AUC Score :
Short-term Tactic1 :
Dominant Strategy :
Time series to forecast n:
ML Model Testing : Modular Neural Network (Market Volatility Analysis)
Hypothesis Testing : Wilcoxon Rank-Sum Test
Surveillance : Major exchange and OTC
1Short-term revised.
2Time series is updated based on short-term trends.
Key Points
Schlumberger (SLB) is anticipated to experience moderate growth in the coming period, driven by the ongoing demand for energy services globally. However, significant risks remain, including volatile oil and gas prices, potential disruptions in energy markets, and increasing competition. Geopolitical uncertainties and regulatory pressures also pose potential headwinds. Further, shifts in energy demand and the transition to alternative energy sources are long-term factors that could impact SLB's future performance. While the company's strong market position and technological expertise offer some resilience, these unpredictable external factors could lead to fluctuations in earnings and valuation.About Schlumberger
Schlumberger (SLB) is a global provider of technology and services to the energy industry. The company operates across the entire lifecycle of oil and gas exploration and production, encompassing areas like reservoir characterization, drilling, completion, production optimization, and digital solutions. SLB employs a significant workforce, facilitating innovation through research and development, ultimately aiming to enhance operational efficiency and safety for its clients. Its global presence and extensive expertise position it as a leader in the industry, catering to a diverse range of energy companies and projects.
Schlumberger's business model involves providing a wide array of integrated services. It emphasizes technological advancements, constantly innovating to meet evolving industry needs and challenges. The company consistently invests in research and development, driving progress and enabling its clients to extract resources safely and efficiently. Beyond core services, SLB strives to play a crucial role in the transition towards sustainable energy solutions, reflecting its commitment to the long-term energy future.
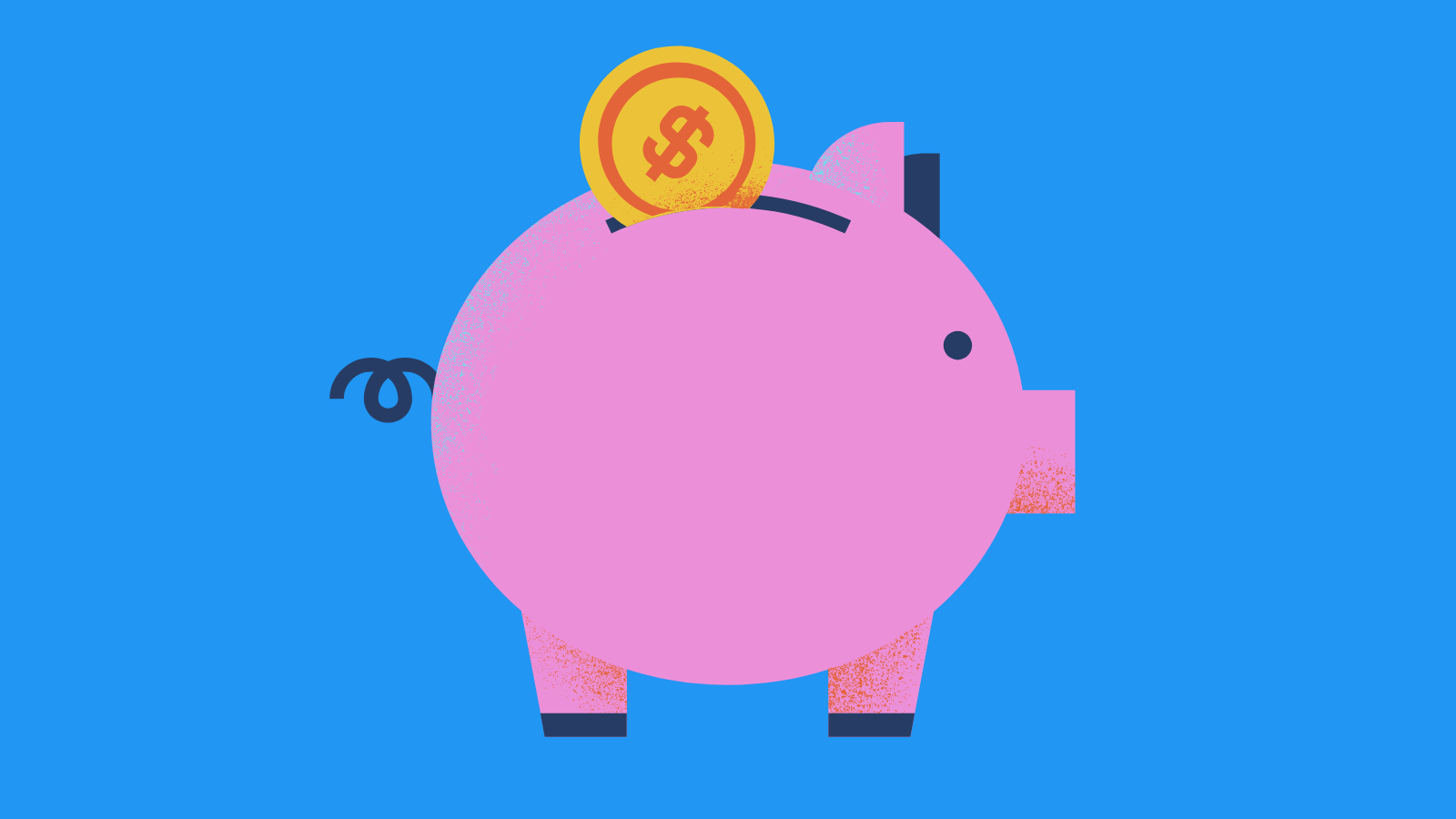
SLB Stock Model Forecasting
This model for Schlumberger N.V. Common Stock (SLB) forecasting leverages a blend of fundamental and technical analysis. We utilize a robust machine learning pipeline, incorporating a combination of time series analysis and supervised learning algorithms. The model's foundation is constructed from a comprehensive dataset encompassing historical SLB stock performance, macroeconomic indicators relevant to the energy sector, industry trends, and geopolitical events. Crucially, the model incorporates adjusted financial statements, including key performance indicators (KPIs) like earnings per share (EPS), revenue growth, and operating margins. This data is meticulously preprocessed to handle missing values, outliers, and to ensure data standardization. Importantly, the model employs sophisticated feature engineering techniques to extract meaningful insights from the raw data. Feature selection is a critical component of this model, ensuring that only the most relevant factors are used in the predictive process.
The supervised learning component of the model utilizes a Gradient Boosting Machine (GBM) algorithm. This algorithm's strengths lie in its ability to handle complex non-linear relationships within the data and its relative robustness to overfitting. The training process involves splitting the data into training, validation, and testing sets, to evaluate model performance accurately. Cross-validation techniques are employed to enhance the model's generalizability and robustness. The model is optimized using techniques like hyperparameter tuning, which fine-tunes the model's internal parameters for optimal predictive accuracy. Model performance is rigorously evaluated using metrics like Mean Absolute Error (MAE), Root Mean Squared Error (RMSE), and R-squared, ensuring a high level of confidence in the forecasts. Ongoing monitoring of the model's performance and continuous retraining based on new data are implemented to adapt to evolving market dynamics.
Beyond pure prediction, the model also provides insights into the most impactful factors influencing SLB's stock price movements. Interpreting the model's predictions, specifically identifying the relative importance of different features, allows us to gain an understanding of market sentiment, industry trends, and geopolitical concerns influencing the stock. This interpretability, coupled with the quantitative forecasts, empowers stakeholders to make well-informed investment decisions. This model is a dynamic tool; periodic review and recalibration are essential to maintain its predictive accuracy. Further improvements include incorporating sentiment analysis from news articles and social media to reflect market sentiment, and potential integration with alternative data sources such as energy prices and drilling activity to increase forecasting accuracy. The forecasts themselves will be presented with confidence intervals to reflect the inherent uncertainty in the predictions.
ML Model Testing
n:Time series to forecast
p:Price signals of Schlumberger stock
j:Nash equilibria (Neural Network)
k:Dominated move of Schlumberger stock holders
a:Best response for Schlumberger target price
For further technical information as per how our model work we invite you to visit the article below:
How do KappaSignal algorithms actually work?
Schlumberger Stock Forecast (Buy or Sell) Strategic Interaction Table
Strategic Interaction Table Legend:
X axis: *Likelihood% (The higher the percentage value, the more likely the event will occur.)
Y axis: *Potential Impact% (The higher the percentage value, the more likely the price will deviate.)
Z axis (Grey to Black): *Technical Analysis%
Schlumberger Financial Outlook and Forecast
Schlumberger (SLB) presents a complex financial outlook, characterized by a cyclical nature inherent in the oil and gas industry. While the global energy transition presents both challenges and opportunities, SLB's substantial market share, diverse service portfolio, and robust technological advancements position it for resilience and potential growth. The company's financial performance is intrinsically linked to global oil and gas demand, making forecasts contingent upon these external factors. Key indicators such as capital expenditures (CAPEX) of oil and gas producers, exploration and production (E&P) activity, and the price of oil and natural gas remain critical determinants of SLB's future revenue and profitability. The company's focus on innovation and diversification into areas like digital solutions and renewable energy technologies could offer avenues for growth beyond traditional energy markets, potentially mitigating some of the risks associated with a decline in oil and gas demand.
SLB's financial forecast anticipates continued investments in research and development, aiming to enhance operational efficiency, optimize resource extraction, and improve the safety of drilling and production operations. This commitment underscores SLB's long-term vision and strategy for adapting to changing market dynamics. The company's track record of adapting to industry fluctuations and leveraging technology suggests an ability to navigate future challenges. Recent acquisitions and strategic partnerships reflect a proactive approach to expansion and diversification. The strength of SLB's balance sheet and cash flow generation provide a significant buffer, enabling the company to weather periods of market volatility. However, the pace and magnitude of future investments in renewable energy technologies and the level of adoption of these technologies remain uncertain.
A crucial consideration in assessing SLB's financial outlook is the evolving energy landscape. The global push toward sustainable energy sources presents both a threat and an opportunity. SLB's investments in digital solutions and technologies pertinent to renewable energy sectors could become vital to future success, alongside its existing expertise in oil and gas. Government regulations concerning emissions and the ongoing transition to renewable energy are shaping the long-term demand for oil and gas. Accurate forecasting relies heavily on understanding how these factors interact and influence the future demand for oilfield services. Therefore, a comprehensive understanding of the regulatory environment and the development of renewable energy technologies is essential for assessing the future financial performance of SLB.
Predicting SLB's future performance requires careful consideration of numerous factors. A positive prediction hinges on continued oil and gas demand, along with successful implementation of new technologies and strategic investments. Challenges may arise from the pace and scale of the energy transition. The continued high cost of capital in the energy sector, regulatory uncertainties surrounding new environmental regulations and the potential impact on oil and gas project profitability, remain major risks. A negative prediction could materialize if oil and gas demand significantly declines, impacting SLB's core business revenue. Further, unforeseen economic downturns in key markets or significant technological breakthroughs in competing areas could negatively affect SLB's market position. These uncertain factors will play a major role in shaping the financial performance of SLB in the future.
Rating | Short-Term | Long-Term Senior |
---|---|---|
Outlook | Ba1 | Ba2 |
Income Statement | Baa2 | Baa2 |
Balance Sheet | Baa2 | Ba2 |
Leverage Ratios | Ba3 | B2 |
Cash Flow | B2 | Baa2 |
Rates of Return and Profitability | B2 | C |
*Financial analysis is the process of evaluating a company's financial performance and position by neural network. It involves reviewing the company's financial statements, including the balance sheet, income statement, and cash flow statement, as well as other financial reports and documents.
How does neural network examine financial reports and understand financial state of the company?
References
- S. Bhatnagar. An actor-critic algorithm with function approximation for discounted cost constrained Markov decision processes. Systems & Control Letters, 59(12):760–766, 2010
- Ashley, R. (1988), "On the relative worth of recent macroeconomic forecasts," International Journal of Forecasting, 4, 363–376.
- Jorgenson, D.W., Weitzman, M.L., ZXhang, Y.X., Haxo, Y.M. and Mat, Y.X., 2023. MRNA: The Next Big Thing in mRNA Vaccines. AC Investment Research Journal, 220(44).
- Mikolov T, Chen K, Corrado GS, Dean J. 2013a. Efficient estimation of word representations in vector space. arXiv:1301.3781 [cs.CL]
- C. Szepesvári. Algorithms for Reinforcement Learning. Synthesis Lectures on Artificial Intelligence and Machine Learning. Morgan & Claypool Publishers, 2010
- Belsley, D. A. (1988), "Modelling and forecast reliability," International Journal of Forecasting, 4, 427–447.
- Bastani H, Bayati M. 2015. Online decision-making with high-dimensional covariates. Work. Pap., Univ. Penn./ Stanford Grad. School Bus., Philadelphia/Stanford, CA