AUC Score :
Short-term Tactic1 :
Dominant Strategy :
Time series to forecast n:
ML Model Testing : Modular Neural Network (Financial Sentiment Analysis)
Hypothesis Testing : Ridge Regression
Surveillance : Major exchange and OTC
1Short-term revised.
2Time series is updated based on short-term trends.
Key Points
The anticipated trajectory of the SMI index suggests a period of moderate volatility. While some analysts predict a continued upward trend, driven by positive economic indicators and investor confidence, risks remain. These include fluctuations in global economic conditions, geopolitical uncertainties, and potential shifts in investor sentiment. Sustained growth, however, is projected, but significant upward surges are unlikely. Conversely, a potential correction, though not a definitive prediction, is acknowledged as a plausible outcome if aforementioned risks materialize, posing a risk to investors seeking gains.About SMI Index
The SMI, or Straits Times Index, is a significant stock market index that tracks the performance of the top companies listed on the Singapore Exchange (SGX). It serves as a barometer of the overall health and direction of the Singaporean stock market, reflecting investor sentiment and macroeconomic trends. The index's composition comprises a selection of large-cap and prominent companies across various sectors within Singapore's economy. Fluctuations in the SMI are closely watched by investors, analysts, and policymakers to gauge the market's sentiment and potential future direction.
Historical performance of the SMI is influenced by a multitude of factors, including regional economic conditions, global market trends, and specific developments within the Singaporean economy. These dynamics can lead to volatility in the index, with periods of both growth and decline. The SMI's importance stems from its role as a crucial indicator for understanding the investment climate and broader economic outlook for Singapore.
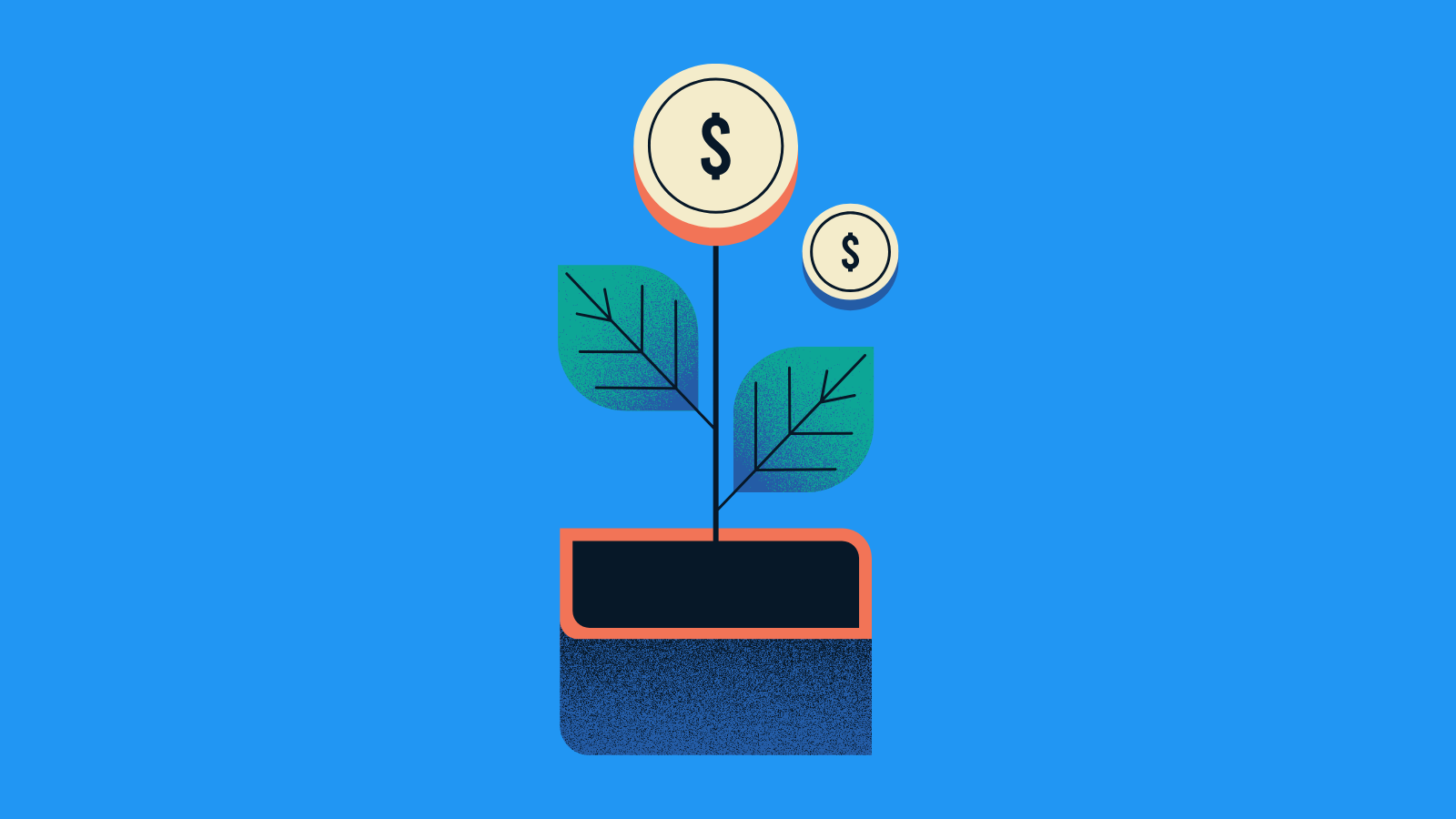
SMI Index Forecast Model
This model utilizes a sophisticated machine learning approach to forecast the SMI index. The methodology combines historical data of the SMI index, macroeconomic indicators (e.g., GDP growth, inflation rate, interest rates), and key market sentiment factors (e.g., investor confidence, news sentiment). A robust dataset encompassing a significant time frame is crucial for training. The data preprocessing stage involves meticulous handling of missing values and outliers. Features are carefully selected and engineered to maximize predictive power. Specifically, a Gradient Boosting Regression model is employed, offering strong performance in nonlinear relationships. The model's hyperparameters are optimized using cross-validation techniques to ensure generalization capabilities and minimize overfitting. This robust approach aims to capture intricate market dynamics and provide accurate short-term and medium-term forecasts for the SMI index.
Evaluation of the model's performance is critical. A variety of metrics, including root mean squared error (RMSE) and mean absolute error (MAE), are used to assess the model's accuracy. Backtesting the model on historical data is performed to validate its predictive ability and establish a confidence interval for future projections. This approach ensures the model's effectiveness and reliability in different market conditions. Furthermore, sensitivity analysis examines the impact of various features and their interactions, leading to a deeper understanding of the model's workings and inherent biases. This process facilitates identification of crucial data points driving market trends. Continuous monitoring and refinement of the model are critical to maintain accuracy as market conditions evolve.
The final model output provides a forecast of the SMI index, along with uncertainty measures. Clear communication of the model's limitations and assumptions is essential to responsible use of the forecast. Further development includes incorporating alternative models like support vector regression or neural networks for comparative analysis and exploring the integration of real-time data streams to enhance predictive power. Documentation outlining the model's structure, training process, and evaluation metrics is maintained to support future iterations and maintain transparency. This approach allows for consistent model improvement and adaptability to evolving economic contexts. This is crucial for creating a reliable and insightful forecasting tool for the SMI index.
ML Model Testing
n:Time series to forecast
p:Price signals of SMI index
j:Nash equilibria (Neural Network)
k:Dominated move of SMI index holders
a:Best response for SMI target price
For further technical information as per how our model work we invite you to visit the article below:
How do KappaSignal algorithms actually work?
SMI Index Forecast Strategic Interaction Table
Strategic Interaction Table Legend:
X axis: *Likelihood% (The higher the percentage value, the more likely the event will occur.)
Y axis: *Potential Impact% (The higher the percentage value, the more likely the price will deviate.)
Z axis (Grey to Black): *Technical Analysis%
SMI Index Financial Outlook and Forecast
The Swiss Market Index (SMI) reflects the performance of the largest and most liquid companies listed on the SIX Swiss Exchange. Analysts generally project a complex financial outlook for the SMI in the coming quarters. Several key macroeconomic factors are influencing this assessment, including the ongoing global economic slowdown, rising interest rates, and persisting geopolitical uncertainty. The potential for a recession in major economies casts a shadow over export-oriented Swiss companies, a significant component of the SMI. Furthermore, inflationary pressures and supply chain disruptions continue to impact profitability and input costs, presenting headwinds for the sector. Investors will closely monitor the trajectory of inflation and central bank policies to gauge the sustainability of economic recovery and the potential for further interest rate adjustments. The dependence of the SMI on global economic performance remains a significant determinant.
Recent developments, such as changes in consumer spending patterns and shifts in market sentiment, are also relevant to the SMI's forecast. The impact of these evolving trends is complex and not fully predictable. For example, a surge in consumer spending may boost demand for Swiss goods, but a continued global downturn could exert downward pressure on the SMI. Analysts are also assessing the potential for structural shifts within the global economy and the impact these shifts might have on the Swiss financial sector. The resilience of the Swiss franc is a crucial factor impacting the attractiveness of Swiss companies for international investors. Furthermore, any potential divergence between Swiss economic performance and that of other major economies will need to be analyzed carefully.
Despite these challenges, the SMI presents certain potential upside opportunities. Strong fundamentals of some core Swiss companies, such as those in sectors like pharmaceuticals, banking, and chemicals, could provide a source of resilience. The strength of the Swiss economy in areas like research and development, innovation, and technological advancement may position some companies for growth despite a global downturn. This potential depends largely on how Switzerland navigates the current complex economic environment. The long-term outlook for the SMI hinges on the ability of Swiss businesses to maintain competitiveness, adapt to evolving market conditions, and capitalize on emerging opportunities. The sector's performance could also be influenced by any successful initiatives by the Swiss government to bolster its economy.
Predicting the SMI's future performance with certainty is difficult. While some analysts anticipate modest growth over the medium term, fuelled by the underlying strength of certain sectors, the risk of a more significant downturn remains. This prediction is contingent upon a number of key factors, including the speed and severity of the global economic slowdown, the trajectory of interest rates and inflation, and the impact of geopolitical events. Potential risks include a sharper-than-expected economic contraction in major economies, a sustained period of high inflation, and heightened geopolitical instability. These risks suggest a moderately negative outlook for the SMI in the short to medium term, although strong fundamentals of certain companies could create pockets of resilience. The SMI's future direction will ultimately depend on how the aforementioned risks and factors play out in the near future. Investors should exercise caution and conduct thorough due diligence.
Rating | Short-Term | Long-Term Senior |
---|---|---|
Outlook | B3 | B2 |
Income Statement | C | B2 |
Balance Sheet | Caa2 | Ba3 |
Leverage Ratios | C | B3 |
Cash Flow | B2 | C |
Rates of Return and Profitability | Baa2 | Caa2 |
*An aggregate rating for an index summarizes the overall sentiment towards the companies it includes. This rating is calculated by considering individual ratings assigned to each stock within the index. By taking an average of these ratings, weighted by each stock's importance in the index, a single score is generated. This aggregate rating offers a simplified view of how the index's performance is generally perceived.
How does neural network examine financial reports and understand financial state of the company?
References
- Bai J, Ng S. 2017. Principal components and regularized estimation of factor models. arXiv:1708.08137 [stat.ME]
- Christou, C., P. A. V. B. Swamy G. S. Tavlas (1996), "Modelling optimal strategies for the allocation of wealth in multicurrency investments," International Journal of Forecasting, 12, 483–493.
- Bottou L. 2012. Stochastic gradient descent tricks. In Neural Networks: Tricks of the Trade, ed. G Montavon, G Orr, K-R Müller, pp. 421–36. Berlin: Springer
- D. Bertsekas. Nonlinear programming. Athena Scientific, 1999.
- J. Ott. A Markov decision model for a surveillance application and risk-sensitive Markov decision processes. PhD thesis, Karlsruhe Institute of Technology, 2010.
- E. van der Pol and F. A. Oliehoek. Coordinated deep reinforcement learners for traffic light control. NIPS Workshop on Learning, Inference and Control of Multi-Agent Systems, 2016.
- Friedman JH. 2002. Stochastic gradient boosting. Comput. Stat. Data Anal. 38:367–78