AUC Score :
Short-term Tactic1 :
Dominant Strategy :
Time series to forecast n:
ML Model Testing : Reinforcement Machine Learning (ML)
Hypothesis Testing : Ridge Regression
Surveillance : Major exchange and OTC
1Short-term revised.
2Time series is updated based on short-term trends.
Key Points
Alto faces a mixed outlook. Production efficiency improvements and a focus on higher-margin products could lead to increased profitability. However, volatile commodity prices, particularly for corn and ethanol, pose a significant risk, potentially squeezing margins and impacting earnings negatively. Regulatory changes related to biofuels mandates and government policies could also create both opportunities and headwinds for Alto's business model, and competitive pressures within the ethanol market are a constant challenge. Furthermore, expansion into new areas like carbon capture and storage offers growth potential, but significant capital expenditures and regulatory hurdles are associated with these ventures, introducing uncertainty and potentially delaying returns on investment.About Alto Ingredients
Alto Ingredients, Inc., a prominent player in the renewable fuels and specialty alcohols sector, operates primarily in the United States. The company focuses on the production and marketing of a diverse range of products, including ethanol, which is a key component in gasoline blends. In addition to ethanol, Alto Ingredients also produces specialty alcohols used in various industrial and consumer applications, such as hand sanitizers and cleaning products. The company's operations are geographically diversified, with production facilities located across several states.
The company's strategy centers on capitalizing on the increasing demand for renewable fuels and sustainable products. Alto Ingredients emphasizes innovation and efficiency in its production processes. This involves investing in advanced technologies and optimizing its facilities to enhance its production capacity and reduce its environmental impact. Furthermore, the company continually seeks to expand its product portfolio and market reach to cater to the evolving needs of its customers and contribute to a more sustainable future.
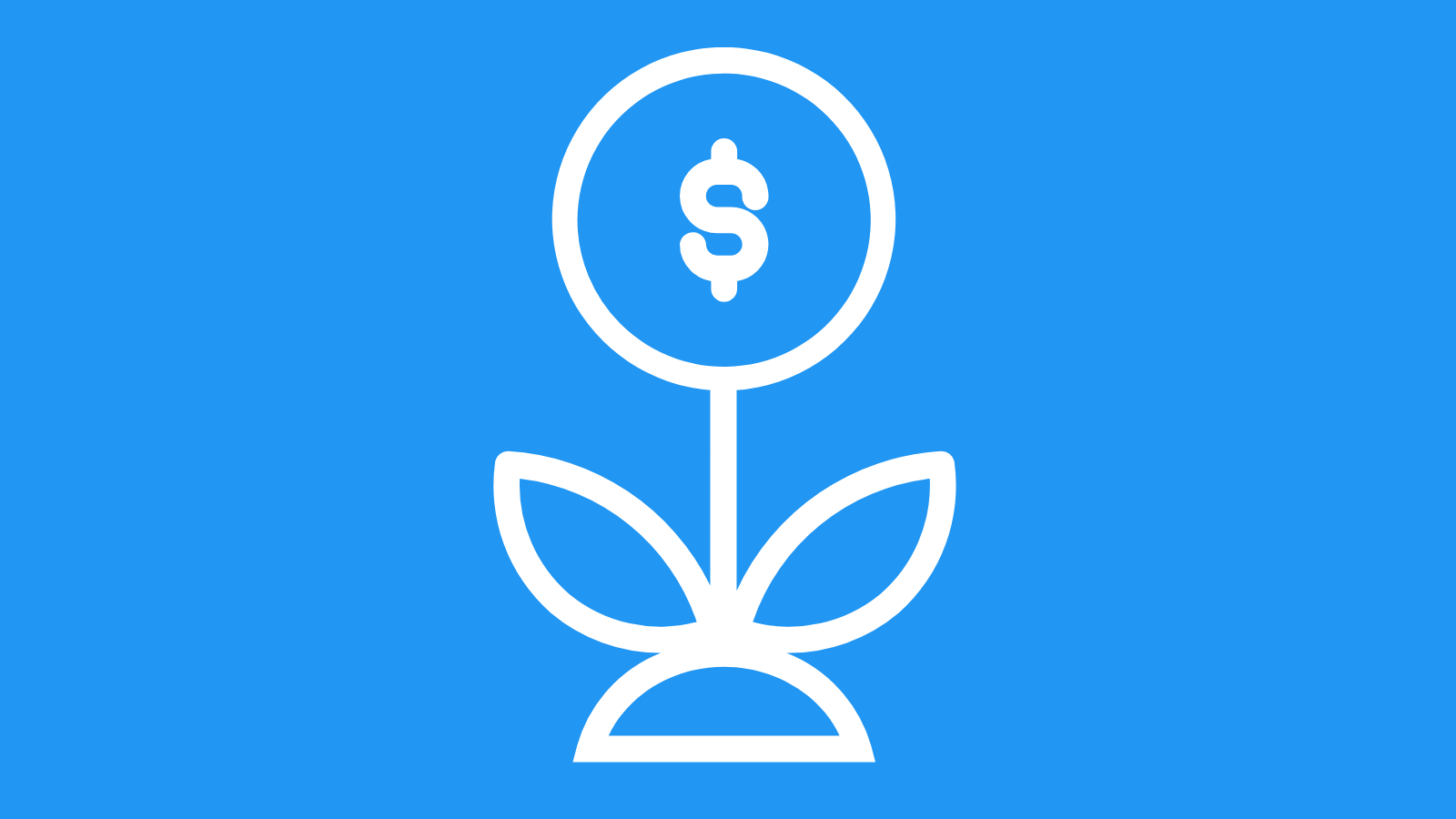
ALTO Stock Price Prediction Model
Our team proposes a sophisticated machine learning model for forecasting the future performance of Alto Ingredients Inc. (ALTO) common stock. This model leverages a diverse set of input features to capture the complex dynamics influencing the company's valuation. We will incorporate technical indicators derived from historical price and volume data, such as moving averages, Relative Strength Index (RSI), and Moving Average Convergence Divergence (MACD). We will also integrate fundamental factors, including quarterly and annual financial statements. These financial metrics will include revenue, earnings per share (EPS), debt-to-equity ratio, and profit margins. Macroeconomic indicators, such as inflation rates, interest rates, and commodity prices (especially corn and ethanol), will be included as well. These external variables are crucial, given Alto Ingredients' exposure to the agricultural and energy sectors.
The model will employ a hybrid approach to maximize predictive accuracy. We will begin with a thorough feature engineering process, including data normalization and the creation of lagged variables. This will be followed by the implementation of several machine learning algorithms, including a Recurrent Neural Network (RNN), specifically a Long Short-Term Memory (LSTM) network, to capture temporal dependencies in the data, along with a Random Forest model to account for non-linear relationships. We will train and test these models using historical ALTO data, dividing the dataset into training, validation, and testing sets. The model's performance will be evaluated based on metrics like Mean Absolute Error (MAE), Root Mean Squared Error (RMSE), and R-squared. The most performant model, or an ensemble of the top-performing models, will be selected for final predictions.
Regular model maintenance is essential. To ensure the model's continued accuracy, it will be retrained periodically with new data, incorporating potential shifts in market conditions or company performance. Model performance will be continuously monitored, with frequent re-evaluation of input features and model parameters. This ensures that the model remains robust and adaptable to the dynamic nature of the stock market. The final model will produce a forecast of ALTO's future performance, providing insights to assist with investment decisions. The model will output a range of possible outcomes and associated probabilities for investors and analysts to assess risk.
```
ML Model Testing
n:Time series to forecast
p:Price signals of Alto Ingredients stock
j:Nash equilibria (Neural Network)
k:Dominated move of Alto Ingredients stock holders
a:Best response for Alto Ingredients target price
For further technical information as per how our model work we invite you to visit the article below:
How do KappaSignal algorithms actually work?
Alto Ingredients Stock Forecast (Buy or Sell) Strategic Interaction Table
Strategic Interaction Table Legend:
X axis: *Likelihood% (The higher the percentage value, the more likely the event will occur.)
Y axis: *Potential Impact% (The higher the percentage value, the more likely the price will deviate.)
Z axis (Grey to Black): *Technical Analysis%
Alto Ingredients Inc. (ALTO) Financial Outlook and Forecast
ALTO, a leading producer of specialty alcohols and essential ingredients, presents a complex financial outlook, reflecting its position within the dynamic biofuels and agricultural sectors. The company's core business of producing ethanol and other products is significantly influenced by fluctuating commodity prices, government regulations, and consumer demand. Examining its recent performance indicates resilience in adapting to these challenges. ALTO has demonstrated an ability to capitalize on opportunities such as the demand for hand sanitizers during the COVID-19 pandemic, and expanding its specialty alcohol portfolio. However, the company's profitability can vary depending on ethanol margins and production costs. Factors such as corn prices, transportation expenses, and competition from other biofuel producers exert considerable pressure on its financial performance. To understand future financial outlook of ALTO, comprehensive analysis of these components is necessary to forecast future growth.
The financial forecast for ALTO hinges on several critical factors. Firstly, policy decisions regarding renewable fuel standards and incentives directly impact the demand for ethanol. Any shifts in these regulations could significantly influence ALTO's production volume and pricing power. Secondly, the global supply and demand dynamics for corn, a primary feedstock for ethanol production, will continue to affect its cost structure. Any unfavorable weather conditions or supply chain disruptions may cause significant fluctuations in the cost of raw materials, impacting profitability. Thirdly, the ongoing development and adoption of electric vehicles (EVs) could pose a long-term risk to the demand for gasoline, and consequently, ethanol. ALTO's ability to diversify its product offerings and explore alternative markets, such as sustainable aviation fuel and specialty alcohol applications, will be crucial in mitigating this risk. In addition, the company's strategic investments in plant upgrades and operational efficiencies are essential for improving its production margins and increasing profitability.
ALTO's future financial success depends on its ability to adapt to the rapidly changing industry landscape. The company's ability to manage its debt levels and maintain a strong balance sheet will be critical for navigating volatile market conditions and investing in future growth opportunities. ALTO has demonstrated some positive steps in this direction, including the acquisition of operations and the focus on expanding its product portfolio. Moreover, the company's expansion into sustainable aviation fuel (SAF) could offer attractive growth prospects as demand for SAF is expected to increase, although these new opportunities often come with significant initial capital expenditures. The success of ALTO's strategic initiatives depends on its ability to execute its plans effectively. Further diversification, through acquisitions or strategic partnerships could offer an additional revenue source and help to reduce its dependence on ethanol markets.
Overall, the outlook for ALTO is cautiously positive. The company's ability to adapt to changing regulations and market dynamics, combined with its strategic focus on expanding its product offerings, positions it for potential growth. The prediction is positive, but success is not guaranteed. However, there are considerable risks to this prediction. Negative risks may include unfavorable changes in government regulations, increased competition, and rising corn prices. Economic downturns and decreased consumer demand could also negatively affect its financial performance. Conversely, successful diversification into new markets, improved operational efficiencies, and favorable policy changes could drive higher revenue and profit, leading to significant returns for investors. The company's ability to navigate these risks and capitalize on opportunities will ultimately determine its long-term financial success.
Rating | Short-Term | Long-Term Senior |
---|---|---|
Outlook | B3 | B2 |
Income Statement | Caa2 | Baa2 |
Balance Sheet | Caa2 | C |
Leverage Ratios | Caa2 | B3 |
Cash Flow | C | B3 |
Rates of Return and Profitability | Baa2 | B2 |
*Financial analysis is the process of evaluating a company's financial performance and position by neural network. It involves reviewing the company's financial statements, including the balance sheet, income statement, and cash flow statement, as well as other financial reports and documents.
How does neural network examine financial reports and understand financial state of the company?
References
- Athey S, Tibshirani J, Wager S. 2016b. Generalized random forests. arXiv:1610.01271 [stat.ME]
- Semenova V, Goldman M, Chernozhukov V, Taddy M. 2018. Orthogonal ML for demand estimation: high dimensional causal inference in dynamic panels. arXiv:1712.09988 [stat.ML]
- Holland PW. 1986. Statistics and causal inference. J. Am. Stat. Assoc. 81:945–60
- Knox SW. 2018. Machine Learning: A Concise Introduction. Hoboken, NJ: Wiley
- Burgess, D. F. (1975), "Duality theory and pitfalls in the specification of technologies," Journal of Econometrics, 3, 105–121.
- Scott SL. 2010. A modern Bayesian look at the multi-armed bandit. Appl. Stoch. Models Bus. Ind. 26:639–58
- Rumelhart DE, Hinton GE, Williams RJ. 1986. Learning representations by back-propagating errors. Nature 323:533–36