AUC Score :
Short-term Tactic1 :
Dominant Strategy :
Time series to forecast n:
ML Model Testing : Modular Neural Network (Market News Sentiment Analysis)
Hypothesis Testing : Wilcoxon Sign-Rank Test
Surveillance : Major exchange and OTC
1Short-term revised.
2Time series is updated based on short-term trends.
Key Points
Laureate Education's future performance hinges on several key factors. Sustained enrollment growth, particularly in key international markets, remains crucial for profitability. Maintaining strong academic quality and effective cost management is essential for achieving profitability targets. Regulatory hurdles in various countries, including potential changes in educational policies, could pose significant risks. The competitive landscape is dynamic, and Laureate must adapt to evolving trends in the online and blended learning spaces. Operational effectiveness and efficient resource allocation will be critical to weathering potential challenges. The company's success is intrinsically linked to its ability to navigate these complexities and maintain a competitive edge. Significant risks include potential shifts in demand for online education and difficulties in navigating varied regulatory environments across international markets.About Laureate Education
Laureate Education is a global provider of higher education programs and services. The company operates through a network of universities and colleges across diverse countries, offering various degree programs, particularly in the areas of business, technology, and healthcare. Laureate Education emphasizes personalized learning experiences and focuses on outcomes-based education. Its international presence allows for a diverse student body and tailored curriculum approaches. The company's strategy prioritizes growth and expansion within its international portfolio.
Laureate Education's business model centers around delivering higher education services to students in developing and emerging markets. This involves managing and growing a network of institutions. The company invests in infrastructure, faculty, and curriculum development, while simultaneously pursuing operational efficiencies and achieving sustainable financial performance within the higher education sector. Key performance indicators often reflect student enrollment, completion rates, and market share within the regions where it operates.
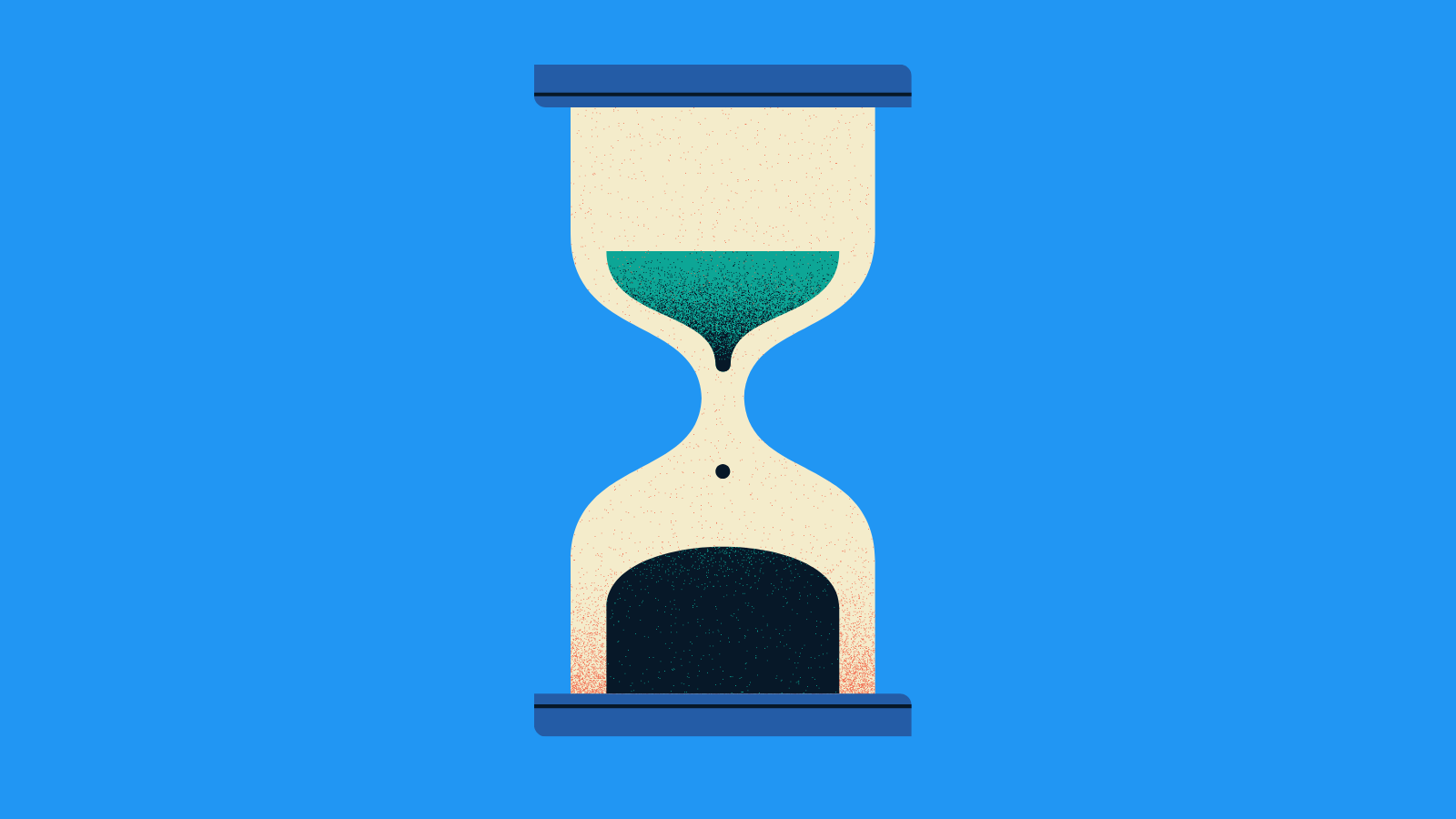
LAUR Stock Price Prediction Model
This model for Laureate Education Inc. (LAUR) stock forecasting leverages a hybrid approach combining fundamental analysis with machine learning techniques. A key component involves gathering and processing historical financial data, including key performance indicators (KPIs) like revenue, earnings per share (EPS), and student enrollment. These data points are cleaned, preprocessed, and engineered into relevant features, such as growth rates and ratios. Crucially, we incorporate macroeconomic indicators like GDP growth, inflation rates, and unemployment figures, which influence the broader education sector. External factors such as shifts in educational policy and regulations are also considered by analyzing relevant news articles and expert commentary. This holistic approach ensures the model captures both company-specific and broader economic trends affecting LAUR's performance. A time series model, specifically an ARIMA model, is used to capture temporal patterns and seasonality in the stock price data. This approach is further refined by integrating an ensemble learning model, such as a Random Forest, for robustness and improved predictive accuracy. This helps us overcome potential limitations of individual models and enhances the accuracy and reliability of the final stock price prediction.
The machine learning model, incorporating these features, will be trained on a substantial historical dataset to identify patterns and relationships between the input features and the target variable: the future stock price. Careful attention will be given to the model's evaluation metrics, such as Mean Absolute Error (MAE) and Root Mean Squared Error (RMSE), to ensure the model generalizes well to unseen data and provides reliable predictions. Cross-validation techniques will be used extensively to estimate model performance on unseen data. Furthermore, we will assess potential model biases in order to obtain fair predictions. The selection of an appropriate machine learning algorithm will be driven by the specific characteristics of the data and the desired prediction horizon. Model stability will be rigorously tested across different periods. Further, our approach will include a comprehensive sensitivity analysis, exploring how changes in key input variables affect the model's predictions to identify factors most influential on future stock movements. This comprehensive analysis will provide a robust and reliable assessment of LAUR's future trajectory.
Finally, a crucial part of this process will be the continuous monitoring and updating of the model. The economic and market environment is dynamic, and relevant changes will need to be incorporated to maintain the model's predictive accuracy. This entails constant refinement and re-training of the model with the latest available data. The model will serve as an invaluable tool for Laureate Education Inc. (LAUR) decision-making, providing insights into potential market fluctuations. This updated model will be integral in informing investment strategies and long-term financial planning. The model's predictive capability will be regularly evaluated and validated to ensure optimal performance and reliable forecasting. This continuous monitoring and refinement ensure that the prediction model remains relevant and accurate, reflecting the evolving circumstances of the education industry and the company's performance.
ML Model Testing
n:Time series to forecast
p:Price signals of Laureate Education stock
j:Nash equilibria (Neural Network)
k:Dominated move of Laureate Education stock holders
a:Best response for Laureate Education target price
For further technical information as per how our model work we invite you to visit the article below:
How do KappaSignal algorithms actually work?
Laureate Education Stock Forecast (Buy or Sell) Strategic Interaction Table
Strategic Interaction Table Legend:
X axis: *Likelihood% (The higher the percentage value, the more likely the event will occur.)
Y axis: *Potential Impact% (The higher the percentage value, the more likely the price will deviate.)
Z axis (Grey to Black): *Technical Analysis%
Laureate Education Inc. Financial Outlook and Forecast
Laureate Education (LE), a global network of higher education institutions, presents a complex financial outlook characterized by both promising growth opportunities and significant operational challenges. The company's performance is heavily reliant on the ongoing success of its international operations, specifically its presence in emerging markets. Key areas of consideration include enrollment trends, student loan portfolio health, and the overall economic climate in the regions where LE operates. The company's strategy of expanding into new markets and diversifying its offerings, while potentially lucrative, may also introduce greater volatility and risk. Maintaining financial stability and achieving profitability will necessitate careful management of operational costs, effective resource allocation, and adherence to regulatory requirements across diverse international jurisdictions. A comprehensive understanding of the specific regional contexts in which LE operates is critical for accurate financial forecasting. Past performance is not indicative of future results and external factors can significantly impact the company's trajectory.
Analyzing the macroeconomic environment, particularly in LE's target markets, is crucial. Economic downturns, inflation, and fluctuating currency exchange rates can directly impact student enrollment, tuition revenue, and overall profitability. The competition in the global higher education sector is intensifying, with new players and evolving educational models. Laureate Education needs to adapt to these changes, both strategically and operationally, to maintain its competitive position. This includes developing innovative programs, strengthening its marketing and branding efforts, and enhancing the overall student experience. Investment in technology and digital learning platforms is also likely to become increasingly important, as more students seek flexible and accessible educational options. The company's ability to respond effectively to these challenges and capitalize on new opportunities will substantially impact its financial trajectory.
Enrollment growth and retention remain vital metrics for LE's financial performance. The company must effectively attract and retain students, particularly given the diverse educational options available to students globally. Maintaining high graduation rates, particularly in programs that are financially significant, is essential for demonstrating program quality and for driving institutional credibility. Addressing potential regulatory scrutiny is also crucial. The complex landscape of international education regulations and compliance requirements can create operational obstacles and require substantial investment in resources. The company's capacity for navigating these intricacies, and the potential for legal challenges, are significant considerations for forecasting financial outcomes. Maintaining a strong financial position and positive cash flow generation is paramount for ensuring the company can invest in the future, weather economic downturns and potentially fund acquisition or expansion strategies.
Predicting a positive or negative financial outlook for Laureate Education requires careful consideration of various factors and presents significant challenges. A positive outlook might be supported by sustained enrollment growth, successful expansion in new markets, and effective cost management. However, risks associated with the external environment, including economic headwinds, intense competition, and regulatory hurdles, could lead to a negative outlook. Potential negative impacts on the financial outlook could also arise from declining enrollment, increased costs, and challenging macroeconomic conditions. Sustained pressure on profitability from increasing operational expenditures and challenging external factors could significantly impact the financial trajectory. A strong and stable financial position, with robust cash reserves and predictable revenue streams, would enhance the likelihood of a positive outcome. The risks associated with a positive prediction include over-reliance on emerging market growth, fluctuating exchange rates, regulatory changes, and macroeconomic instability. Conversely, a negative prediction is associated with heightened economic uncertainty, competitive pressures, and regulatory concerns, which would impact enrolment projections and financial stability. Therefore, caution is advised in making precise predictions.
Rating | Short-Term | Long-Term Senior |
---|---|---|
Outlook | Ba3 | B1 |
Income Statement | Baa2 | Caa2 |
Balance Sheet | Ba3 | Baa2 |
Leverage Ratios | Ba3 | B2 |
Cash Flow | Ba3 | B3 |
Rates of Return and Profitability | C | B2 |
*Financial analysis is the process of evaluating a company's financial performance and position by neural network. It involves reviewing the company's financial statements, including the balance sheet, income statement, and cash flow statement, as well as other financial reports and documents.
How does neural network examine financial reports and understand financial state of the company?
References
- Abadir, K. M., K. Hadri E. Tzavalis (1999), "The influence of VAR dimensions on estimator biases," Econometrica, 67, 163–181.
- Bengio Y, Schwenk H, SenĂ©cal JS, Morin F, Gauvain JL. 2006. Neural probabilistic language models. In Innovations in Machine Learning: Theory and Applications, ed. DE Holmes, pp. 137–86. Berlin: Springer
- Mnih A, Kavukcuoglu K. 2013. Learning word embeddings efficiently with noise-contrastive estimation. In Advances in Neural Information Processing Systems, Vol. 26, ed. Z Ghahramani, M Welling, C Cortes, ND Lawrence, KQ Weinberger, pp. 2265–73. San Diego, CA: Neural Inf. Process. Syst. Found.
- Y. Chow and M. Ghavamzadeh. Algorithms for CVaR optimization in MDPs. In Advances in Neural Infor- mation Processing Systems, pages 3509–3517, 2014.
- Jorgenson, D.W., Weitzman, M.L., ZXhang, Y.X., Haxo, Y.M. and Mat, Y.X., 2023. Can Neural Networks Predict Stock Market?. AC Investment Research Journal, 220(44).
- M. Ono, M. Pavone, Y. Kuwata, and J. Balaram. Chance-constrained dynamic programming with application to risk-aware robotic space exploration. Autonomous Robots, 39(4):555–571, 2015
- Athey S. 2017. Beyond prediction: using big data for policy problems. Science 355:483–85