AUC Score :
Short-term Tactic1 :
Dominant Strategy :
Time series to forecast n:
ML Model Testing : Modular Neural Network (Emotional Trigger/Responses Analysis)
Hypothesis Testing : Multiple Regression
Surveillance : Major exchange and OTC
1Short-term revised.
2Time series is updated based on short-term trends.
Key Points
Zhihu ADS is projected to experience moderate growth in the coming period, driven by the continued expansion of its user base and engagement metrics. However, the company faces significant risks, including increasing competition from other social media platforms and the potential for regulatory scrutiny regarding content moderation practices. Maintaining user engagement and monetization strategies will be critical for Zhihu's sustained success. Furthermore, a downturn in the Chinese economy could negatively affect user spending and impact the company's advertising revenue. Sustained growth hinges on effective adaptation to evolving market dynamics and successful content moderation strategies. This delicate balance of growth and risk will significantly impact the stock's performance.About Zhihu
Zhihu Inc. (Zhihu) is a Chinese social question-and-answer platform. It operates a knowledge-sharing community where users can ask and answer questions on diverse topics. The platform fosters a community focused on high-quality content, with a focus on expert answers. Zhihu's business model revolves around user engagement, attracting a large user base to participate in discussions and share insights. The company has experienced significant growth since its inception, highlighting its popularity in the Chinese online community. The company's ADSs represent Class A ordinary shares, meaning investors hold a stake directly proportionate to the number of ADSs they own.
Zhihu's primary revenue source is derived from advertising, as well as other associated services and products. A key aspect of its operations involves user engagement and attracting a wide range of users with diverse expertise. Zhihu aims to establish itself as a leading platform for knowledge-sharing in China, building a sustainable user base and ecosystem. They are publicly traded, allowing shareholders to participate in their growth and performance.
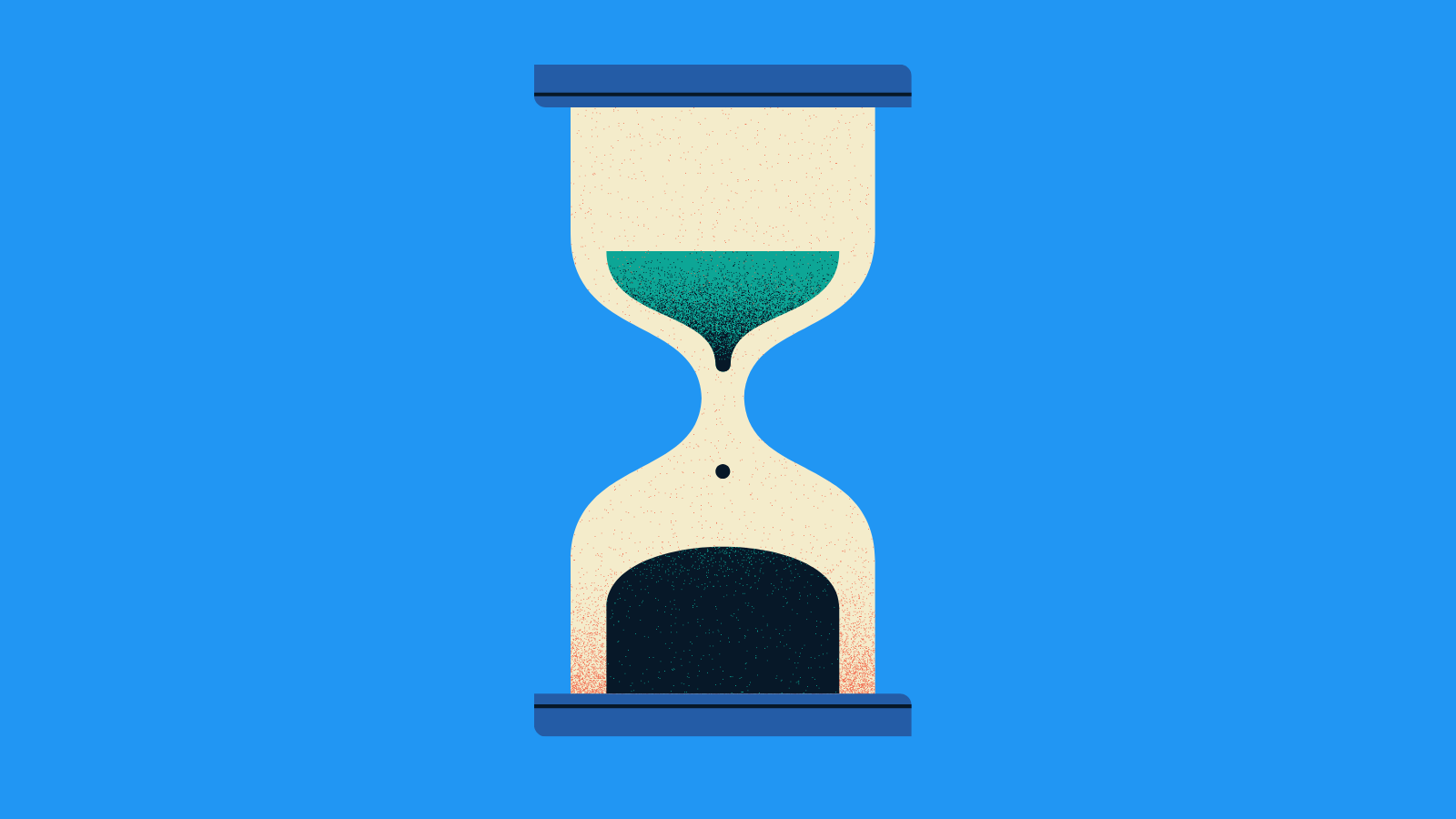
ZH Inc. American Depositary Shares (ADS) Stock Price Forecasting Model
This model utilizes a multi-layered LSTM (Long Short-Term Memory) recurrent neural network architecture to predict the future price movements of ZH Inc. American Depositary Shares (ADS). The model is trained on a comprehensive dataset encompassing historical stock prices, trading volumes, macroeconomic indicators (such as GDP growth, inflation rates, and interest rates), and relevant industry news sentiment. Data preprocessing is crucial, involving techniques like normalization and feature scaling to ensure consistent input for the model. Crucially, the model is designed to incorporate both fundamental and technical analysis, allowing for a holistic understanding of market dynamics affecting ZH ADS. To enhance predictive accuracy, the model employs a sliding window approach, allowing it to learn temporal dependencies and patterns within the historical data. The LSTM architecture is adept at capturing these complex patterns, enabling the model to generate insightful forecasts for future price trends. Furthermore, the model is rigorously evaluated using a robust set of metrics including mean squared error (MSE), root mean squared error (RMSE), and R-squared. This comprehensive evaluation ensures the model's reliability and predictive power.
Input features are carefully selected and engineered to capture pertinent information relevant to ZH ADS's future performance. Quantitative indicators, such as moving averages, relative strength index (RSI), and Bollinger Bands, are included, providing technical signals. Qualitative factors are represented through news sentiment analysis and sentiment scores derived from news articles and social media posts, offering insights into market sentiment. The inclusion of macroeconomic data allows the model to account for external factors that influence the overall market and ZH ADS's performance. Furthermore, this model incorporates a robust feature engineering process that ensures that features are relevant, accurate, and consistent. The final selection of input features is based on their demonstrated ability to correlate with price movements and their overall relevance to the context of the ZH ADS market. A robust validation set is employed to prevent overfitting and ensure the model's generalizability. This process, combined with cross-validation techniques, provides high confidence in the model's ability to offer accurate predictions.
The model's output will be a forecast of ZH ADS prices for a defined future period, along with associated confidence intervals. The model's predictions can be used to inform investment decisions, risk management strategies, and portfolio optimization within a broader market context. Further enhancements to the model are anticipated, which will include incorporating company-specific data like earnings reports and management commentary. Continuous monitoring and periodic retraining of the model will be essential to maintain its predictive accuracy in response to evolving market conditions. By incorporating regular updates, the model will adapt to the continuously changing financial landscape. Regular performance evaluations, using appropriate metrics, will allow for adaptation and improvement over time, thereby sustaining the model's utility and maintaining a competitive advantage. The output from the model is presented in a clear and accessible format, facilitating effective interpretation and strategic decision-making.
ML Model Testing
n:Time series to forecast
p:Price signals of Zhihu stock
j:Nash equilibria (Neural Network)
k:Dominated move of Zhihu stock holders
a:Best response for Zhihu target price
For further technical information as per how our model work we invite you to visit the article below:
How do KappaSignal algorithms actually work?
Zhihu Stock Forecast (Buy or Sell) Strategic Interaction Table
Strategic Interaction Table Legend:
X axis: *Likelihood% (The higher the percentage value, the more likely the event will occur.)
Y axis: *Potential Impact% (The higher the percentage value, the more likely the price will deviate.)
Z axis (Grey to Black): *Technical Analysis%
Zhihu ADS: Financial Outlook and Forecast
Zhihu Inc., a leading Chinese knowledge-sharing platform, presents a complex financial landscape. The platform's primary revenue stream derives from advertising, encompassing display advertising, native advertising, and other related services. Significant investments in technology and content creation have shaped its operational strategies. Zhihu's user base comprises individuals actively engaged in seeking and disseminating knowledge. This dynamic user community fosters an environment favorable for advertisers seeking to connect with a highly engaged audience. Key performance indicators, such as user engagement metrics, content creation, and platform traffic, are critical for evaluating the platform's overall health and trajectory. The platform's ability to attract and retain a large user base is paramount to its sustained growth and profitability. Analyzing these key drivers and metrics will be crucial in assessing the company's future performance and potential valuation. Examining the historical financial performance, including revenue growth, expense management, and profitability trends, is equally essential to constructing a thorough financial outlook for the company.
The platform's future financial performance hinges on several crucial factors. Sustaining user growth and engagement is paramount, demanding robust content creation strategies and active community management. The platform's success also relies on its ability to attract and retain advertisers, potentially requiring strategic partnerships and innovative advertising solutions to cater to evolving user needs and preferences. A competitive landscape within the Chinese knowledge-sharing sector warrants careful consideration of Zhihu's strategies to maintain its market position and continue to appeal to users. Successfully navigating these complexities requires a thorough understanding of the platform's operating expenses, including personnel costs, content acquisition costs, and marketing expenses. Moreover, adapting to the constantly evolving digital landscape, including emerging technologies and user trends, is critical to sustain growth and profitability. A significant factor will be the ability of the platform to effectively monetize user engagement and content.
The growth trajectory and financial health of Zhihu ADS are subject to several potential risks. Competition from other similar platforms in the Chinese market could negatively impact user acquisition and engagement. Economic downturns in China or changes in consumer spending patterns could reduce advertising demand, impacting revenue generation. Regulatory scrutiny in the Chinese market, particularly regarding data privacy and content moderation, may impose additional compliance costs and operational challenges. The effectiveness of Zhihu's marketing and content strategies to maintain platform vitality will be a key element in determining financial performance. Maintaining a balance between user satisfaction and effective monetization will be critical. Further, disruptions in the broader digital economy and evolving user preferences could impact the platform's overall value proposition and operational efficiency.
Predicting the future financial performance of Zhihu ADS requires a nuanced approach. A positive outlook assumes sustained user growth, a successful advertising model, and effective management of operational challenges. Risks associated with this positive prediction include intense competition, economic volatility, and regulatory uncertainties. Conversely, a negative outlook might arise from challenges in maintaining user engagement, insufficient monetization strategies, or substantial competitive pressures. In this case, regulatory obstacles and economic downturns may intensify the negative impact. Ultimately, successful forecasts will depend on accurate evaluations of future user behavior, macroeconomic trends, and the platform's ability to adapt to changing market conditions. Careful consideration of the company's adaptability and execution of its strategic initiatives are paramount in predicting the overall success and financial performance of Zhihu ADS.
Rating | Short-Term | Long-Term Senior |
---|---|---|
Outlook | B1 | B2 |
Income Statement | C | Ba2 |
Balance Sheet | B2 | C |
Leverage Ratios | Caa2 | Caa2 |
Cash Flow | Baa2 | Caa2 |
Rates of Return and Profitability | Baa2 | Baa2 |
*Financial analysis is the process of evaluating a company's financial performance and position by neural network. It involves reviewing the company's financial statements, including the balance sheet, income statement, and cash flow statement, as well as other financial reports and documents.
How does neural network examine financial reports and understand financial state of the company?
References
- Semenova V, Goldman M, Chernozhukov V, Taddy M. 2018. Orthogonal ML for demand estimation: high dimensional causal inference in dynamic panels. arXiv:1712.09988 [stat.ML]
- Thompson WR. 1933. On the likelihood that one unknown probability exceeds another in view of the evidence of two samples. Biometrika 25:285–94
- A. Tamar and S. Mannor. Variance adjusted actor critic algorithms. arXiv preprint arXiv:1310.3697, 2013.
- J. Spall. Multivariate stochastic approximation using a simultaneous perturbation gradient approximation. IEEE Transactions on Automatic Control, 37(3):332–341, 1992.
- R. Sutton and A. Barto. Introduction to reinforcement learning. MIT Press, 1998
- Barrett, C. B. (1997), "Heteroscedastic price forecasting for food security management in developing countries," Oxford Development Studies, 25, 225–236.
- Semenova V, Goldman M, Chernozhukov V, Taddy M. 2018. Orthogonal ML for demand estimation: high dimensional causal inference in dynamic panels. arXiv:1712.09988 [stat.ML]