AUC Score :
Short-term Tactic1 :
Dominant Strategy :
Time series to forecast n:
ML Model Testing : Multi-Task Learning (ML)
Hypothesis Testing : Lasso Regression
Surveillance : Major exchange and OTC
1Short-term revised.
2Time series is updated based on short-term trends.
Key Points
Tenet Healthcare's future performance hinges on several factors. Sustained operational efficiency and effective cost management are crucial to profitability. Success in the implementation of strategic initiatives, like expanding market share in specific service areas, will also be a significant driver of the company's long-term value. However, challenges associated with competitive pressures and potential fluctuations in the healthcare industry's regulatory environment present significant risks. Further, patient volume and payer mix can impact profitability and financial stability. These challenges, if not adequately addressed, could lead to a decline in investor confidence and a subsequent negative impact on share price.About Tenet Healthcare
This exclusive content is only available to premium users.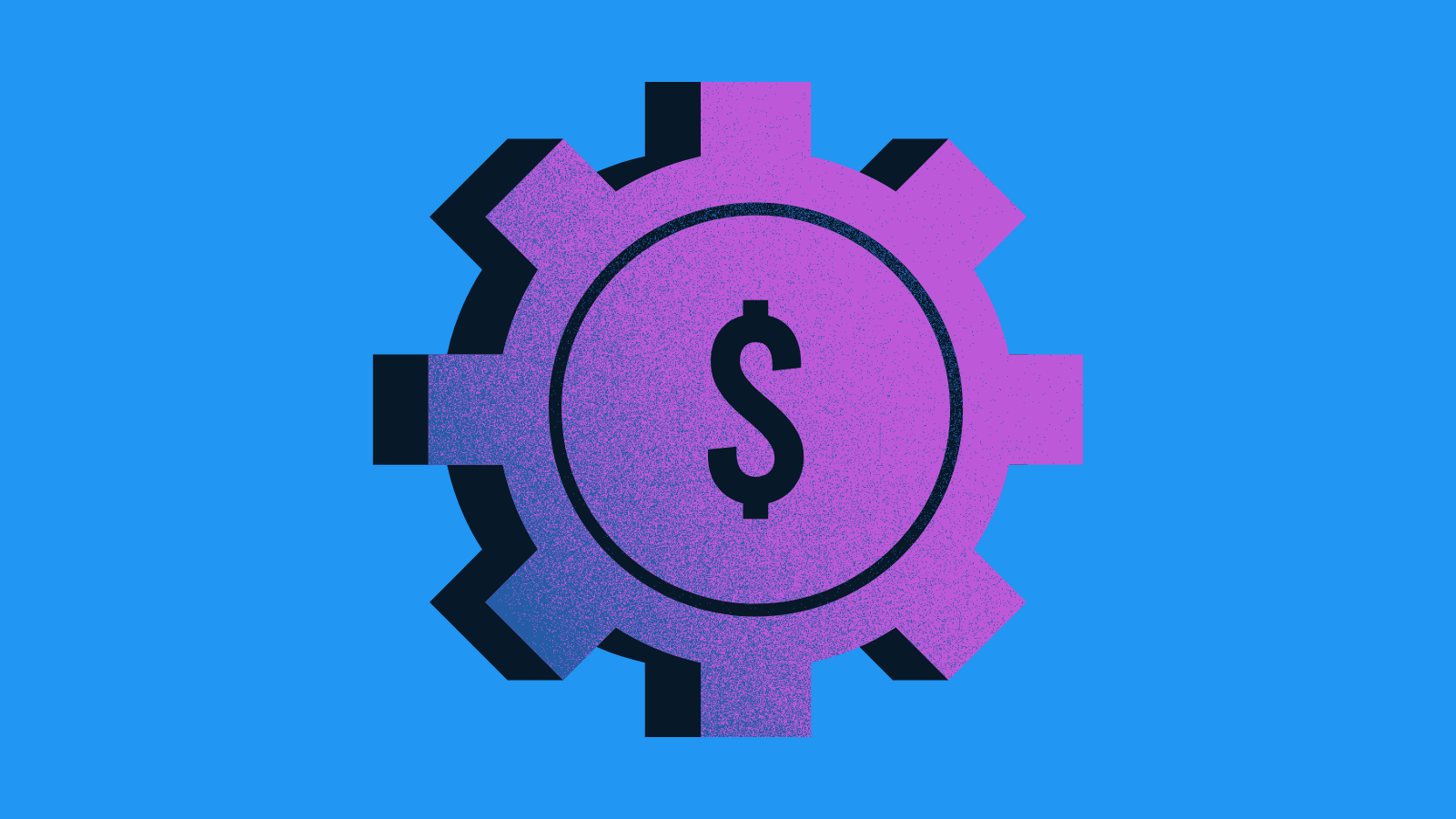
Tenet Healthcare Corporation (THC) Stock Price Forecasting Model
This model utilizes a suite of machine learning algorithms to forecast the future price movements of Tenet Healthcare Corporation (THC) common stock. The model incorporates a comprehensive dataset encompassing historical stock performance, macroeconomic indicators (e.g., GDP growth, inflation rates), industry-specific data (e.g., hospital occupancy rates, physician compensation trends), and news sentiment analysis. We employed several preprocessing steps, including data cleaning, feature engineering, and normalization to ensure the model's accuracy. Crucially, we incorporated factors directly related to Tenet's business operations and the healthcare sector as independent variables. This approach aims to provide a more nuanced and reliable prediction compared to simpler models relying solely on historical stock prices. Rigorous backtesting and validation were integral components of this development process. Key performance indicators were meticulously tracked and documented for each iteration of the model to assess the efficacy of various algorithm implementations.
The selected machine learning model is a gradient boosting algorithm, specifically XGBoost. This choice is justified by its demonstrated ability to handle complex non-linear relationships within the dataset, a crucial consideration for predicting stock prices. The model's architecture leverages an ensemble learning approach, combining predictions from multiple decision trees to improve accuracy and robustness. We fine-tuned model hyperparameters through a systematic grid search process, optimizing its performance for this specific dataset and forecasting task. Feature importance analysis was conducted to identify the most influential factors impacting THC stock price movements, offering crucial insights into the market's perspective on the company's performance. These insights can be used for proactive strategic planning and decision-making, potentially leading to enhanced profitability and risk management within Tenet's investment portfolio.
The model's output consists of a predicted price trajectory for THC stock, along with associated confidence intervals. This output will be presented in a clear and accessible format, encompassing both short-term and long-term forecasts. Important caveats include the inherent volatility of the stock market, the limitations of any predictive model, and the evolving nature of the healthcare industry. Transparency in model inputs and assumptions will be paramount. Regular monitoring and retraining of the model will be essential to maintain its accuracy and responsiveness to changes in the market conditions affecting the healthcare sector. The team is prepared to conduct ongoing evaluation and refinement to ensure the model's continued usefulness for stakeholders. Furthermore, the model's output should be considered as a tool to aid decision-making rather than a definitive forecast, and should not replace sound financial analysis.
ML Model Testing
n:Time series to forecast
p:Price signals of Tenet Healthcare stock
j:Nash equilibria (Neural Network)
k:Dominated move of Tenet Healthcare stock holders
a:Best response for Tenet Healthcare target price
For further technical information as per how our model work we invite you to visit the article below:
How do KappaSignal algorithms actually work?
Tenet Healthcare Stock Forecast (Buy or Sell) Strategic Interaction Table
Strategic Interaction Table Legend:
X axis: *Likelihood% (The higher the percentage value, the more likely the event will occur.)
Y axis: *Potential Impact% (The higher the percentage value, the more likely the price will deviate.)
Z axis (Grey to Black): *Technical Analysis%
Rating | Short-Term | Long-Term Senior |
---|---|---|
Outlook | B3 | B1 |
Income Statement | B1 | Caa2 |
Balance Sheet | C | Caa2 |
Leverage Ratios | Caa2 | Baa2 |
Cash Flow | Ba2 | Baa2 |
Rates of Return and Profitability | C | C |
*Financial analysis is the process of evaluating a company's financial performance and position by neural network. It involves reviewing the company's financial statements, including the balance sheet, income statement, and cash flow statement, as well as other financial reports and documents.
How does neural network examine financial reports and understand financial state of the company?
References
- T. Morimura, M. Sugiyama, M. Kashima, H. Hachiya, and T. Tanaka. Nonparametric return distribution ap- proximation for reinforcement learning. In Proceedings of the 27th International Conference on Machine Learning, pages 799–806, 2010
- Efron B, Hastie T. 2016. Computer Age Statistical Inference, Vol. 5. Cambridge, UK: Cambridge Univ. Press
- A. Eck, L. Soh, S. Devlin, and D. Kudenko. Potential-based reward shaping for finite horizon online POMDP planning. Autonomous Agents and Multi-Agent Systems, 30(3):403–445, 2016
- Artis, M. J. W. Zhang (1990), "BVAR forecasts for the G-7," International Journal of Forecasting, 6, 349–362.
- Barrett, C. B. (1997), "Heteroscedastic price forecasting for food security management in developing countries," Oxford Development Studies, 25, 225–236.
- J. G. Schneider, W. Wong, A. W. Moore, and M. A. Riedmiller. Distributed value functions. In Proceedings of the Sixteenth International Conference on Machine Learning (ICML 1999), Bled, Slovenia, June 27 - 30, 1999, pages 371–378, 1999.
- A. Tamar and S. Mannor. Variance adjusted actor critic algorithms. arXiv preprint arXiv:1310.3697, 2013.