AUC Score :
Short-term Tactic1 :
Dominant Strategy :
Time series to forecast n:
ML Model Testing : Modular Neural Network (Financial Sentiment Analysis)
Hypothesis Testing : ElasticNet Regression
Surveillance : Major exchange and OTC
1Short-term revised.
2Time series is updated based on short-term trends.
Key Points
The TR/CC CRB Heating Oil index is anticipated to exhibit moderate volatility in the coming period. Factors influencing this forecast include global economic conditions, energy market dynamics, and potential geopolitical events. Forecasts suggest a slight upward trend, driven by anticipated increases in demand and potential supply constraints. However, this upward trajectory may be tempered by fluctuating crude oil prices and broader market sentiment. Risks associated with these predictions include unexpected disruptions to global energy supply, shifts in consumer demand, and unforeseen macroeconomic developments. These risks could cause significant deviations from the predicted trend.About TR/CC CRB Heating Oil Index
The TR/CC CRB Heating Oil index is a market-based indicator reflecting the current price of heating oil. It's compiled and disseminated by a recognized commodity exchange, such as the CRB, and represents the average price across various traded grades and locations. This index provides a benchmark for market participants, including energy companies, traders, and consumers, to assess current market conditions and make informed decisions regarding buying, selling, and forecasting future trends. The index is often used in contracts and agreements related to heating oil.
The index's fluctuations are influenced by several factors, including global supply and demand, weather patterns, geopolitical events, and economic conditions. Understanding these influences is crucial for interpreting the index's variations. Importantly, it should be recognized that the index is a snapshot of a specific time and location, and may not directly reflect all aspects of the broader heating oil market or localized prices.
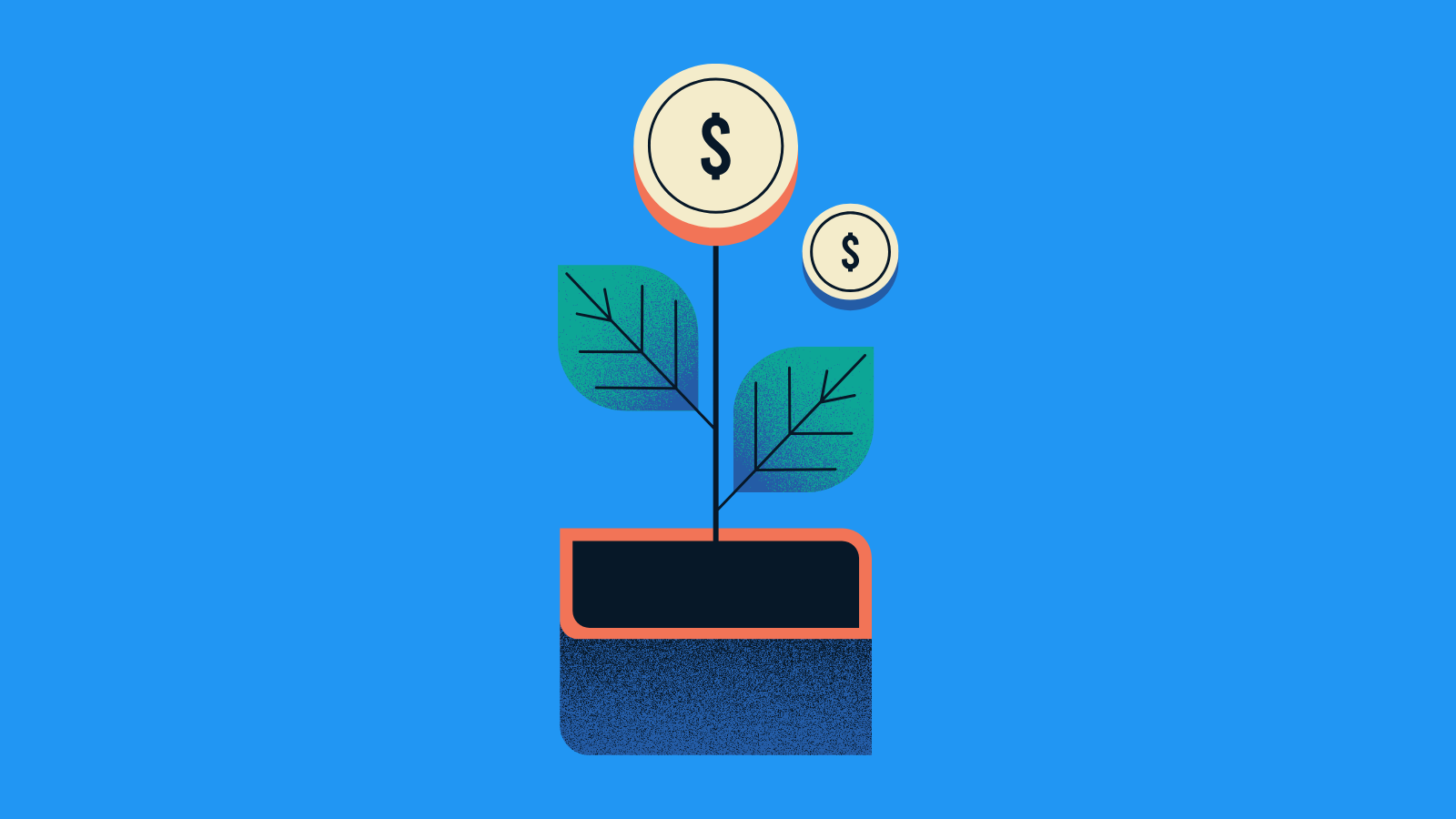
TR/CC CRB Heating Oil Index Price Forecasting Model
This model utilizes a time series forecasting approach to predict the TR/CC CRB Heating Oil index. A crucial component of the model is data preprocessing. Historical data, encompassing a comprehensive range of factors affecting the index (e.g., global energy demand, crude oil prices, weather patterns, and economic indicators), is meticulously cleaned and transformed. This involves handling missing values, outlier detection, and feature scaling to ensure data integrity and model efficiency. Key features are selected through feature engineering and dimensionality reduction techniques like Principal Component Analysis (PCA), optimizing the model's performance by focusing on the most relevant data. A robust time series decomposition is performed to isolate trend, seasonality, and cyclical components within the data, providing a deeper understanding of the underlying patterns. Finally, a carefully selected forecasting algorithm is deployed. ARIMA (Autoregressive Integrated Moving Average) models and/or SARIMA models are employed, which have proven effective in capturing the dynamic nature of commodity indices. These algorithms leverage past index values and identified patterns to generate predictions for future values.
Model validation is a critical stage. Cross-validation techniques are implemented to assess the model's generalizability and robustness across different periods. This ensures that the model's performance is not overly reliant on the specific training data. Metrics such as Mean Absolute Error (MAE), Root Mean Squared Error (RMSE), and Mean Absolute Percentage Error (MAPE) are used to evaluate the predictive accuracy of the model. Regularized regression techniques, like Ridge or Lasso, can be incorporated for improved model stability and reduced overfitting, especially when dealing with high-dimensional data. The results of these evaluations are rigorously scrutinized to identify any potential biases or weaknesses in the model's predictions. Further model tuning and refinement may be necessary to improve forecasting accuracy based on these findings. Periodic re-evaluation of the model is crucial for maintaining predictive accuracy given the dynamic nature of commodity markets and the potential for changes in the influencing variables.
Model deployment involves integrating the chosen model into a production system to generate real-time predictions. This requires careful consideration of computational efficiency and scalability to handle the increasing volume of data and requests. A comprehensive documentation of the model's architecture, data sources, and validation procedures is essential for transparency and reproducibility. Monitoring the model's performance in a live environment is vital to identify any deterioration in predictive accuracy over time. Regular updates to the model using fresh data are necessary to adapt to evolving market conditions. Continuous monitoring and retraining will ensure that the model remains effective in forecasting the TR/CC CRB Heating Oil index under diverse market circumstances. This ongoing refinement is key to the model's long-term effectiveness and reliability.
ML Model Testing
n:Time series to forecast
p:Price signals of TR/CC CRB Heating Oil index
j:Nash equilibria (Neural Network)
k:Dominated move of TR/CC CRB Heating Oil index holders
a:Best response for TR/CC CRB Heating Oil target price
For further technical information as per how our model work we invite you to visit the article below:
How do KappaSignal algorithms actually work?
TR/CC CRB Heating Oil Index Forecast Strategic Interaction Table
Strategic Interaction Table Legend:
X axis: *Likelihood% (The higher the percentage value, the more likely the event will occur.)
Y axis: *Potential Impact% (The higher the percentage value, the more likely the price will deviate.)
Z axis (Grey to Black): *Technical Analysis%
TR/CC CRB Heating Oil Financial Outlook and Forecast
The financial outlook for the TR/CC CRB heating oil market hinges on a complex interplay of global energy dynamics, economic growth projections, and geopolitical events. Current market analysis suggests a mixed picture. While the overall demand for heating oil is anticipated to remain steady in the coming quarters, the price trajectory is uncertain. Factors influencing this prediction include the pace of global economic recovery, the potential for extreme weather events, and the continued volatility in international energy markets. The current geopolitical climate, specifically any escalation of tensions or disruptions in supply chains, could significantly impact the market's stability and price trends. Recent developments in renewable energy adoption and energy efficiency initiatives are also important considerations; these trends, if successful, may reduce future demand for traditional heating sources like heating oil in the long term, albeit with varying degrees of impact on the short-term market.
Several macroeconomic indicators are critical to understanding the market's direction. A robust global economy, with sustained industrial activity and consumer spending, would likely support higher demand for heating oil, translating to increased prices. Conversely, a slowdown in economic growth or a recessionary period could depress demand and lead to downward pressure on prices. Fluctuations in crude oil prices, a key input to heating oil production, will also exert a significant influence on the market. Changes in the cost of refining heating oil, alongside production costs and transportation expenses, affect the final product pricing. Investors need to closely monitor these factors to gauge the trajectory of the TR/CC CRB heating oil market.
Seasonal factors also play a pivotal role. The market's performance is often intertwined with the specific demands of the heating season. A particularly harsh winter could significantly increase heating demand, leading to higher prices. Conversely, a mild winter could result in subdued demand and thus potentially lower prices. Historical data on heating oil prices and the impact of weather patterns can be invaluable in anticipating such seasonal shifts. Ultimately, an accurate forecast requires a granular understanding of these intersecting economic and environmental factors. Additionally, any unforeseen disruptions, such as unexpected pipeline closures or refinery problems, must be considered when constructing a market outlook.
Predicting the future price trajectory of the TR/CC CRB heating oil market presents a challenge. A positive outlook suggests that with moderate economic growth and manageable supply chain disruptions, heating oil prices will likely remain stable in the near term. However, this optimistic prediction carries risks. Geopolitical instability, unexpected weather patterns, or significant shifts in energy policy could quickly impact the market, leading to substantial price fluctuations. Furthermore, the long-term trend towards renewable energy sources poses a substantial risk to the traditional fossil fuel sector, potentially diminishing demand for heating oil in the future. While a positive outlook relies on moderate economic growth and sustainable supply chains, the risks associated with unforeseen events and long-term energy trends demand a cautious approach to market participation.
Rating | Short-Term | Long-Term Senior |
---|---|---|
Outlook | B2 | Ba3 |
Income Statement | Baa2 | Caa2 |
Balance Sheet | B3 | Baa2 |
Leverage Ratios | Caa2 | Caa2 |
Cash Flow | C | Caa2 |
Rates of Return and Profitability | Caa2 | Baa2 |
*An aggregate rating for an index summarizes the overall sentiment towards the companies it includes. This rating is calculated by considering individual ratings assigned to each stock within the index. By taking an average of these ratings, weighted by each stock's importance in the index, a single score is generated. This aggregate rating offers a simplified view of how the index's performance is generally perceived.
How does neural network examine financial reports and understand financial state of the company?
References
- N. B ̈auerle and J. Ott. Markov decision processes with average-value-at-risk criteria. Mathematical Methods of Operations Research, 74(3):361–379, 2011
- V. Borkar. Q-learning for risk-sensitive control. Mathematics of Operations Research, 27:294–311, 2002.
- K. Tumer and D. Wolpert. A survey of collectives. In K. Tumer and D. Wolpert, editors, Collectives and the Design of Complex Systems, pages 1–42. Springer, 2004.
- R. Sutton and A. Barto. Introduction to reinforcement learning. MIT Press, 1998
- V. Borkar. Stochastic approximation: a dynamical systems viewpoint. Cambridge University Press, 2008
- Athey S, Imbens G, Wager S. 2016a. Efficient inference of average treatment effects in high dimensions via approximate residual balancing. arXiv:1604.07125 [math.ST]
- Chipman HA, George EI, McCulloch RE. 2010. Bart: Bayesian additive regression trees. Ann. Appl. Stat. 4:266–98