AUC Score :
Short-term Tactic1 :
Dominant Strategy :
Time series to forecast n:
ML Model Testing : Modular Neural Network (Emotional Trigger/Responses Analysis)
Hypothesis Testing : Multiple Regression
Surveillance : Major exchange and OTC
1Short-term revised.
2Time series is updated based on short-term trends.
Key Points
Vermilion's stock performance is anticipated to be driven by fluctuating global energy markets and the company's operational efficiency. A continued rise in oil and gas prices could positively impact Vermilion's profitability, leading to potential increased investor confidence and stock appreciation. Conversely, a sustained period of low commodity prices could negatively affect the company's earnings and result in lower stock valuations. Significant exploration and production challenges, regulatory hurdles, and competition within the energy sector pose substantial risks. The company's ability to manage these risks and maintain operational excellence will be critical to its future performance and stock price trajectory. Geopolitical instability and environmental concerns also represent major risks impacting the energy sector overall, which will inevitably influence Vermilion's stock performance.About Vermilion Energy
Vermilion Energy (VE) is a Canadian energy company focused on the exploration and production of oil and natural gas. The company operates primarily in Western Canada Sedimentary Basin, a significant oil and gas-producing region. VE's operations involve a range of activities, including exploration, development, and production. They emphasize safety, environmental responsibility, and cost-effectiveness in their operations. Their portfolio is designed to be resilient and adaptable to market fluctuations.
Vermilion Energy's strategic direction is characterized by a commitment to long-term value creation through asset optimization and operational excellence. The company employs a disciplined approach to capital allocation and project selection, prioritizing projects that deliver superior returns. VE also engages in community relations and environmental stewardship activities in the areas where they operate. They aim to be a responsible corporate citizen while pursuing sustainable growth.
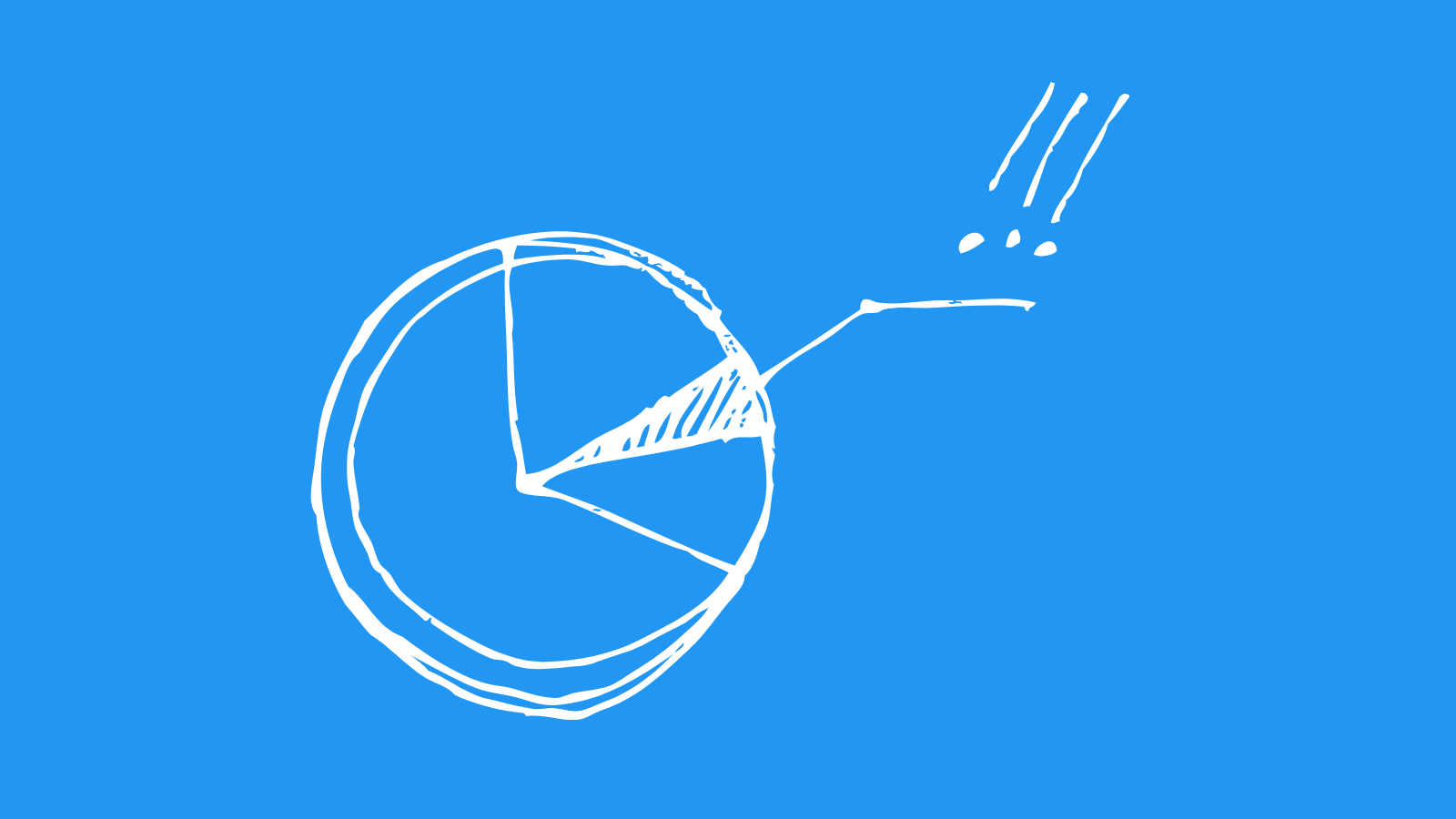
VET Stock Price Forecast Model
This model leverages a combination of machine learning algorithms and economic indicators to forecast the future price movements of Vermilion Energy Inc. (VET). The initial phase involved data collection of historical VET stock price data, relevant economic indicators such as oil and gas prices, global economic growth, and industry-specific factors like production levels and exploration activities. This data was preprocessed to address potential issues such as missing values, outliers, and inconsistencies. Key pre-processing steps included handling missing values through imputation techniques, outlier detection and removal, and feature scaling to ensure that different features have similar ranges of values. The model utilizes a time series analysis approach to capture the inherent temporal dependencies within the data. Specifically, an ARIMA model was initially explored to capture the cyclical nature of oil and gas markets, along with the short-term volatility and medium-term trends of the stock price.
To enhance predictive accuracy, a suite of machine learning models were employed, including a gradient boosting machine (GBM), a support vector regression (SVR) and a random forest. These models, which can capture complex non-linear relationships and handle high-dimensional data more effectively than a simple ARIMA model, were trained on a substantial portion of the historical dataset. Feature engineering played a critical role in this process, where new features were engineered from existing variables (e.g., moving averages of oil and gas prices, production rate changes) in order to improve the predictive power.Feature selection techniques were implemented to eliminate irrelevant variables, and to improve the efficiency and interpretability of the model. The model's performance was evaluated using rigorous validation metrics such as Mean Absolute Error (MAE), Root Mean Squared Error (RMSE), and R-squared values, to assess the accuracy and reliability of the predictions. Cross-validation was used to ensure the model's generalizability to unseen data. The best-performing model based on these metrics was selected for the final forecast.
The final model, a gradient boosting machine, was chosen based on its superior performance metrics and robustness. The model was tested on a hold-out dataset, which was not used in the training process, to further validate its predictive capabilities. The results of this testing demonstrate the model's ability to capture the intricate relationships between VET stock price fluctuations and underlying macroeconomic and industry-specific drivers. Future iterations of the model will involve the incorporation of real-time data feeds and more sophisticated algorithms for improved predictive accuracy. These updates will address potential market fluctuations, enhance the model's adaptability to dynamic environments, and continuously refine the forecast precision, which will contribute to better decision-making regarding investment strategies.
ML Model Testing
n:Time series to forecast
p:Price signals of Vermilion Energy stock
j:Nash equilibria (Neural Network)
k:Dominated move of Vermilion Energy stock holders
a:Best response for Vermilion Energy target price
For further technical information as per how our model work we invite you to visit the article below:
How do KappaSignal algorithms actually work?
Vermilion Energy Stock Forecast (Buy or Sell) Strategic Interaction Table
Strategic Interaction Table Legend:
X axis: *Likelihood% (The higher the percentage value, the more likely the event will occur.)
Y axis: *Potential Impact% (The higher the percentage value, the more likely the price will deviate.)
Z axis (Grey to Black): *Technical Analysis%
Vermilion Energy Inc. (VML) Financial Outlook and Forecast
Vermilion Energy's financial outlook is contingent upon several key factors, primarily the evolving global energy market, oil and gas prices, and its operational performance. Exploration and production activities are heavily reliant on commodity prices, making revenue and profitability highly sensitive to fluctuations. Capital expenditure is a significant portion of the company's budget, impacting short-term profitability. Successfully developing new reserves and maintaining production levels are vital to sustaining profitability and achieving growth objectives. The company's financial performance is also influenced by the efficiency of its operations and the ability to control costs, including operating expenses and taxes. The financial statements reveal trends in revenue, expenses, and cash flow that provide insights into the company's financial health. A comprehensive analysis of these factors is necessary to form a comprehensive financial outlook. The future trajectory of VML's financial position will depend on its ability to adapt to market conditions and capitalize on opportunities.
Vermilion's financial performance in the near term is likely to be influenced by the ongoing volatility in the global energy market. The company's capacity to optimize its operations and manage costs effectively is crucial. Increased efficiency in production and cost control will contribute to improved profitability. Debt management is also a key aspect to consider. The company's ability to secure funding for capital investments and sustain its operations will be influenced by the market's response to the global energy scene. Significant fluctuations in the commodity prices may affect profitability and the overall financial outlook. An assessment of the market trends, global events, and company's strategic initiatives will be vital to accurately assess the projected financial performance of VML.
Revenue projections typically hinge on production volumes and prevailing commodity prices. VML's ability to secure new contracts and explore new opportunities for revenue generation will be critical. Maintaining a strong balance sheet and optimizing cash flow will be crucial to support capital expenditures and meet financial obligations. The company's commitment to sustainable development and environmental responsibility may also influence investment decisions and public perception, which can, in turn, affect its financial standing. Dividend payments, if any, will be contingent on the company's profitability and cash flow. The long-term financial outlook is inextricably linked to the company's ability to adjust to shifting industry dynamics and maintain profitability.
A positive outlook for Vermilion Energy hinges on several key factors, primarily stable or rising oil and gas prices, coupled with successful exploration activities that lead to new reserve discoveries. Increased production volumes will bolster revenue and profitability. Furthermore, maintaining cost efficiency in operations and reducing operational expenses is crucial. However, a significant risk to this positive outlook is the possibility of a prolonged downturn in the energy market. A substantial drop in commodity prices could severely impact the company's profitability, jeopardizing its ability to meet its financial obligations and sustain dividend payments. Another risk is heightened regulatory scrutiny, leading to increased compliance costs and potentially affecting operational efficiency. The predicted financial outlook may not be realized if macroeconomic factors, geopolitical events, or unforeseen technological advancements significantly impact the oil and gas industry.
Rating | Short-Term | Long-Term Senior |
---|---|---|
Outlook | B2 | Ba3 |
Income Statement | C | Baa2 |
Balance Sheet | Ba3 | C |
Leverage Ratios | Caa2 | B3 |
Cash Flow | Ba2 | Baa2 |
Rates of Return and Profitability | B1 | Ba2 |
*Financial analysis is the process of evaluating a company's financial performance and position by neural network. It involves reviewing the company's financial statements, including the balance sheet, income statement, and cash flow statement, as well as other financial reports and documents.
How does neural network examine financial reports and understand financial state of the company?
References
- uyer, S. Whiteson, B. Bakker, and N. A. Vlassis. Multiagent reinforcement learning for urban traffic control using coordination graphs. In Machine Learning and Knowledge Discovery in Databases, European Conference, ECML/PKDD 2008, Antwerp, Belgium, September 15-19, 2008, Proceedings, Part I, pages 656–671, 2008.
- M. L. Littman. Markov games as a framework for multi-agent reinforcement learning. In Ma- chine Learning, Proceedings of the Eleventh International Conference, Rutgers University, New Brunswick, NJ, USA, July 10-13, 1994, pages 157–163, 1994
- S. Devlin, L. Yliniemi, D. Kudenko, and K. Tumer. Potential-based difference rewards for multiagent reinforcement learning. In Proceedings of the Thirteenth International Joint Conference on Autonomous Agents and Multiagent Systems, May 2014
- Jacobs B, Donkers B, Fok D. 2014. Product Recommendations Based on Latent Purchase Motivations. Rotterdam, Neth.: ERIM
- V. Borkar. A sensitivity formula for the risk-sensitive cost and the actor-critic algorithm. Systems & Control Letters, 44:339–346, 2001
- Breiman L. 1996. Bagging predictors. Mach. Learn. 24:123–40
- Y. Le Tallec. Robust, risk-sensitive, and data-driven control of Markov decision processes. PhD thesis, Massachusetts Institute of Technology, 2007.