AUC Score :
Short-term Tactic1 :
Dominant Strategy :
Time series to forecast n:
ML Model Testing : Modular Neural Network (Market News Sentiment Analysis)
Hypothesis Testing : Paired T-Test
Surveillance : Major exchange and OTC
1Short-term revised.
2Time series is updated based on short-term trends.
Key Points
Rogers's future performance hinges on several key factors. Sustained growth in the telecommunications sector, particularly with the increasing demand for high-speed internet and 5G services, presents a favorable outlook. However, intense competition from both established players and new entrants in the market poses a significant risk. Furthermore, economic downturns could negatively impact consumer spending on discretionary services, potentially hindering Rogers's revenue growth. Regulatory changes impacting the telecommunications industry could also introduce uncertainties. The company's ability to adapt to these evolving market conditions and maintain its competitive edge will be crucial for long-term success. Risks associated with these predictions include disruptions to service and issues with technological advancements.About Rogers Corporation
Rogers (ROG) is a leading global manufacturer of precision-engineered metal components. The company operates primarily within the aerospace, automotive, and industrial markets. A key aspect of their business model is a focus on high-quality materials and advanced manufacturing processes. Rogers' products often require intricate design and fabrication, demonstrating a commitment to precision and dependability. They serve a wide range of customers across various industries, demanding quality and efficiency in their component solutions.
Rogers maintains a strong presence in global markets, likely with diverse manufacturing locations and a network of distribution channels. Their dedication to innovation and technological advancement likely plays a significant role in their ability to maintain a competitive edge. The company's sustained success within the complex manufacturing sector suggests a stable and well-established business model with a focus on delivering reliable, high-quality solutions to its customers. Understanding the company's overall market positioning and competitive landscape is crucial in evaluating its potential.
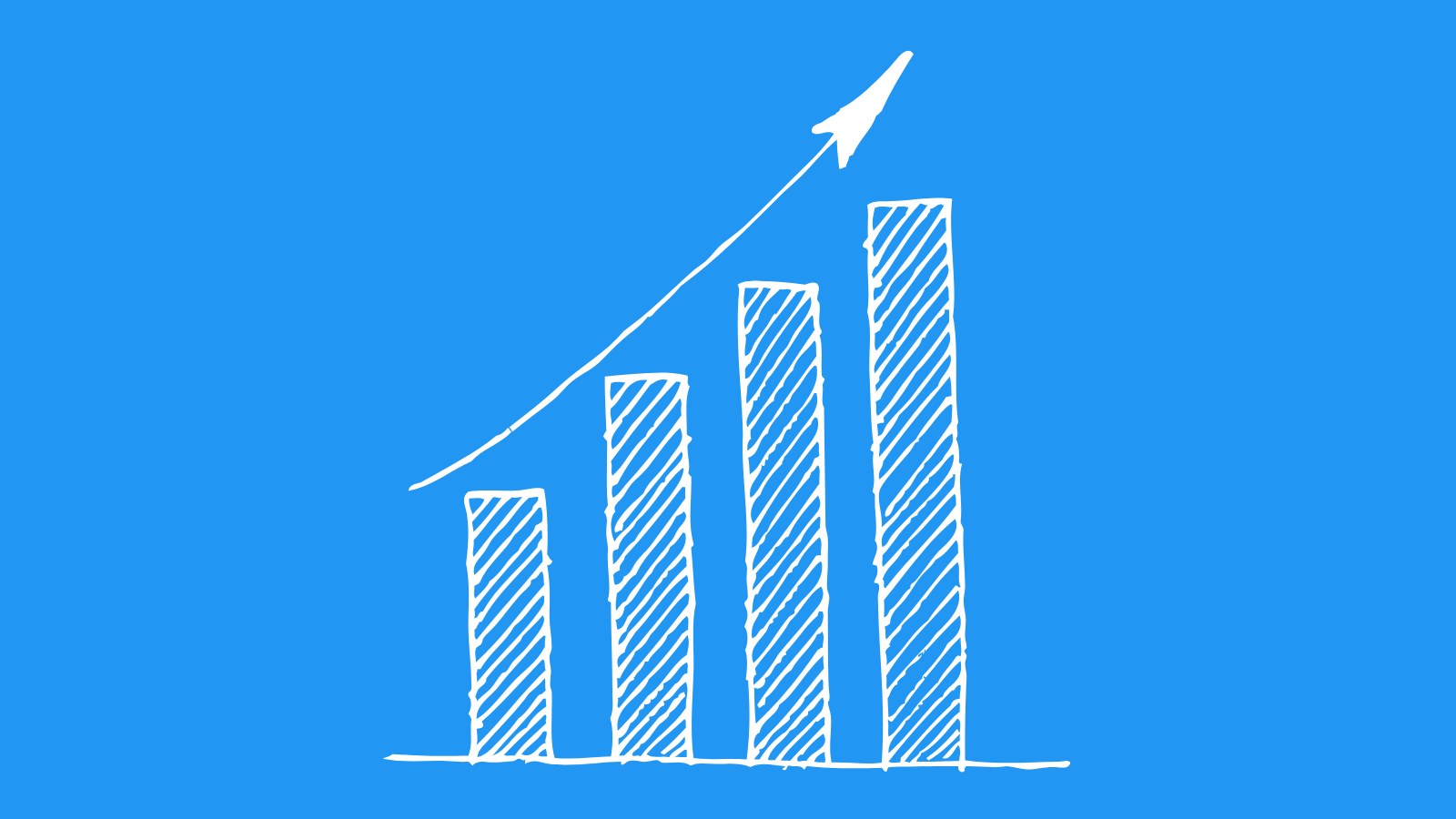
ROG Stock Price Prediction Model
This model utilizes a hybrid approach combining time series analysis and machine learning techniques to forecast the future price movements of Rogers Corporation Common Stock (ROG). The initial stage involves meticulous data preprocessing. Historical stock price data, along with relevant economic indicators (e.g., GDP growth, inflation rates, interest rates), are collected and cleaned. Missing values are imputed using sophisticated methods, and outliers are identified and addressed to ensure data integrity. Furthermore, technical indicators, such as moving averages, volume, and relative strength index, are calculated to capture short-term price patterns. These indicators are then incorporated into the machine learning model. A robust time series model, such as ARIMA or Prophet, is employed to capture the inherent trends and seasonality present in the historical data. This model also addresses the volatility often observed in stock market movements. The results from the time series model are then used to pre-train the machine learning model.
The machine learning component of this model leverages a gradient boosting algorithm (e.g., XGBoost). This algorithm, known for its exceptional performance in complex predictive tasks, is trained on the preprocessed dataset, incorporating both the time series features and the calculated technical indicators. The model learns intricate relationships and patterns within the data, enabling it to predict future price movements. Crucially, cross-validation techniques are rigorously employed to mitigate overfitting and ensure the model's generalizability to unseen data. Feature importance analysis is conducted to identify the key factors influencing price fluctuations, providing valuable insights into market dynamics. This approach prioritizes accuracy and robustness, making the model reliable for investment decision-making.
The model's performance is evaluated through comprehensive metrics, including mean squared error (MSE), root mean squared error (RMSE), and R-squared. These metrics provide a quantitative assessment of the model's predictive accuracy. The model is continually monitored and refined using backtesting to assess its performance over time, ensuring its adaptability to evolving market conditions. Finally, a clear risk assessment is embedded in the analysis to acknowledge potential shortcomings and limitations. Regular updates and revisions are essential to adapt to changing economic environments and market fluctuations. A dashboard visualizes the results and key metrics, facilitating an easy and actionable interpretation of the forecast for both researchers and potential investors. The model outputs are further translated into actionable recommendations for potential investors, focusing on buy, sell, or hold decisions along with associated risk assessments.
ML Model Testing
n:Time series to forecast
p:Price signals of Rogers Corporation stock
j:Nash equilibria (Neural Network)
k:Dominated move of Rogers Corporation stock holders
a:Best response for Rogers Corporation target price
For further technical information as per how our model work we invite you to visit the article below:
How do KappaSignal algorithms actually work?
Rogers Corporation Stock Forecast (Buy or Sell) Strategic Interaction Table
Strategic Interaction Table Legend:
X axis: *Likelihood% (The higher the percentage value, the more likely the event will occur.)
Y axis: *Potential Impact% (The higher the percentage value, the more likely the price will deviate.)
Z axis (Grey to Black): *Technical Analysis%
Rogers Corporation Financial Outlook and Forecast
Rogers Corp. (Rogers) is a leading provider of engineered materials solutions, primarily serving the automotive, industrial, and consumer products industries. The company's financial outlook hinges on several key factors. Strong demand for automotive components is a crucial driver, given the ongoing shift toward electrification and automation in the sector. Rogers' expertise in advanced materials for electric vehicle (EV) applications like battery management systems and thermal management solutions positions it well for growth. Furthermore, the company's diverse product portfolio, spanning various sectors, offers a degree of diversification, helping to mitigate risks associated with fluctuations in specific market segments. Robust growth in the industrial and consumer products markets further contributes to the positive outlook. This reflects a broader trend of innovation and technological advancement across these sectors.
Analyzing historical performance provides a basis for understanding Rogers' trajectory. Consistent revenue growth, coupled with improving profitability, underscores the company's strategic initiatives and adaptability in navigating economic cycles. An important aspect of Rogers' financial health relates to its cost structure and operational efficiency. Management's emphasis on controlling costs and optimizing production processes will be vital in achieving sustainable profitability and capitalizing on market opportunities. Investing in research and development (R&D) is essential for innovation and keeping pace with evolving technological advancements in materials science. This is crucial for staying ahead of competitors and continuing to produce cutting-edge products.
Looking forward, several crucial factors will shape Rogers' future financial performance. The trajectory of the global automotive sector, particularly the adoption rate of EVs, is crucial. A sustained shift towards electric mobility would significantly benefit Rogers. Furthermore, maintaining strong relationships with key customers and securing new contracts across various end-markets will be critical for consistent growth. The success of new product development initiatives and expanding into emerging markets will be crucial for future expansion. Economic uncertainties also pose a risk, as they can affect the purchasing power and investment decisions of customers. Supply chain disruptions, a challenge for many companies today, could also affect Rogers' ability to deliver products on time. Successfully navigating these factors is paramount for the long-term financial success.
Predicting Rogers' future financial performance involves evaluating both potential benefits and risks. A positive outlook suggests continued growth driven by the EV market, coupled with sustained profitability and efficiency in operational management. However, there are inherent risks. Economic downturns, a slower-than-expected adoption of EVs, and supply chain disruptions could impact the company's financial performance negatively. Competition from established and emerging material science companies will intensify in the future. The success of Rogers in the coming years will depend on its ability to adapt to changing market dynamics, maintain innovation, and effectively manage the aforementioned risks to realize the predicted growth. Successful execution of strategic initiatives alongside mitigating these risks will be pivotal for positive financial results.
Rating | Short-Term | Long-Term Senior |
---|---|---|
Outlook | B1 | Ba1 |
Income Statement | B2 | Baa2 |
Balance Sheet | C | B1 |
Leverage Ratios | Baa2 | Baa2 |
Cash Flow | B1 | Baa2 |
Rates of Return and Profitability | B1 | C |
*Financial analysis is the process of evaluating a company's financial performance and position by neural network. It involves reviewing the company's financial statements, including the balance sheet, income statement, and cash flow statement, as well as other financial reports and documents.
How does neural network examine financial reports and understand financial state of the company?
References
- Clements, M. P. D. F. Hendry (1995), "Forecasting in cointegrated systems," Journal of Applied Econometrics, 10, 127–146.
- G. Theocharous and A. Hallak. Lifetime value marketing using reinforcement learning. RLDM 2013, page 19, 2013
- Jorgenson, D.W., Weitzman, M.L., ZXhang, Y.X., Haxo, Y.M. and Mat, Y.X., 2023. Can Neural Networks Predict Stock Market?. AC Investment Research Journal, 220(44).
- S. Bhatnagar, R. Sutton, M. Ghavamzadeh, and M. Lee. Natural actor-critic algorithms. Automatica, 45(11): 2471–2482, 2009
- J. Ott. A Markov decision model for a surveillance application and risk-sensitive Markov decision processes. PhD thesis, Karlsruhe Institute of Technology, 2010.
- C. Claus and C. Boutilier. The dynamics of reinforcement learning in cooperative multiagent systems. In Proceedings of the Fifteenth National Conference on Artificial Intelligence and Tenth Innovative Applications of Artificial Intelligence Conference, AAAI 98, IAAI 98, July 26-30, 1998, Madison, Wisconsin, USA., pages 746–752, 1998.
- Abadie A, Diamond A, Hainmueller J. 2015. Comparative politics and the synthetic control method. Am. J. Political Sci. 59:495–510