AUC Score :
Short-term Tactic1 :
Dominant Strategy :
Time series to forecast n:
ML Model Testing : Multi-Instance Learning (ML)
Hypothesis Testing : Ridge Regression
Surveillance : Major exchange and OTC
1Short-term revised.
2Time series is updated based on short-term trends.
Key Points
The TR/CC CRB ex Energy ER index is anticipated to experience moderate fluctuations in the coming period, driven by the interplay of global economic conditions, supply chain dynamics, and commodity prices. Potential upward pressure could stem from increasing demand for raw materials in emerging markets and ongoing geopolitical tensions, however headwinds from rising interest rates and potential global recessionary pressures could lead to a downtrend. The risk associated with these predictions includes the possibility of unforeseen external events significantly impacting the index's trajectory, such as sudden shifts in investor sentiment or drastic changes in geopolitical landscapes. Volatility is therefore expected to remain a key characteristic of the index's performance.About TR/CC CRB ex Energy ER Index
This exclusive content is only available to premium users.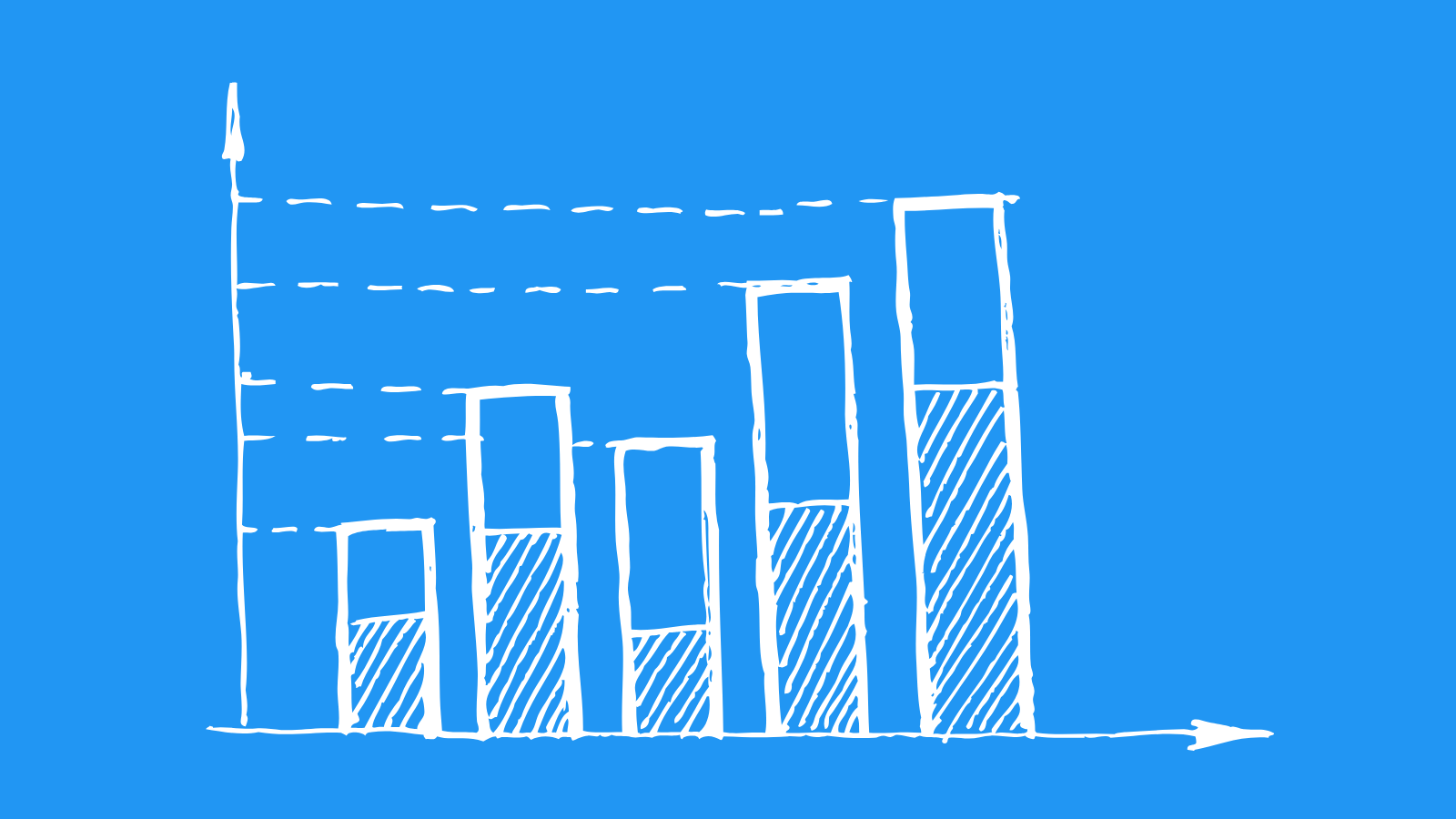
TR/CC CRB ex Energy ER Index Forecasting Model
This model utilizes a combination of time series analysis and machine learning techniques to forecast the TR/CC CRB ex Energy ER index. Initial data preprocessing focuses on handling missing values and outliers, crucial for ensuring model robustness. We employ a robust statistical approach to identify and address potential seasonality and trends within the time series data. To capture complex relationships and non-linear patterns, a Gradient Boosting model is chosen. This model's flexibility allows it to learn intricate correlations between various macroeconomic indicators, such as GDP growth, inflation rates, and interest rates, that are potentially correlated to the index movements. Feature engineering is essential, creating derived variables from the original data. For example, moving averages and indicators that demonstrate trends over time are calculated to enhance the model's predictive power. Cross-validation techniques are rigorously applied to ensure that the model generalizes well to unseen data, thereby minimizing the risk of overfitting.
Model training involves separating the historical data into training and testing sets. The Gradient Boosting model is trained on the training data, iteratively adjusting its parameters to minimize prediction errors. The testing set is used to evaluate the model's performance on unseen data, assessing its ability to predict future index values accurately. Crucial metrics for evaluating the model's performance include mean absolute error (MAE), root mean squared error (RMSE), and R-squared. Furthermore, we investigate the model's stability over time through backtesting procedures. Model performance is benchmarked against other competing models using similar data, and a thorough sensitivity analysis examining the impact of different hyperparameters on prediction accuracy is conducted. This process helps refine the model's architecture and maximize its forecasting capabilities.
Finally, a comprehensive risk assessment and uncertainty analysis are performed to quantify the potential errors inherent in the model's predictions. Confidence intervals are established around the predicted values to account for inherent variability and provide a range of possible outcomes. This crucial step allows stakeholders to assess the potential for both upside and downside risks. The model is then deployed and integrated into a real-time monitoring system. Regular model retraining on new data and monitoring of performance metrics is essential to ensure ongoing accuracy and effectiveness. This iterative approach to model development ensures its suitability for dynamic market conditions. A detailed documentation outlining the model's inputs, outputs, limitations, and validation results is produced for transparency and maintainability.
ML Model Testing
n:Time series to forecast
p:Price signals of TR/CC CRB ex Energy ER index
j:Nash equilibria (Neural Network)
k:Dominated move of TR/CC CRB ex Energy ER index holders
a:Best response for TR/CC CRB ex Energy ER target price
For further technical information as per how our model work we invite you to visit the article below:
How do KappaSignal algorithms actually work?
TR/CC CRB ex Energy ER Index Forecast Strategic Interaction Table
Strategic Interaction Table Legend:
X axis: *Likelihood% (The higher the percentage value, the more likely the event will occur.)
Y axis: *Potential Impact% (The higher the percentage value, the more likely the price will deviate.)
Z axis (Grey to Black): *Technical Analysis%
TR/CC CRB ex Energy ER Index Financial Outlook and Forecast
The TR/CC CRB ex Energy ER Index, a composite measure of commodity prices excluding energy components, presents a complex financial landscape for investors. Its performance is intrinsically linked to various global economic factors, including industrial output, raw material demand, and geopolitical events. A comprehensive analysis requires scrutinizing the interconnectedness of these variables to understand likely future trends. Key considerations include the strength of global manufacturing activity, the pace of emerging market growth, and the potential impact of supply chain disruptions. Understanding the historical relationship between the index and these external factors is crucial for making informed predictions. Analyzing historical data alongside expert commentary can provide insights into the potential for sustained growth or contraction.
Several economic indicators provide context for forecasting the TR/CC CRB ex Energy ER Index. The International Monetary Fund's (IMF) World Economic Outlook, alongside reports from organizations like the Organization for Economic Co-operation and Development (OECD), offer valuable insight into global growth prospects. The degree of optimism or pessimism surrounding future economic activity directly influences commodity demand. Inflationary pressures and interest rate decisions from central banks significantly affect raw material prices. Examining trends in import/export data from key economies can also reveal potential shifts in demand patterns, ultimately impacting the index. Further, examining supply chain bottlenecks, and the dynamics of producer and consumer confidence, provide a clearer picture of the potential for volatility.
Current forecasts for the TR/CC CRB ex Energy ER Index generally suggest a moderate outlook. While some analysts anticipate a continuation of the index's growth momentum driven by continued industrial activity, others emphasize potential headwinds. Geopolitical uncertainty, particularly regarding international trade relations and potential conflict, remains a significant source of risk. These uncertainties may lead to temporary setbacks in commodity prices and subsequent fluctuations in the index's value. A detailed analysis of the global manufacturing environment, agricultural production, and resource availability is vital to discern the drivers behind any short-term fluctuations and assess the sustainability of the long-term trend. Considering these factors will potentially allow investors to gauge the level of risk associated with various investment strategies.
Predicting the future direction of the TR/CC CRB ex Energy ER Index is challenging. A positive prediction would hinge on sustained global economic growth, increased industrial output, and favorable supply chain conditions. However, risks to this positive outlook include potential economic slowdowns, supply chain disruptions, unexpected geopolitical tensions, and unforeseen inflationary pressures. Conversely, a negative prediction could stem from weakening economic activity, falling demand for raw materials, and lingering supply chain constraints. Investors should be prepared for potential volatility in the index and should consider diversifying their investment portfolio to mitigate potential risks. Ultimately, a cautious approach, grounded in thorough research and continuous monitoring of key economic indicators, is vital for navigating the complexities of this market. The index's future performance will likely be a reflection of the interaction of these multiple, interconnected factors.
Rating | Short-Term | Long-Term Senior |
---|---|---|
Outlook | B2 | Caa1 |
Income Statement | B3 | B1 |
Balance Sheet | Caa2 | C |
Leverage Ratios | Caa2 | C |
Cash Flow | Caa2 | Caa2 |
Rates of Return and Profitability | Baa2 | C |
*An aggregate rating for an index summarizes the overall sentiment towards the companies it includes. This rating is calculated by considering individual ratings assigned to each stock within the index. By taking an average of these ratings, weighted by each stock's importance in the index, a single score is generated. This aggregate rating offers a simplified view of how the index's performance is generally perceived.
How does neural network examine financial reports and understand financial state of the company?
References
- Dimakopoulou M, Zhou Z, Athey S, Imbens G. 2018. Balanced linear contextual bandits. arXiv:1812.06227 [cs.LG]
- Hornik K, Stinchcombe M, White H. 1989. Multilayer feedforward networks are universal approximators. Neural Netw. 2:359–66
- A. Tamar and S. Mannor. Variance adjusted actor critic algorithms. arXiv preprint arXiv:1310.3697, 2013.
- Zeileis A, Hothorn T, Hornik K. 2008. Model-based recursive partitioning. J. Comput. Graph. Stat. 17:492–514 Zhou Z, Athey S, Wager S. 2018. Offline multi-action policy learning: generalization and optimization. arXiv:1810.04778 [stat.ML]
- Scholkopf B, Smola AJ. 2001. Learning with Kernels: Support Vector Machines, Regularization, Optimization, and Beyond. Cambridge, MA: MIT Press
- D. S. Bernstein, S. Zilberstein, and N. Immerman. The complexity of decentralized control of Markov Decision Processes. In UAI '00: Proceedings of the 16th Conference in Uncertainty in Artificial Intelligence, Stanford University, Stanford, California, USA, June 30 - July 3, 2000, pages 32–37, 2000.
- J. Filar, D. Krass, and K. Ross. Percentile performance criteria for limiting average Markov decision pro- cesses. IEEE Transaction of Automatic Control, 40(1):2–10, 1995.