularly Long Short-Term Memory (LSTM) networks, will be utilized to capture sequential dependencies in historical data. The model's performance will be continuously monitored and validated using backtesting and real-time data analysis. We will also incorporate explainable AI (XAI) techniques to understand the model's decision-making process. Our approach strives to provide actionable insights for investors and to inform Artelo's financial strategies.
ML Model Testing
n:Time series to forecast
p:Price signals of Artelo Biosciences stock
j:Nash equilibria (Neural Network)
k:Dominated move of Artelo Biosciences stock holders
a:Best response for Artelo Biosciences target price
For further technical information as per how our model work we invite you to visit the article below:
How do KappaSignal algorithms actually work?
Artelo Biosciences Stock Forecast (Buy or Sell) Strategic Interaction Table
Strategic Interaction Table Legend:
X axis: *Likelihood% (The higher the percentage value, the more likely the event will occur.)
Y axis: *Potential Impact% (The higher the percentage value, the more likely the price will deviate.)
Z axis (Grey to Black): *Technical Analysis%
Artelo Biosciences Financial Outlook and Forecast
Artelo Biosciences (ARTL) is a clinical-stage biopharmaceutical company focused on developing novel therapeutics that modulate the endocannabinoid system (ECS). The company's financial outlook is largely tied to the success of its clinical trials, particularly its lead drug candidate, ART27.13, which is being developed for the treatment of anorexia associated with cancer and other indications. The company's strategy centers on leveraging the ECS, a complex signaling system involved in numerous physiological processes, to address unmet medical needs. Recent financings and collaborations will provide important resources for ongoing clinical trials and development programs. However, the development timeline for ART27.13 and other pipeline candidates remains subject to the inherent uncertainties of drug development. ARTL is a biotechnology company; the value is closely linked to the progress and outcomes of ongoing clinical trials, which often take an extended time frame.
The company's financial forecast hinges on several key factors, including clinical trial results, regulatory approvals, and potential partnerships. Positive data from Phase 2 and subsequent clinical trials for ART27.13 would significantly enhance ARTL's valuation and attract potential investors. Furthermore, obtaining regulatory approvals from authorities such as the U.S. Food and Drug Administration (FDA) or the European Medicines Agency (EMA) is critical for commercialization and revenue generation. Additionally, strategic collaborations with larger pharmaceutical companies could provide ARTL with much-needed financial backing and expertise to advance its drug development programs. The company currently has limited revenue, and its financials are significantly driven by research and development expenses. ARTL is currently in the preclinical and clinical stage of drug development and will not generate revenue until it is able to commercialize any products.
ARL's ability to secure additional funding through public offerings, private placements, or partnerships with other pharmaceutical companies will also significantly affect its outlook. Successful completion of clinical trials is essential to demonstrate the effectiveness and safety of the company's drug candidates, but the potential for unfavorable trial results, clinical trial delays, and difficulty in securing additional funding create substantial risks. The company may experience volatility in its stock price, making it a high-risk, high-reward investment. The success of ARTL is tightly linked to ART27.13's development. ARTL could face delays or fail to achieve a commercialized product from ART27.13 or any other drug in its pipeline, and this could reduce or eliminate the value of ARTL stock.
Overall, the financial forecast for ARTL remains positive, assuming successful execution of its clinical development strategy and regulatory approvals for ART27.13. It is expected that ART27.13 will be successful in treating anorexia. The company's strong focus on the ECS, coupled with its innovative pipeline, positions it well for future growth, provided it can overcome the inherent challenges of the biopharmaceutical industry. The primary risks to this positive outlook include: failure in clinical trials of ART27.13 or other pipeline candidates, regulatory setbacks, and the need for additional funding. Securing sufficient funding to continue operations and advance its drug development programs is critical for ARTL's long-term viability. Potential negative outcomes in clinical trials or a failure to secure additional funding could significantly impede the company's progress and negatively affect its financial outlook and stock price. Therefore, it is essential to closely monitor the company's clinical trial results, regulatory filings, and financial performance.
Rating | Short-Term | Long-Term Senior |
---|---|---|
Outlook | B3 | Caa1 |
Income Statement | Caa2 | Caa2 |
Balance Sheet | Caa2 | C |
Leverage Ratios | C | Caa2 |
Cash Flow | B2 | Caa2 |
Rates of Return and Profitability | B2 | C |
*Financial analysis is the process of evaluating a company's financial performance and position by neural network. It involves reviewing the company's financial statements, including the balance sheet, income statement, and cash flow statement, as well as other financial reports and documents.
How does neural network examine financial reports and understand financial state of the company?
References
- Wu X, Kumar V, Quinlan JR, Ghosh J, Yang Q, et al. 2008. Top 10 algorithms in data mining. Knowl. Inform. Syst. 14:1–37
- Chernozhukov V, Escanciano JC, Ichimura H, Newey WK. 2016b. Locally robust semiparametric estimation. arXiv:1608.00033 [math.ST]
- Vapnik V. 2013. The Nature of Statistical Learning Theory. Berlin: Springer
- H. Kushner and G. Yin. Stochastic approximation algorithms and applications. Springer, 1997.
- Athey S, Mobius MM, Pál J. 2017c. The impact of aggregators on internet news consumption. Unpublished manuscript, Grad. School Bus., Stanford Univ., Stanford, CA
- Christou, C., P. A. V. B. Swamy G. S. Tavlas (1996), "Modelling optimal strategies for the allocation of wealth in multicurrency investments," International Journal of Forecasting, 12, 483–493.
- Matzkin RL. 1994. Restrictions of economic theory in nonparametric methods. In Handbook of Econometrics, Vol. 4, ed. R Engle, D McFadden, pp. 2523–58. Amsterdam: Elsevier