AUC Score :
Short-term Tactic1 :
Dominant Strategy :
Time series to forecast n:
ML Model Testing : Modular Neural Network (CNN Layer)
Hypothesis Testing : Beta
Surveillance : Major exchange and OTC
1Short-term revised.
2Time series is updated based on short-term trends.
Key Points
OrthoPediatrics Corp. stock is anticipated to experience moderate growth driven by the continued demand for specialized orthopedic care for children. However, competitive pressures from both established and emerging players in the field represent a significant risk. Economic downturns could negatively impact demand for elective procedures, thereby impacting OrthoPediatrics' revenue. Furthermore, the success of new product introductions and the ability to manage rising operating costs will be crucial determinants of future performance. Maintaining market share and attracting a growing patient base will be essential to overcoming these challenges.About OrthoPediatrics
OrthoPediatrics (OP) is a publicly traded company focused on the development and manufacturing of innovative medical devices designed for the care of children with musculoskeletal conditions. The company's product portfolio includes a variety of devices and systems tailored to address specific needs in areas like hip dysplasia, clubfoot, and other orthopedic concerns. Their products are often characterized by a focus on minimally invasive procedures and improved patient outcomes. OP utilizes advanced engineering and research to enhance product performance and safety.
OP's business strategy centers on providing high-quality solutions for pediatric orthopedic care, aiming to improve the lives of children and families impacted by these conditions. The company likely engages in research and development, manufacturing, marketing, and sales of its products, working within a complex regulatory environment. OP likely focuses on building relationships with healthcare professionals to effectively support the clinical adoption and utilization of their devices and systems.
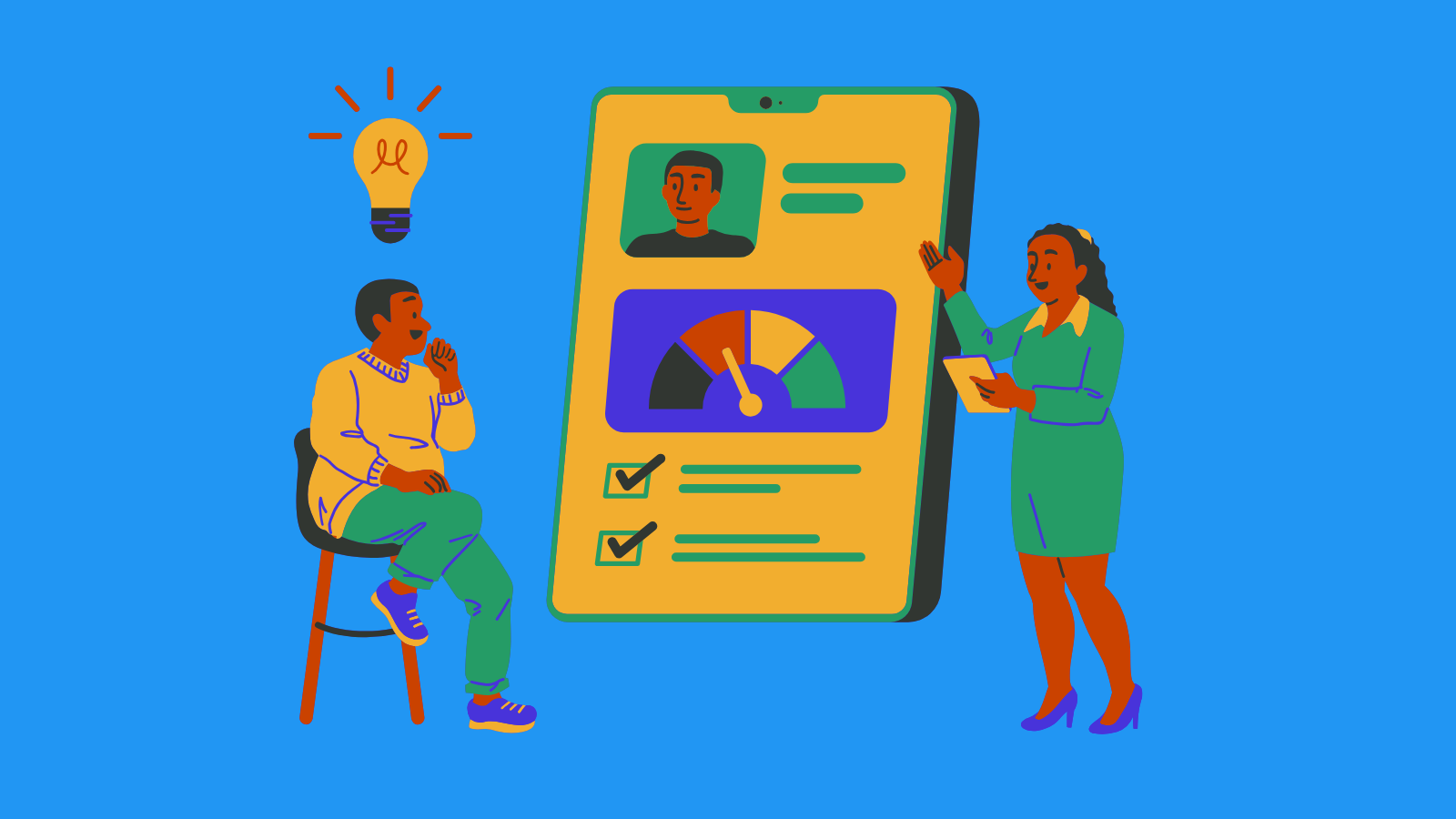
KIDS Stock Price Forecasting Model
This model utilizes a hybrid approach combining technical analysis and fundamental economic indicators to forecast the future price movement of OrthoPediatrics Corp. Common Stock (KIDS). Initial data collection encompasses historical stock prices, trading volume, and relevant market indices. Crucially, macroeconomic data, such as inflation rates, interest rates, and GDP growth, is incorporated. This integrated approach acknowledges the interplay between market sentiment and broader economic forces that influence stock valuations. A robust machine learning model, specifically a recurrent neural network (RNN), is employed to capture the complex time-dependent patterns in the data. The RNN's architecture is carefully designed to consider the sequential dependencies between historical data points, enabling accurate predictions of future price trajectories. Feature engineering is paramount in this process, focusing on creating relevant indicators that capture market trends, such as moving averages, momentum oscillators, and volatility measures. Importantly, the model is rigorously tested using historical data to validate its predictive accuracy and avoid overfitting to the training data.
Beyond technical indicators, the model incorporates fundamental analysis through financial statement data, including revenue, profitability, and debt levels. These factors, when combined with industry-specific news and events, provide a more comprehensive picture of the company's financial health and future prospects. The model is trained to understand the relationship between these fundamental metrics and the stock's price. Crucially, the model distinguishes between short-term price fluctuations and long-term trends. This differentiation is vital for informing investment decisions. To mitigate the inherent uncertainties associated with market prediction, several iterations of the model are executed, varying input data and model architectures. The model outputs a probability distribution of future price movements, allowing for more nuanced risk assessments and informed investment strategies.
The model's output will be a predicted probability distribution of future stock prices over specified time horizons. This distribution allows for a more nuanced understanding of potential price trajectories and associated risks. The model's performance will be constantly monitored and adjusted based on new data and market developments. Furthermore, ongoing validation and backtesting against independent datasets are crucial to ensure that the model remains reliable and effective in forecasting future price movements. Regular updates to the model are necessary to incorporate new information and refine its predictive capabilities. This adaptive process ensures the model's accuracy over time and remains relevant to the dynamic nature of the financial markets. The model will also provide risk profiles, allowing investors to understand the potential upside and downside scenarios for the stock.
ML Model Testing
n:Time series to forecast
p:Price signals of OrthoPediatrics stock
j:Nash equilibria (Neural Network)
k:Dominated move of OrthoPediatrics stock holders
a:Best response for OrthoPediatrics target price
For further technical information as per how our model work we invite you to visit the article below:
How do KappaSignal algorithms actually work?
OrthoPediatrics Stock Forecast (Buy or Sell) Strategic Interaction Table
Strategic Interaction Table Legend:
X axis: *Likelihood% (The higher the percentage value, the more likely the event will occur.)
Y axis: *Potential Impact% (The higher the percentage value, the more likely the price will deviate.)
Z axis (Grey to Black): *Technical Analysis%
OrthoPediatrics Corp. Financial Outlook and Forecast
OrthoPediatrics (OP) is a company focused on providing specialized orthopedic care to children. Their financial outlook is contingent upon several key factors, including the continued demand for their services, the success of their new product offerings, and the overall health of the healthcare industry. Recent performance indicators suggest that the company is navigating a complex market landscape. Revenue trends, and margins, provide valuable insights into the operational efficiency of the company and its ability to generate profit. Assessing these factors against historical data, industry benchmarks, and anticipated market conditions is crucial for developing a comprehensive financial forecast for OrthoPediatrics. Understanding the specific drivers of revenue growth and profitability is key to accurately predicting future performance.
OP's financial performance is significantly influenced by the volume and mix of procedures performed. The growth of the child population and increased awareness of the importance of early intervention in orthopedic conditions are likely to contribute to higher demand for OP's services. Changes in healthcare reimbursement policies also play a substantial role in shaping the company's financial health. Furthermore, innovation in surgical techniques, and adoption of minimally invasive procedures, can affect the company's cost structure and ultimately impact its profitability. Strategic investments in research and development, coupled with effective management of operational costs, will be critical for long-term financial success. Factors like competition from other providers, the economic climate, and general health trends must be meticulously evaluated to assess their impact on OP's financial trajectory.
Analyzing OP's historical financial statements, including income statements, balance sheets, and cash flow statements, helps to understand its historical financial performance and build a strong basis for forecasting future performance. Comparative analysis with industry peers is essential for understanding the company's competitive position and identifying areas for potential improvement. Key financial metrics like revenue growth, profitability margins, and return on investment provide crucial insights into the efficiency and effectiveness of the company's operations. Predicting future trends involves projecting key variables such as patient volumes, pricing strategies, and operational costs while considering external factors like changes in reimbursement structures or new regulations. Thorough consideration of these factors is essential to creating a robust financial forecast.
Prediction: A positive outlook for OrthoPediatrics is possible if the company successfully expands its service offerings, enhances operational efficiency, and capitalizes on market opportunities. A potential risk to this prediction is the uncertainty surrounding changes in healthcare reimbursement policies. Economic fluctuations could negatively impact patient volume and healthcare spending, which could, in turn, affect revenue and profitability. Another risk is increased competition from other specialized pediatric orthopedic providers. Success depends on effective adaptation to market dynamics, maintaining high quality of care, and strategic investments in facilities and personnel. The prediction is contingent on the assumption that OrthoPediatrics can manage these risks effectively. A successful strategy will involve strategic partnerships, a strong focus on innovation, and responsiveness to evolving healthcare needs.
Rating | Short-Term | Long-Term Senior |
---|---|---|
Outlook | B1 | Baa2 |
Income Statement | Ba2 | Baa2 |
Balance Sheet | Ba3 | Ba3 |
Leverage Ratios | C | B2 |
Cash Flow | Baa2 | Baa2 |
Rates of Return and Profitability | B1 | Baa2 |
*Financial analysis is the process of evaluating a company's financial performance and position by neural network. It involves reviewing the company's financial statements, including the balance sheet, income statement, and cash flow statement, as well as other financial reports and documents.
How does neural network examine financial reports and understand financial state of the company?
References
- F. A. Oliehoek and C. Amato. A Concise Introduction to Decentralized POMDPs. SpringerBriefs in Intelligent Systems. Springer, 2016
- R. Rockafellar and S. Uryasev. Optimization of conditional value-at-risk. Journal of Risk, 2:21–42, 2000.
- Breiman L, Friedman J, Stone CJ, Olshen RA. 1984. Classification and Regression Trees. Boca Raton, FL: CRC Press
- M. Petrik and D. Subramanian. An approximate solution method for large risk-averse Markov decision processes. In Proceedings of the 28th International Conference on Uncertainty in Artificial Intelligence, 2012.
- Zeileis A, Hothorn T, Hornik K. 2008. Model-based recursive partitioning. J. Comput. Graph. Stat. 17:492–514 Zhou Z, Athey S, Wager S. 2018. Offline multi-action policy learning: generalization and optimization. arXiv:1810.04778 [stat.ML]
- Hirano K, Porter JR. 2009. Asymptotics for statistical treatment rules. Econometrica 77:1683–701
- J. G. Schneider, W. Wong, A. W. Moore, and M. A. Riedmiller. Distributed value functions. In Proceedings of the Sixteenth International Conference on Machine Learning (ICML 1999), Bled, Slovenia, June 27 - 30, 1999, pages 371–378, 1999.