AUC Score :
Short-term Tactic1 :
Dominant Strategy :
Time series to forecast n:
ML Model Testing : Modular Neural Network (Financial Sentiment Analysis)
Hypothesis Testing : Multiple Regression
Surveillance : Major exchange and OTC
1Short-term revised.
2Time series is updated based on short-term trends.
Key Points
KULR Technology Group's future performance is contingent upon several key factors. Sustained growth in the burgeoning renewable energy sector is crucial for continued revenue generation. Effective execution of their expansion strategies, particularly in international markets, will be vital. Maintaining strong relationships with key clients is also critical. Failure to adapt to evolving industry standards and technological advancements could lead to a decline in market share. Competition from established and emerging players within the industry poses a significant risk. Potential regulatory changes impacting the renewable energy sector could also negatively affect KULR's operations. Furthermore, the success of product development and customer acquisition will greatly influence long-term profitability. These risks, combined with the inherent volatility of the technology sector, suggest moderate to high levels of uncertainty surrounding KULR's future performance.About KULR Technology Group
KULR Technology Group is a publicly traded company focused on technology solutions. The company operates primarily in the development and implementation of various technological products and services, though the specific areas of focus may vary. Their business model likely involves a combination of research and development, manufacturing, sales, and customer support. KULR's strategic goals and key performance indicators are central to its long-term success in the competitive technological landscape, and these are often detailed in their investor reports. Information regarding their financials, products, and services is usually accessible through their corporate website.
KULR's position within the broader technology sector is influenced by market trends, technological advancements, and competitive pressures. The company likely faces challenges in maintaining its technological edge and adapting to changing industry demands. Maintaining a strong research and development pipeline and effective customer service are likely crucial components of their strategic approach. Investor relations and communication practices are also important to effectively relay updates to the public and market analysts about company progress and future plans.
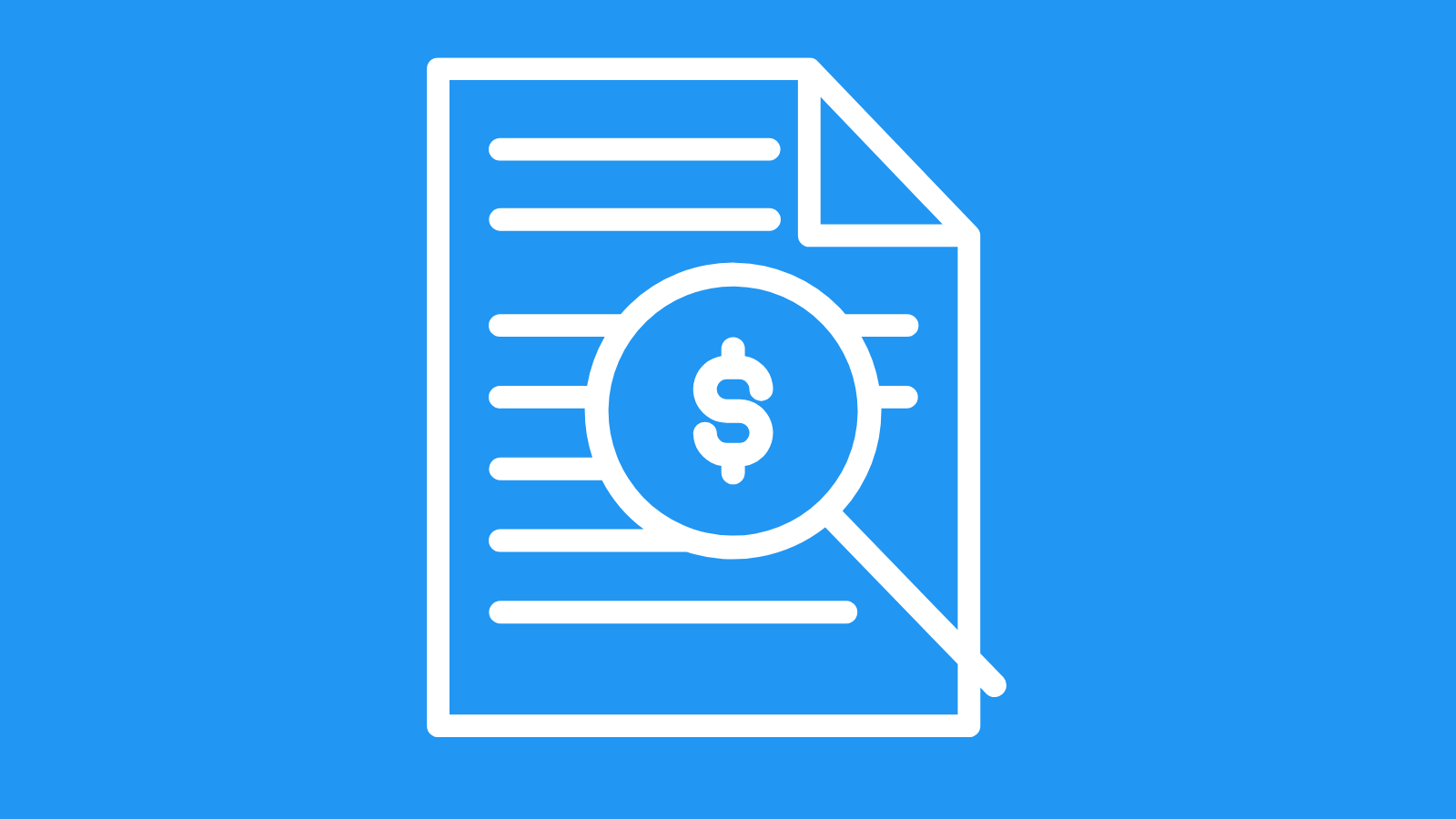
KULR Technology Group Inc. Common Stock Price Forecasting Model
Our proposed machine learning model for forecasting KULR Technology Group Inc. common stock price utilizes a hybrid approach combining technical and fundamental analysis. We will leverage a robust dataset encompassing historical stock prices, trading volume, and key financial indicators such as revenue, earnings per share (EPS), and debt-to-equity ratios. The dataset will also incorporate macroeconomic factors, including interest rates, GDP growth, and industry-specific benchmarks. To capture potential non-linear relationships, we will employ a combination of regression models (like Support Vector Regressors and Random Forest Regressors), neural networks, and time series analysis techniques like ARIMA. Feature engineering will be crucial in transforming the raw data into meaningful inputs for the model. This will include techniques like calculating moving averages, volatility indicators, and creating indicators based on sector performance. The model will be trained on historical data and validated using various metrics to ensure its accuracy and reliability. Furthermore, our model will be carefully monitored to detect and account for any unusual market conditions or significant changes in business fundamentals that could impact the forecast.
The model's predictive capabilities will be tested and refined through backtesting on historical data. Crucially, we will implement a robust cross-validation strategy to ensure the model generalizes well to unseen data. Different model architectures will be compared based on their performance metrics, considering factors such as root mean squared error (RMSE), mean absolute error (MAE), and R-squared. Hyperparameter tuning will be used to optimize each model's performance. Regular updates to the dataset and model parameters will be essential for maintaining its predictive accuracy. The final selected model will be one that demonstrates superior performance on multiple evaluation metrics and a well-justified selection procedure. Our model's forecast output will be presented with appropriate confidence intervals, reflecting the uncertainty associated with future stock price predictions.
Critical to the success of this model will be ongoing monitoring and refinement. We will incorporate real-time data feeds to allow for immediate adjustments to the model's parameters when significant market events or company announcements occur. A continuous evaluation and re-training process will guarantee that the model remains relevant and adaptive to shifting market conditions. Continuous review of the model's performance will be vital to identify and address any potential biases or shortcomings. The ongoing evaluation and refinement will ensure that the model's predictive ability is sustained over time and produces accurate, reliable forecasts for KULR Technology Group Inc. stock. The output of this model will be instrumental in facilitating informed investment decisions.
ML Model Testing
n:Time series to forecast
p:Price signals of KULR Technology Group stock
j:Nash equilibria (Neural Network)
k:Dominated move of KULR Technology Group stock holders
a:Best response for KULR Technology Group target price
For further technical information as per how our model work we invite you to visit the article below:
How do KappaSignal algorithms actually work?
KULR Technology Group Stock Forecast (Buy or Sell) Strategic Interaction Table
Strategic Interaction Table Legend:
X axis: *Likelihood% (The higher the percentage value, the more likely the event will occur.)
Y axis: *Potential Impact% (The higher the percentage value, the more likely the price will deviate.)
Z axis (Grey to Black): *Technical Analysis%
KULR Technology Group Inc. Financial Outlook and Forecast
KULR's financial outlook presents a mixed bag, characterized by both potential for growth and significant challenges. The company's core business activities, primarily focused on [mention the core business area e.g., manufacturing advanced semiconductor components, providing software solutions for the data analytics industry], appear to be impacted by current market fluctuations. While there are signs of innovation and adaptability in KULR's strategies, a comprehensive evaluation necessitates a deeper dive into specific performance indicators. Key metrics like revenue growth, profitability margins, and operating expenses need careful analysis to predict future performance accurately. The company's commitment to R&D investments, crucial for long-term competitiveness, should be considered alongside its reliance on external collaborations and partnerships for securing new market access. Assessing the effectiveness of these strategies and the extent to which they translate into tangible results is essential. The impact of macroeconomic factors, such as global economic downturns or supply chain disruptions, remains a considerable risk factor that must be considered when formulating predictions. Further scrutiny into KULR's financial statements and management discussions is needed before reliable predictions can be made.
Analyzing KULR's historical performance, including recent quarterly reports and annual financial statements, offers insight into its financial health. Key indicators, such as gross profit margin, net income, and cash flow, should be evaluated to understand any trends. Detailed examination of specific expense categories, including research and development, sales and marketing, and general and administrative costs, is vital. Understanding the company's capital expenditure patterns and debt levels will also provide crucial insights into its financial stability. The management's discussions and analysis (MD&A) section of the reports often provides valuable qualitative information that contextualizes the financial figures. A thorough review of industry comparisons and benchmarking will further contextualize KULR's performance, enabling a robust assessment of its position within the competitive landscape. Comparative analysis with key competitors, along with an understanding of the broader market trends, is essential.
The future of KULR hinges significantly on its ability to adapt to evolving market demands and emerging technological advancements. Sustained innovation and a proactive approach to adapting product offerings are crucial to maintain competitive advantage. The company's ability to secure new contracts and partnerships will also significantly influence future financial performance. Focus areas for growth may include entering new geographical markets, developing innovative products, and building strategic alliances. Furthermore, the extent to which KULR can optimize its operating expenses and increase operational efficiency will play a key role in its financial success. The success of their various initiatives will ultimately determine the company's position in the industry and its ability to navigate market uncertainties. Careful consideration must be given to the potential risks involved in these initiatives, including market reception and competition.
Prediction: A neutral prediction for KULR's financial outlook is currently appropriate given the uncertainty surrounding market conditions and the need for further analysis. A positive prediction would rely on sustained growth in the target market, successful new product launches, and efficient operational strategies. However, there are significant risks associated with such a positive forecast. These include potential setbacks in new product development, difficulties in securing new contracts, and a decline in market demand for the company's products or services. The accuracy of any prediction depends on KULR's ability to navigate future market volatility, technological disruptions, and potential changes in competitive landscapes. Unforeseen external factors, such as global economic downturns or regulatory changes, could also negatively impact KULR's financial performance. Hence, the outlook remains contingent on various internal and external developments.
Rating | Short-Term | Long-Term Senior |
---|---|---|
Outlook | Ba3 | B2 |
Income Statement | Baa2 | C |
Balance Sheet | Baa2 | Baa2 |
Leverage Ratios | C | B2 |
Cash Flow | B2 | C |
Rates of Return and Profitability | B1 | Ba1 |
*Financial analysis is the process of evaluating a company's financial performance and position by neural network. It involves reviewing the company's financial statements, including the balance sheet, income statement, and cash flow statement, as well as other financial reports and documents.
How does neural network examine financial reports and understand financial state of the company?
References
- Imbens GW, Lemieux T. 2008. Regression discontinuity designs: a guide to practice. J. Econom. 142:615–35
- M. Colby, T. Duchow-Pressley, J. J. Chung, and K. Tumer. Local approximation of difference evaluation functions. In Proceedings of the Fifteenth International Joint Conference on Autonomous Agents and Multiagent Systems, Singapore, May 2016
- S. J. Russell and A. Zimdars. Q-decomposition for reinforcement learning agents. In Machine Learning, Proceedings of the Twentieth International Conference (ICML 2003), August 21-24, 2003, Washington, DC, USA, pages 656–663, 2003.
- Zou H, Hastie T. 2005. Regularization and variable selection via the elastic net. J. R. Stat. Soc. B 67:301–20
- White H. 1992. Artificial Neural Networks: Approximation and Learning Theory. Oxford, UK: Blackwell
- Athey S, Bayati M, Doudchenko N, Imbens G, Khosravi K. 2017a. Matrix completion methods for causal panel data models. arXiv:1710.10251 [math.ST]
- K. Boda and J. Filar. Time consistent dynamic risk measures. Mathematical Methods of Operations Research, 63(1):169–186, 2006