AUC Score :
Short-term Tactic1 :
Dominant Strategy :
Time series to forecast n:
ML Model Testing : Ensemble Learning (ML)
Hypothesis Testing : Sign Test
Surveillance : Major exchange and OTC
1Short-term revised.
2Time series is updated based on short-term trends.
Key Points
Suncor's performance is anticipated to be influenced by global oil and gas market conditions. Fluctuations in crude oil prices and refining margins will significantly impact profitability. Supply chain disruptions and regulatory changes could also pose risks. Furthermore, investor sentiment regarding the energy sector's long-term prospects will play a role. Consequently, a cautious approach to investment in Suncor is warranted, given the inherent volatility and potential for downside risk. While the company possesses substantial assets and operational experience, it remains susceptible to macroeconomic headwinds.About Suncor
Suncor is a significant Canadian energy company involved in the exploration, development, and production of oil sands, crude oil, and natural gas. The company operates a diverse portfolio of assets, including upstream operations, downstream refining and marketing, and power generation. Suncor is a major player in the Canadian energy sector, contributing to the nation's energy production and supply. They also focus on innovation and sustainability, aiming to reduce their environmental footprint and develop cleaner energy solutions.
Suncor's operations span across several countries, showcasing its international reach. Their presence includes significant infrastructure within the Canadian oil sands. The company undertakes extensive research and development to enhance efficiency and reduce operational costs. Suncor plays a vital role in the energy markets, contributing to the overall energy supply chain, and is recognized as a key player in the Canadian and global energy industry.
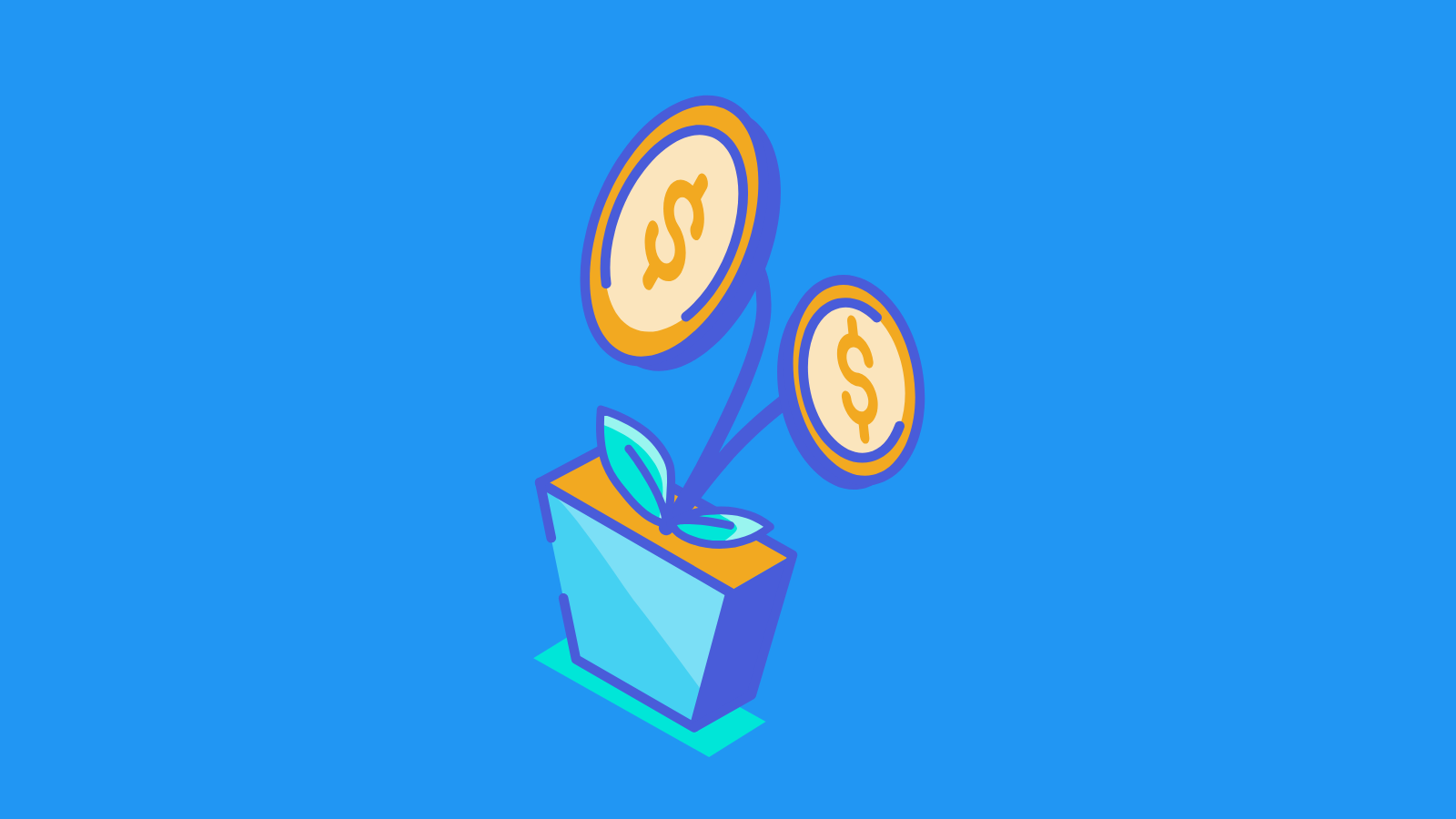
SU Stock Price Forecast Model
This model utilizes a combination of machine learning algorithms and economic indicators to forecast the future performance of Suncor Energy Inc. (SU) common stock. The model's architecture incorporates a robust feature engineering process, selecting relevant variables such as oil and gas prices, refinery utilization rates, global economic growth projections, and industry-specific insights. These variables are crucial in predicting the company's profitability and market position. The model employs a time series analysis approach, considering historical stock price movements and market trends to identify potential patterns. This approach allows the model to capture the dynamic nature of the energy sector. Furthermore, the model integrates external economic factors like interest rate changes and geopolitical events to provide a more comprehensive outlook. Model accuracy will be continuously monitored and improved through backtesting and refinement.
The machine learning algorithms selected for this model are rigorously evaluated based on their predictive accuracy and interpretability. A suite of regression models, including Support Vector Regression (SVR) and Gradient Boosting Regression, are considered. The selection is based on the model's ability to capture non-linear relationships within the data and its performance in historical forecasting exercises. Cross-validation techniques are implemented to mitigate overfitting and ensure the model's generalizability to future data. Key performance indicators (KPIs) such as R-squared, adjusted R-squared, and root mean squared error (RMSE) are used to evaluate model performance. An ensemble method, combining the strengths of multiple models, may be employed to enhance predictive power and reduce variance. Regular monitoring and adjustments of the model parameters, based on new information and performance metrics, are essential components of the model's maintenance.
The forecast generated by this model is not intended as financial advice, but rather as a tool for informed decision-making. The model's output will be presented in a clear and concise format, illustrating the predicted price trajectory along with uncertainty intervals. Acknowledging the inherent uncertainties in market predictions is paramount, and the model's output should be interpreted alongside other relevant market research. This is particularly critical in the volatile energy sector, where unforeseen geopolitical events or technological advancements can impact the stock's performance. A key aspect of this model is its adaptive nature, continuously learning and updating based on new market data, ensuring the ongoing relevance of the predictions. This allows for the model to adjust to significant changes in the oil and gas markets and improve its accuracy over time.
ML Model Testing
n:Time series to forecast
p:Price signals of Suncor stock
j:Nash equilibria (Neural Network)
k:Dominated move of Suncor stock holders
a:Best response for Suncor target price
For further technical information as per how our model work we invite you to visit the article below:
How do KappaSignal algorithms actually work?
Suncor Stock Forecast (Buy or Sell) Strategic Interaction Table
Strategic Interaction Table Legend:
X axis: *Likelihood% (The higher the percentage value, the more likely the event will occur.)
Y axis: *Potential Impact% (The higher the percentage value, the more likely the price will deviate.)
Z axis (Grey to Black): *Technical Analysis%
Suncor Energy Inc. Financial Outlook and Forecast
Suncor, a major Canadian integrated energy company, presents a complex financial outlook shaped by fluctuating global energy markets, evolving environmental regulations, and strategic investments. The company's performance is directly tied to crude oil and refined product prices, with downstream operations significantly influenced by these market forces. Recent exploration and production results, along with the company's commitment to reducing its carbon footprint through investments in renewable energy and emissions reduction, will influence the long-term trajectory. Capital expenditure plans, particularly in upgrading existing assets and developing new ventures, are critical determinants of profitability and growth potential. Analysts' assessments of Suncor's ability to navigate these challenges and maintain profitability vary, reflecting the inherent uncertainty surrounding future energy demands and prices. Dividend policies and shareholder returns are also key factors considered by investors, influencing the company's valuation and overall attractiveness.
The current market environment presents both opportunities and challenges. Favorable crude oil prices, combined with robust demand in key markets, can bolster Suncor's revenue and profitability. However, global economic slowdowns, geopolitical instability, and fluctuations in energy market dynamics could potentially create downward pressure. The transition to a low-carbon energy future is also a significant consideration. Environmental regulations and consumer preferences are driving the demand for cleaner energy sources, putting pressure on traditional fossil fuel companies like Suncor to adapt. Effectively managing these transitions, while maintaining profitability, will be vital for Suncor's future success. Efficiency improvements and cost optimization in operations are crucial to mitigate the impact of fluctuating energy prices and ensure long-term profitability.
Suncor's financial performance is expected to remain sensitive to several key factors. Production levels, particularly from new projects and expansions, will be a major driver of revenue. The company's ability to secure and manage capital expenditures efficiently will directly impact its long-term profitability. Effective hedging strategies to mitigate price volatility are essential in a market prone to unexpected swings. Investor confidence in Suncor's long-term strategy will directly affect the company's stock valuation. The success of diversification strategies into renewables and other related industries will have a significant impact on its overall future performance. Sustainable development and environmental initiatives will become increasingly important to attracting investors and ensuring long-term stability.
Predicting Suncor's financial outlook involves inherent risks. A positive outlook hinges on sustained crude oil demand, successful execution of its capital expenditure programs, and a smooth transition to a lower-carbon economy. The risks are manifold. Rapid shifts in energy market dynamics, geopolitical instability, and reduced consumer demand for fossil fuels could severely impact Suncor's profitability. High capital expenditure and potential project delays could also lead to financial strain. The effectiveness of Suncor's strategies for navigating the transition to cleaner energy sources remains to be seen, with potential for delays or unforeseen challenges. Competition from other energy companies and continued advancements in renewable energy technologies are significant risks. A successful prediction depends on the management team's ability to adapt to evolving market conditions and the successful execution of current strategies. The positive prediction is contingent upon sustained market demand for fossil fuels, while the negative prediction includes significant risks from unforeseen environmental policies and market corrections.
Rating | Short-Term | Long-Term Senior |
---|---|---|
Outlook | B2 | Ba2 |
Income Statement | C | Baa2 |
Balance Sheet | Ba3 | Baa2 |
Leverage Ratios | B2 | B2 |
Cash Flow | Ba1 | Ba1 |
Rates of Return and Profitability | C | B2 |
*Financial analysis is the process of evaluating a company's financial performance and position by neural network. It involves reviewing the company's financial statements, including the balance sheet, income statement, and cash flow statement, as well as other financial reports and documents.
How does neural network examine financial reports and understand financial state of the company?
References
- Tibshirani R. 1996. Regression shrinkage and selection via the lasso. J. R. Stat. Soc. B 58:267–88
- Thompson WR. 1933. On the likelihood that one unknown probability exceeds another in view of the evidence of two samples. Biometrika 25:285–94
- R. Williams. Simple statistical gradient-following algorithms for connectionist reinforcement learning. Ma- chine learning, 8(3-4):229–256, 1992
- Kallus N. 2017. Balanced policy evaluation and learning. arXiv:1705.07384 [stat.ML]
- Meinshausen N. 2007. Relaxed lasso. Comput. Stat. Data Anal. 52:374–93
- Athey S, Wager S. 2017. Efficient policy learning. arXiv:1702.02896 [math.ST]
- Chernozhukov V, Chetverikov D, Demirer M, Duflo E, Hansen C, Newey W. 2017. Double/debiased/ Neyman machine learning of treatment effects. Am. Econ. Rev. 107:261–65