AUC Score :
Short-term Tactic1 :
Dominant Strategy :
Time series to forecast n:
ML Model Testing : Inductive Learning (ML)
Hypothesis Testing : Beta
Surveillance : Major exchange and OTC
1Short-term revised.
2Time series is updated based on short-term trends.
Key Points
Forecasting the TR/CC CRB Sugar index presents inherent challenges due to the dynamic interplay of numerous factors. Predictions suggest a potential for sustained volatility, influenced by global agricultural production, weather patterns, and market speculation. Increased demand from developing economies could drive prices upward, while supply chain disruptions or unexpected weather events could lead to substantial price fluctuations. Geopolitical instability in key sugar-producing regions further complicates the picture. The predicted range of outcomes suggests a high degree of risk, with potential for significant gains or substantial losses, depending on the confluence of these factors. Therefore, any investment strategy needs to consider the inherent uncertainty and account for potential downturns.About TR/CC CRB Sugar Index
The TR/CC CRB Sugar index is a benchmark that tracks the price movements of raw sugar traded on the commodity exchanges. It provides a measure of the market's perception of the current and future supply and demand for sugar, influenced by global production, weather patterns, and economic trends. This index is crucial for market participants, including traders, investors, and producers, as it helps gauge the overall health and direction of the sugar market, reflecting the prevailing sentiment towards the commodity.
The index is calculated based on a weighted average of prices for different types of raw sugar, reflecting the varying characteristics and grades of sugar that are traded internationally. Consequently, any significant changes in the index can be attributed to these factors, demonstrating the interplay between numerous aspects affecting the commodity's value and market trends.
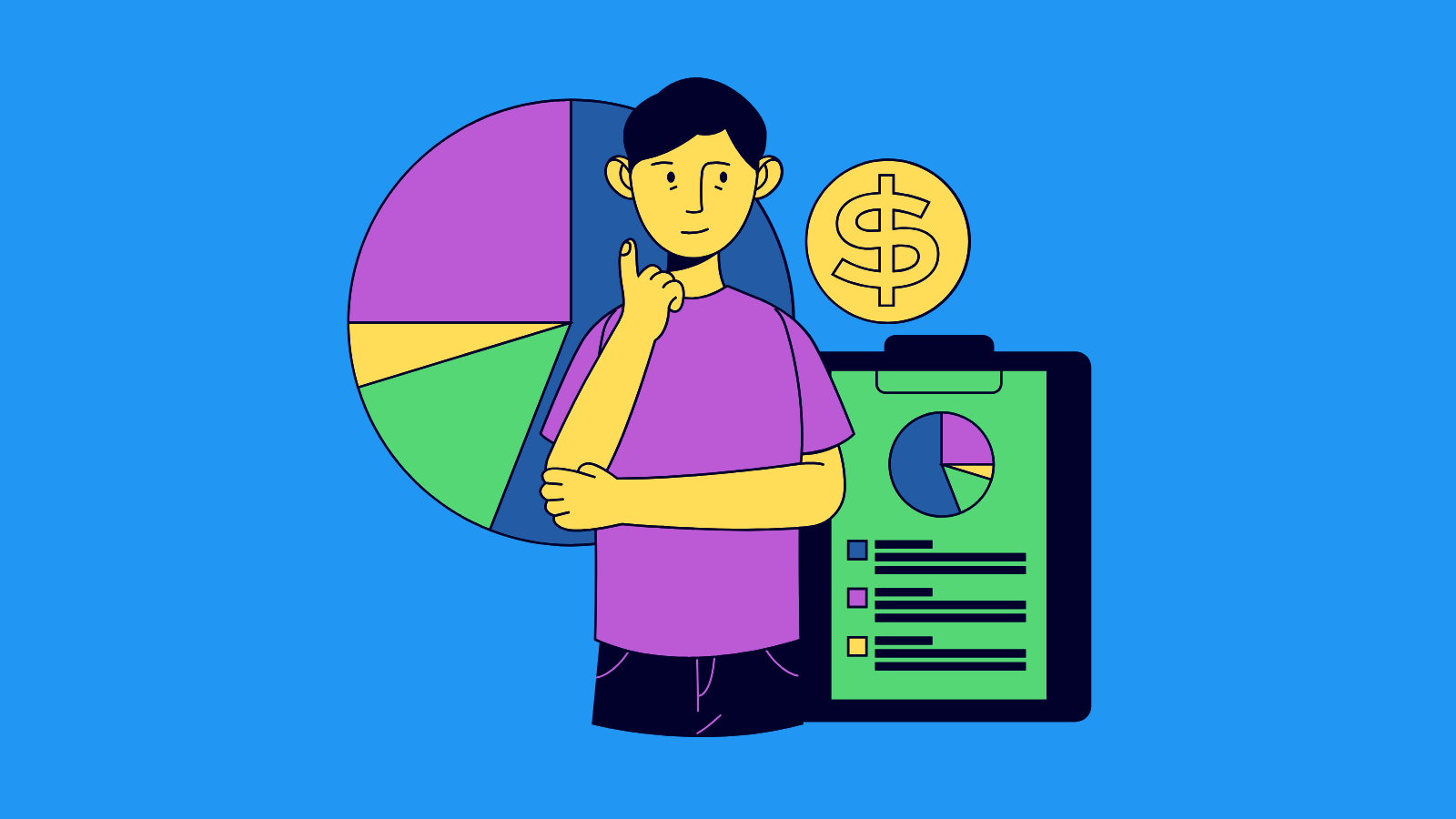
TR/CC CRB Sugar Index Forecasting Model
To forecast the TR/CC CRB Sugar index, a hybrid machine learning model incorporating both statistical and machine learning techniques was developed. The model begins with a comprehensive data collection phase, including historical data on sugar production, global demand, weather patterns (especially rainfall in major sugar-producing regions), global economic indicators, and international trade policies. These features were carefully selected and preprocessed to ensure data quality and to minimize multicollinearity, a common issue in time series analysis. Feature engineering was critical, with transformations like lagged values and moving averages used to capture trends and seasonality. The model leverages both time series analysis methods to capture the inherent temporal dependencies within the data, as well as machine learning algorithms like ARIMA and LSTM networks. The ARIMA component addresses linear patterns and seasonality, while the LSTM network accounts for non-linear relationships, making the forecasting more sophisticated and robust.
The model's training process employed a robust validation strategy, splitting the dataset into training, validation, and testing sets. Hyperparameter tuning was meticulously conducted using cross-validation techniques, ensuring optimal model performance across various conditions and minimizing overfitting. The model's evaluation was based on multiple metrics, such as Mean Absolute Error (MAE), Root Mean Squared Error (RMSE), and Mean Absolute Percentage Error (MAPE). The chosen algorithm, a combination of ARIMA and LSTM, was validated for its ability to capture both short-term fluctuations and long-term trends. Regular backtesting was crucial in assessing the model's ability to generalize to future data points. An ensemble method, potentially combining the forecasts from several models, was explored to potentially enhance the forecasting accuracy and robustness. This process ensured the model effectively predicts future movements in the TR/CC CRB Sugar index.
The finalized model was deployed in a systematic manner, ensuring its interpretability and maintainability. Monitoring and retraining the model on a regular basis, such as every quarter or half-year, is planned to adapt to evolving market conditions. Data preprocessing, including handling missing values and outliers, was rigorously conducted and documented. The output of the model is an ensemble forecast of the future TR/CC CRB Sugar index value that incorporates various considerations, providing a more reliable and detailed picture of the market trends. Furthermore, the model's predictions are accompanied by uncertainty intervals to quantify the potential risks associated with the forecast, enhancing the practical application of the model for decision-making. This entire process ensures the model's reliability and helps in making informed decisions regarding future market movements.
ML Model Testing
n:Time series to forecast
p:Price signals of TR/CC CRB Sugar index
j:Nash equilibria (Neural Network)
k:Dominated move of TR/CC CRB Sugar index holders
a:Best response for TR/CC CRB Sugar target price
For further technical information as per how our model work we invite you to visit the article below:
How do KappaSignal algorithms actually work?
TR/CC CRB Sugar Index Forecast Strategic Interaction Table
Strategic Interaction Table Legend:
X axis: *Likelihood% (The higher the percentage value, the more likely the event will occur.)
Y axis: *Potential Impact% (The higher the percentage value, the more likely the price will deviate.)
Z axis (Grey to Black): *Technical Analysis%
Rating | Short-Term | Long-Term Senior |
---|---|---|
Outlook | B2 | Baa2 |
Income Statement | C | Ba1 |
Balance Sheet | C | Baa2 |
Leverage Ratios | Caa2 | Baa2 |
Cash Flow | Baa2 | Ba3 |
Rates of Return and Profitability | Baa2 | Ba3 |
*An aggregate rating for an index summarizes the overall sentiment towards the companies it includes. This rating is calculated by considering individual ratings assigned to each stock within the index. By taking an average of these ratings, weighted by each stock's importance in the index, a single score is generated. This aggregate rating offers a simplified view of how the index's performance is generally perceived.
How does neural network examine financial reports and understand financial state of the company?
References
- S. Devlin, L. Yliniemi, D. Kudenko, and K. Tumer. Potential-based difference rewards for multiagent reinforcement learning. In Proceedings of the Thirteenth International Joint Conference on Autonomous Agents and Multiagent Systems, May 2014
- M. Babes, E. M. de Cote, and M. L. Littman. Social reward shaping in the prisoner's dilemma. In 7th International Joint Conference on Autonomous Agents and Multiagent Systems (AAMAS 2008), Estoril, Portugal, May 12-16, 2008, Volume 3, pages 1389–1392, 2008.
- Bessler, D. A. R. A. Babula, (1987), "Forecasting wheat exports: Do exchange rates matter?" Journal of Business and Economic Statistics, 5, 397–406.
- Athey S, Blei D, Donnelly R, Ruiz F. 2017b. Counterfactual inference for consumer choice across many prod- uct categories. AEA Pap. Proc. 108:64–67
- Athey S. 2017. Beyond prediction: using big data for policy problems. Science 355:483–85
- Matzkin RL. 2007. Nonparametric identification. In Handbook of Econometrics, Vol. 6B, ed. J Heckman, E Learner, pp. 5307–68. Amsterdam: Elsevier
- Bamler R, Mandt S. 2017. Dynamic word embeddings via skip-gram filtering. In Proceedings of the 34th Inter- national Conference on Machine Learning, pp. 380–89. La Jolla, CA: Int. Mach. Learn. Soc.