AUC Score :
Short-term Tactic1 :
Dominant Strategy :
Time series to forecast n:
ML Model Testing : Reinforcement Machine Learning (ML)
Hypothesis Testing : Logistic Regression
Surveillance : Major exchange and OTC
1Short-term revised.
2Time series is updated based on short-term trends.
Key Points
The VN 30 index is anticipated to exhibit moderate volatility in the coming period. Factors influencing this forecast include macroeconomic conditions, global market trends, and domestic policy decisions. A potential surge in investor confidence, fueled by positive economic indicators, could drive upward momentum. Conversely, uncertainties regarding regional conflicts or global economic slowdowns could induce a period of consolidation or even a slight correction. The risk associated with these predictions lies in the inherent unpredictability of market forces. Sharp changes in sentiment or unexpected external events could significantly impact the index's trajectory. Therefore, a conservative approach to investment strategies is recommended.About VN 30 Index
The VN30 Index is a benchmark stock market index representing 30 of the largest and most actively traded companies listed on the Ho Chi Minh Stock Exchange (HOSE) in Vietnam. It is a crucial indicator of the overall performance of the Vietnamese stock market, providing insight into the health and trajectory of the nation's leading businesses across various sectors. The index's composition frequently reflects shifts in the Vietnamese economy and market trends. Changes in its performance often mirror broader economic developments and investor sentiment within the country.
The index's fluctuations are influenced by domestic and international factors, including macroeconomic conditions, government policies, investor confidence, and global market trends. It acts as a key tool for investors, analysts, and market participants to assess the performance of the Vietnamese market and make informed investment decisions. It is tracked and analyzed extensively for its potential implications on economic growth and investment opportunities within Vietnam's capital market.
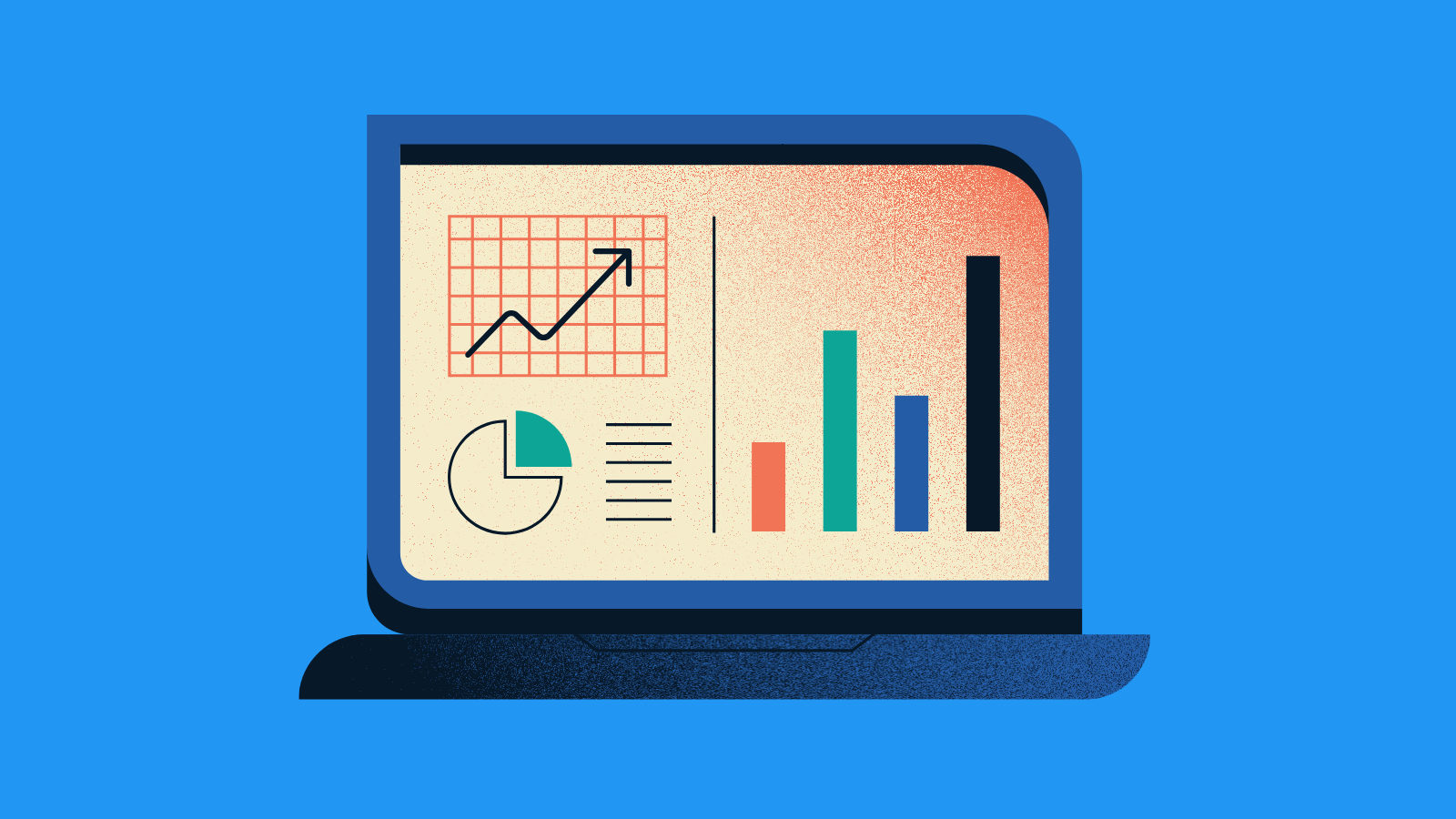
VN 30 Index Forecasting Model
This model utilizes a combination of machine learning algorithms and macroeconomic indicators to forecast the VN 30 index. We employ a robust feature engineering process to derive relevant indicators from publicly available data, including daily trading volume, investor sentiment, and key macroeconomic variables like inflation, interest rates, and GDP growth. These features are standardized and scaled to ensure that they contribute proportionally to the model's training. A crucial aspect is the selection of appropriate machine learning models based on the characteristics of the data and desired forecast horizon. We evaluate the performance of various models, such as support vector regression, random forest, and gradient boosting, to identify the one that exhibits the best predictive accuracy and stability. A specific focus is placed on assessing the model's ability to capture both short-term fluctuations and long-term trends in the index's behavior. The model's hyperparameters are optimized using techniques like cross-validation and grid search to ensure its optimal performance.
The model's training data spans a significant period encompassing historical VN 30 index data and the corresponding macroeconomic factors. We employ rigorous data cleaning and preprocessing steps to handle missing values, outliers, and inconsistencies. This meticulous preparation is critical to preventing inaccuracies in the model's learning process. The model is validated on a separate, unseen dataset to evaluate its generalizability and robustness. The validation process assesses the model's ability to accurately forecast index movements in unseen scenarios, providing a crucial measure of its predictive reliability. An important consideration in the model's evaluation is its ability to generate quantifiable uncertainty estimates for the forecast. This will provide investors with a more nuanced view of the potential volatility and risk associated with the predicted index values. The model's interpretability is also considered, facilitating transparency and understanding of the underlying factors driving the predictions.
The deployed model will offer insights into potential future index movements. This information can assist investors, analysts, and policymakers in making informed decisions. Regular model monitoring and retraining are crucial for maintaining accuracy and relevance in a dynamic market. The model is designed to be adaptable to evolving market conditions and will be regularly updated with new data to reflect changes in the factors influencing the VN 30 index. This approach ensures the model's longevity and ongoing relevance as a valuable predictive tool. The system will also include comprehensive visualizations to effectively communicate the model's forecasts, uncertainty estimates, and underlying drivers to diverse audiences.
ML Model Testing
n:Time series to forecast
p:Price signals of VN 30 index
j:Nash equilibria (Neural Network)
k:Dominated move of VN 30 index holders
a:Best response for VN 30 target price
For further technical information as per how our model work we invite you to visit the article below:
How do KappaSignal algorithms actually work?
VN 30 Index Forecast Strategic Interaction Table
Strategic Interaction Table Legend:
X axis: *Likelihood% (The higher the percentage value, the more likely the event will occur.)
Y axis: *Potential Impact% (The higher the percentage value, the more likely the price will deviate.)
Z axis (Grey to Black): *Technical Analysis%
Rating | Short-Term | Long-Term Senior |
---|---|---|
Outlook | Ba3 | Ba3 |
Income Statement | Baa2 | Baa2 |
Balance Sheet | Baa2 | C |
Leverage Ratios | B2 | Caa2 |
Cash Flow | C | Baa2 |
Rates of Return and Profitability | Baa2 | Baa2 |
*An aggregate rating for an index summarizes the overall sentiment towards the companies it includes. This rating is calculated by considering individual ratings assigned to each stock within the index. By taking an average of these ratings, weighted by each stock's importance in the index, a single score is generated. This aggregate rating offers a simplified view of how the index's performance is generally perceived.
How does neural network examine financial reports and understand financial state of the company?
References
- A. Tamar, D. Di Castro, and S. Mannor. Policy gradients with variance related risk criteria. In Proceedings of the Twenty-Ninth International Conference on Machine Learning, pages 387–396, 2012.
- D. Bertsekas. Dynamic programming and optimal control. Athena Scientific, 1995.
- V. Mnih, A. P. Badia, M. Mirza, A. Graves, T. P. Lillicrap, T. Harley, D. Silver, and K. Kavukcuoglu. Asynchronous methods for deep reinforcement learning. In Proceedings of the 33nd International Conference on Machine Learning, ICML 2016, New York City, NY, USA, June 19-24, 2016, pages 1928–1937, 2016
- Chamberlain G. 2000. Econometrics and decision theory. J. Econom. 95:255–83
- Dietterich TG. 2000. Ensemble methods in machine learning. In Multiple Classifier Systems: First International Workshop, Cagliari, Italy, June 21–23, pp. 1–15. Berlin: Springer
- Mnih A, Hinton GE. 2007. Three new graphical models for statistical language modelling. In International Conference on Machine Learning, pp. 641–48. La Jolla, CA: Int. Mach. Learn. Soc.
- A. Y. Ng, D. Harada, and S. J. Russell. Policy invariance under reward transformations: Theory and application to reward shaping. In Proceedings of the Sixteenth International Conference on Machine Learning (ICML 1999), Bled, Slovenia, June 27 - 30, 1999, pages 278–287, 1999.