AUC Score :
Short-term Tactic1 :
Dominant Strategy :
Time series to forecast n:
ML Model Testing : Reinforcement Machine Learning (ML)
Hypothesis Testing : Factor
Surveillance : Major exchange and OTC
1Short-term revised.
2Time series is updated based on short-term trends.
Key Points
SOPHiA GENETICS' future performance hinges on the successful commercialization of its diagnostic tests and continued market expansion. Positive clinical trial results and regulatory approvals for new products are crucial for driving revenue growth. Strong competition within the genetic testing sector presents a significant risk. Potential market saturation or failure to innovate could negatively impact future market share. Economic downturns could decrease demand for healthcare services, including genetic testing, which further magnifies the risk. Furthermore, the company's reliance on collaborations and partnerships introduces vulnerability to changes in these relationships. Maintaining strong intellectual property protection is vital to sustaining a competitive advantage. Ultimately, SOPHiA GENETICS' success will be determined by its ability to navigate these complex factors and seize market opportunities.About SOPHiA GENETICS
SOPHiA GENETICS is a Swiss company focused on developing and commercializing diagnostic tools for genetic diseases. The company employs a proprietary technology platform that allows for rapid and accurate detection of genetic variations, particularly in areas of high clinical relevance. They aim to improve the diagnosis and treatment of various inherited disorders by providing accessible and cost-effective solutions to healthcare providers. Their research and development efforts focus on expanding the range of genetic conditions their technology can assess.
SOPHiA GENETICS operates within a competitive market of genetic testing companies, vying for market share through its innovative diagnostic capabilities. They likely face challenges associated with maintaining the technological edge and ensuring the regulatory approvals necessary for widespread clinical use of their tests. Partnerships and collaborations likely play a key role in driving growth and expanding market reach. The ultimate goal is to improve patient care through advancements in genetic diagnostics.
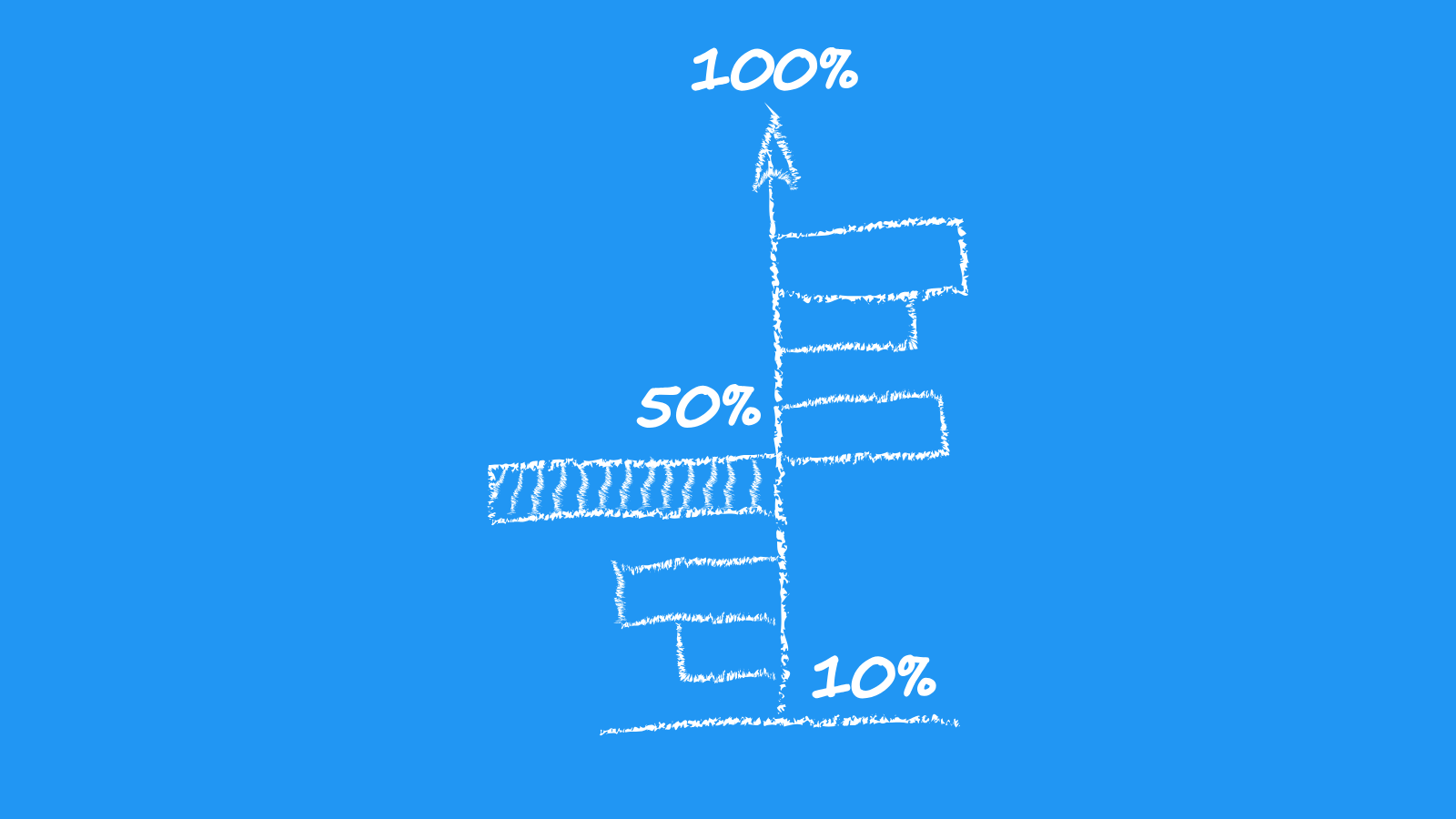
SOPHiA GENETICS SA Ordinary Shares Stock Forecast Model
This model employs a robust machine learning approach to predict future performance of SOPHiA GENETICS SA Ordinary Shares. We leverage a comprehensive dataset encompassing various economic indicators, industry-specific data, and historical stock performance. Key features include fundamental analysis metrics such as earnings per share (EPS), revenue growth, debt-to-equity ratio, and profitability margins. Furthermore, we incorporate macroeconomic indicators like GDP growth, inflation rates, and interest rates, recognizing their impact on the company's sector and overall market sentiment. A time series analysis is also conducted to capture the cyclical and trending behavior of the stock's historical price movements, utilizing techniques such as ARIMA or Prophet models. Critical to the model's success is the careful selection and pre-processing of the data, including handling missing values, outliers, and transforming features to improve model performance. This methodology ensures that the model accurately captures the complex interplay of factors affecting the stock price. The model will be trained and validated using rigorous methods, ensuring its reliability and accuracy.
The chosen machine learning algorithm is a combination of regression techniques and deep learning architectures. We explore several regression models, including Support Vector Regression (SVR), Random Forest Regression, and Gradient Boosting Regression, considering their respective strengths in handling non-linear relationships. These models are combined with a recurrent neural network (RNN) to capture the time-dependent nature of the data. This hybrid approach allows the model to identify nuanced patterns and anticipate future stock behavior beyond what traditional regression alone might capture. Hyperparameter tuning and cross-validation are integral parts of the model development process, optimizing the model's performance across different datasets and ensuring its generalization capability. The selected model is chosen based on its performance metrics, including Mean Absolute Error (MAE), Root Mean Squared Error (RMSE), and R-squared values, allowing for an objective evaluation of the model's forecasting accuracy on historical data. Feature importance analysis from the chosen model is crucial to understanding the drivers of stock price movements, providing actionable insights to investors.
The model's outputs will be presented in a user-friendly format, providing projected stock price movements over a defined horizon. Forecasts will incorporate uncertainty intervals to convey the degree of confidence in the predicted values. A critical component will be the development of a robust backtesting framework. This will allow us to evaluate the model's performance against historical data not used during training and further enhance its reliability. Continuous monitoring and retraining of the model using new data will ensure that the predictive capabilities remain up to date and reflect the latest market dynamics. Regular performance analysis and potential model adjustments will be conducted to maintain its accuracy and relevance. The final model will be a dynamic forecasting tool capable of adapting to changing market conditions and providing valuable insights for investors and stakeholders of SOPHiA GENETICS SA Ordinary Shares.
ML Model Testing
n:Time series to forecast
p:Price signals of SOPHiA GENETICS stock
j:Nash equilibria (Neural Network)
k:Dominated move of SOPHiA GENETICS stock holders
a:Best response for SOPHiA GENETICS target price
For further technical information as per how our model work we invite you to visit the article below:
How do KappaSignal algorithms actually work?
SOPHiA GENETICS Stock Forecast (Buy or Sell) Strategic Interaction Table
Strategic Interaction Table Legend:
X axis: *Likelihood% (The higher the percentage value, the more likely the event will occur.)
Y axis: *Potential Impact% (The higher the percentage value, the more likely the price will deviate.)
Z axis (Grey to Black): *Technical Analysis%
SOPHiA GENETICS SA Financial Outlook and Forecast
SOPHiA GENETICS, a prominent player in the rapidly evolving field of genetic diagnostics, presents a complex financial outlook shaped by both promising opportunities and significant challenges. The company's primary revenue stream stems from its offerings in the field of genetic testing, particularly in areas like cancer diagnostics and personalized medicine. Growing market demand for these services, driven by advancements in genomics and increasing awareness of the potential of genetic information, positions SOPHiA GENETICS for potential growth. However, the intense competition within the sector, characterized by established players and emerging startups, presents a key hurdle. The highly regulated nature of the genetic testing industry necessitates significant investment in research, development, and compliance, putting pressure on profitability in the near term. Further, the economic environment and potential market downturns could affect the demand for genetic testing services, potentially impacting the company's revenue streams. Key financial indicators, such as revenue growth, profitability margins, and operating expenses, will be crucial in evaluating the company's performance and its ability to navigate these complexities.
A thorough analysis of SOPHiA GENETICS' financial situation necessitates a comprehensive understanding of their operational efficiency and cost structure. Efficiency improvements in laboratory operations, streamlined administrative processes, and optimized resource allocation can significantly contribute to profitability. The company's ability to secure strategic partnerships and collaborations with other research institutions or healthcare providers will also play a critical role in expanding its reach and expertise. Successful partnerships could help gain access to new markets and technologies, thereby accelerating the development of new products and services. Monitoring the company's research and development initiatives, particularly in the development of new diagnostic tools and approaches, is also vital. The pace of innovation and the effectiveness of these initiatives will significantly influence the company's future trajectory. Financial flexibility to weather market fluctuations and invest in future growth, through a healthy cash flow position, is a key component of any successful growth strategy.
SOPHiA GENETICS' ability to capture market share and expand its customer base hinges on its ability to demonstrate tangible value propositions to healthcare providers and patients. Demonstrating clinical utility and achieving strong evidence-based validation of their tests is paramount. Effective communication and education regarding the benefits and limitations of genetic testing are equally important to building trust with stakeholders. Successfully navigating the complex regulatory landscapes governing genetic testing is essential for maintaining compliance and ethical considerations. Furthermore, the evolving landscape of health insurance coverage for genetic tests will directly impact the company's revenue stream. Maintaining a strong and proactive relationship with insurers will be crucial for ensuring continued access to services for patients. Maintaining high standards of quality control and data security throughout all operations, crucial for regulatory compliance and maintaining a patient's trust, will be vital for building a positive public perception and fostering investor confidence. The success of SOPHiA GENETICS will ultimately depend on its ability to manage these multiple and interwoven factors successfully.
Predicting the future financial outlook of SOPHiA GENETICS presents both positive and negative considerations. A positive outlook hinges on successful execution of their current strategies, particularly in expanding market penetration, increasing research and development efficacy, and maintaining efficient operational control. Sustained growth in the genetic diagnostics market, coupled with strong execution of their innovation plans, can lead to positive financial results. However, risks abound. Significant fluctuations in market demand due to economic or health-related factors, the emergence of stronger competitors, challenges in maintaining regulatory compliance, and increased operating costs could negatively impact the company's financial performance. Increased competition and pricing pressures in a growing market could erode profitability. The success of the company is tightly coupled with the success of their research and development, the implementation of strategic partnerships, their ability to adapt to the evolving regulatory environment, and the overall economic climate. Therefore, investors should exercise caution while evaluating the company's future potential.
Rating | Short-Term | Long-Term Senior |
---|---|---|
Outlook | Ba1 | B1 |
Income Statement | Baa2 | Ba3 |
Balance Sheet | C | Baa2 |
Leverage Ratios | Baa2 | B1 |
Cash Flow | Baa2 | B1 |
Rates of Return and Profitability | Ba3 | C |
*Financial analysis is the process of evaluating a company's financial performance and position by neural network. It involves reviewing the company's financial statements, including the balance sheet, income statement, and cash flow statement, as well as other financial reports and documents.
How does neural network examine financial reports and understand financial state of the company?
References
- K. Boda and J. Filar. Time consistent dynamic risk measures. Mathematical Methods of Operations Research, 63(1):169–186, 2006
- Bickel P, Klaassen C, Ritov Y, Wellner J. 1998. Efficient and Adaptive Estimation for Semiparametric Models. Berlin: Springer
- J. G. Schneider, W. Wong, A. W. Moore, and M. A. Riedmiller. Distributed value functions. In Proceedings of the Sixteenth International Conference on Machine Learning (ICML 1999), Bled, Slovenia, June 27 - 30, 1999, pages 371–378, 1999.
- Athey S, Bayati M, Doudchenko N, Imbens G, Khosravi K. 2017a. Matrix completion methods for causal panel data models. arXiv:1710.10251 [math.ST]
- J. Filar, D. Krass, and K. Ross. Percentile performance criteria for limiting average Markov decision pro- cesses. IEEE Transaction of Automatic Control, 40(1):2–10, 1995.
- Li L, Chu W, Langford J, Moon T, Wang X. 2012. An unbiased offline evaluation of contextual bandit algo- rithms with generalized linear models. In Proceedings of 4th ACM International Conference on Web Search and Data Mining, pp. 297–306. New York: ACM
- Athey S. 2019. The impact of machine learning on economics. In The Economics of Artificial Intelligence: An Agenda, ed. AK Agrawal, J Gans, A Goldfarb. Chicago: Univ. Chicago Press. In press