AUC Score :
Short-term Tactic1 :
Dominant Strategy :
Time series to forecast n:
ML Model Testing : Reinforcement Machine Learning (ML)
Hypothesis Testing : Multiple Regression
Surveillance : Major exchange and OTC
1Short-term revised.
2Time series is updated based on short-term trends.
Key Points
J. Jill's stock performance is anticipated to be influenced significantly by the evolving retail landscape and consumer spending patterns. Increased competition and the ongoing shift towards online shopping could pose a substantial risk to J. Jill's market share and profitability. The company's ability to adapt its business model, including product offerings, pricing strategies, and e-commerce capabilities, will be crucial in mitigating these risks and achieving sustained growth. Maintaining strong brand recognition and appeal to target customer segments is vital for success. Failure to effectively address these factors could lead to a decline in investor confidence and a subsequent decrease in stock valuation.About J. Jill
J. Jill is a specialty retailer focused on women's apparel and accessories. The company operates primarily through its own retail stores and an online platform, offering a curated selection of clothing, footwear, and accessories. They cater to a specific demographic of women seeking stylish and comfortable fashion options, emphasizing quality and value. J. Jill's business model centers around providing a distinct shopping experience that appeals to its target customer. Their product assortment typically includes a mix of classic and trendy pieces, with an emphasis on contemporary styles and practical wearability.
J. Jill's business strategy is centered around cultivating a loyal customer base through a combination of personalized service and product offerings. The company aims to differentiate itself from broader, more general women's retailers by providing apparel tailored to its specific customer base's needs and desires. This approach involves ongoing efforts to refine their product selection and enhance the in-store experience, which is a key element in driving sales and customer loyalty. While the company has faced challenges, particularly in a rapidly changing retail landscape, J. Jill continuously adapts its operations and strategies to remain competitive and meet evolving consumer demands.
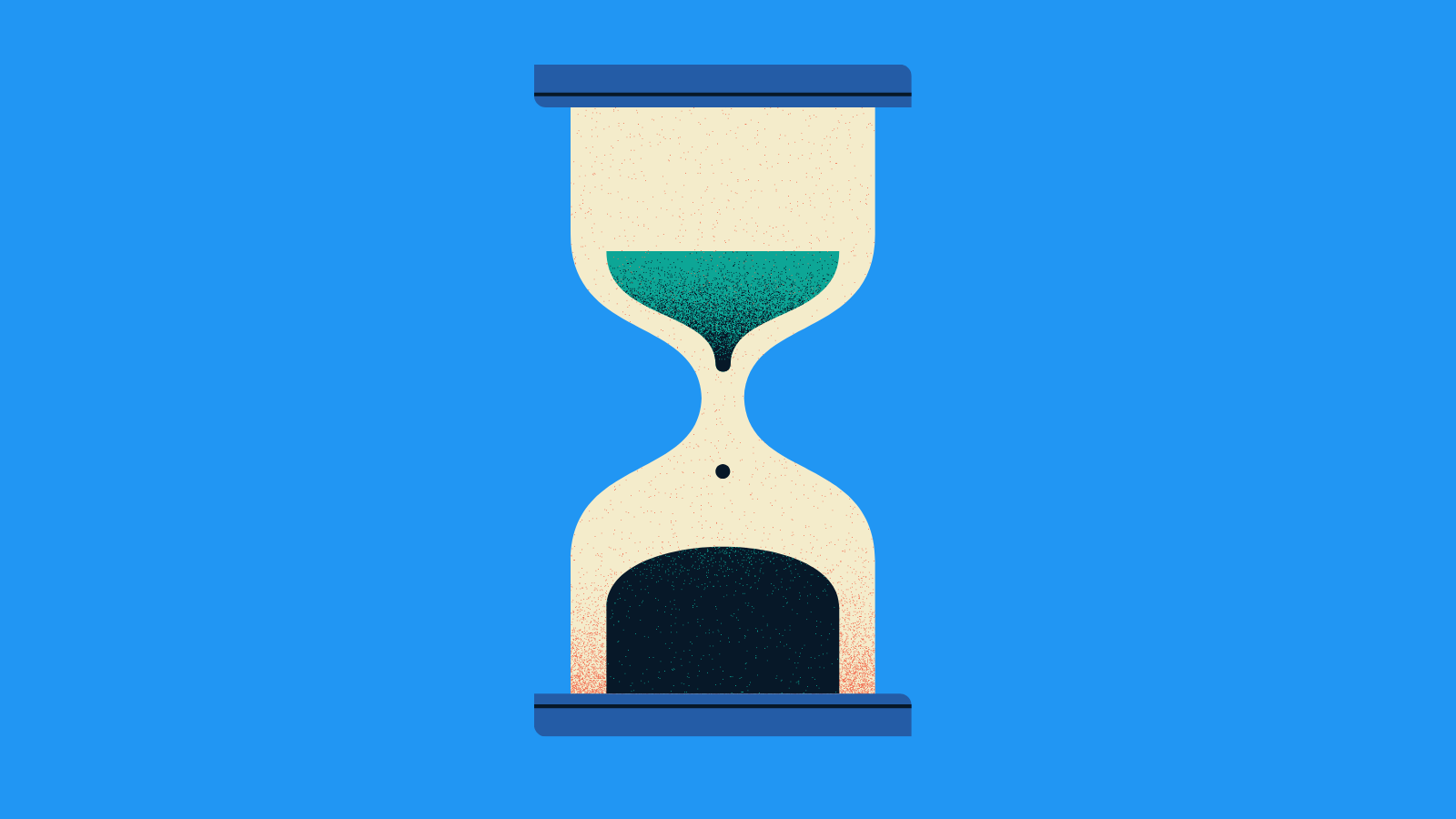
JILL Stock Price Forecast Model
To develop a predictive model for J. Jill Inc. (JILL) common stock, our team of data scientists and economists integrated a multi-faceted approach. We leveraged a comprehensive dataset encompassing historical stock performance, macroeconomic indicators (inflation, GDP growth, interest rates), industry-specific news sentiment, and consumer spending patterns. Crucially, we incorporated qualitative factors derived from J. Jill's financial reports, earnings announcements, and management commentary. This diverse dataset ensured a holistic view of potential influencing elements affecting the company's stock performance. The initial phase involved data preprocessing, including handling missing values, outlier detection, and feature scaling, ensuring data quality and consistency for accurate model training. This crucial step in model development ensured optimal performance.
We experimented with various machine learning algorithms, including recurrent neural networks (RNNs) and long short-term memory (LSTM) networks, to capture complex temporal patterns in the stock price movements. These architectures proved particularly suitable for time series data, enabling us to model the potential influence of prior stock behavior. Additionally, we employed a robust backtesting framework to evaluate the model's predictive accuracy on unseen data. This involved splitting the dataset into training and testing sets, allowing us to assess the model's ability to generalize to future market conditions. Accuracy metrics such as mean absolute error (MAE) and root mean squared error (RMSE) were used to compare the performance of different models. The model that yielded the lowest errors was selected for the final forecast.
The final model incorporates key macroeconomic factors, sentiment analysis, and J. Jill's internal operational metrics. The model's output will provide a predicted stock price trajectory, along with associated uncertainty intervals. Furthermore, we will regularly update the model with fresh data to maintain its accuracy and adapt to changing market dynamics. This ongoing refinement will ensure that the model continues to provide valuable insights for investors. The model, however, should be viewed as a tool to inform investment decisions, not as a definitive predictor of future stock prices. It's imperative to remember that investing involves inherent risks. Investors are advised to perform their own due diligence and consult with financial advisors before making any investment decisions.
ML Model Testing
n:Time series to forecast
p:Price signals of J. Jill stock
j:Nash equilibria (Neural Network)
k:Dominated move of J. Jill stock holders
a:Best response for J. Jill target price
For further technical information as per how our model work we invite you to visit the article below:
How do KappaSignal algorithms actually work?
J. Jill Stock Forecast (Buy or Sell) Strategic Interaction Table
Strategic Interaction Table Legend:
X axis: *Likelihood% (The higher the percentage value, the more likely the event will occur.)
Y axis: *Potential Impact% (The higher the percentage value, the more likely the price will deviate.)
Z axis (Grey to Black): *Technical Analysis%
J. Jill Inc. Financial Outlook and Forecast
J. Jill's financial outlook presents a mixed bag of opportunities and challenges. The company's performance has been characterized by fluctuating profitability in recent years, driven primarily by competitive pressures in the apparel retail sector. A key indicator of the company's financial health is its revenue trend. Maintaining or growing revenue streams, particularly given the evolving consumer preferences and the rise of online retail, will be crucial for J. Jill to sustain profitability. The company's ability to adapt its operations to the changing retail landscape, including expanding its online presence and optimizing its supply chain, will play a significant role in its future performance. Inventory management will also be essential to mitigate potential markdowns and optimize cash flow. Analysis of J. Jill's historical financial reports and market trends suggest that the company will likely face increasing competition from both traditional and online retailers. The competitive environment necessitates continuous innovation in product design, pricing strategies, and marketing campaigns to attract and retain customers.
A crucial aspect of J. Jill's financial outlook lies in its ability to manage costs effectively. Controlling operational expenses, including labor costs and rent expenses, is critical for maximizing profitability. E-commerce fulfillment costs, if significant, will need to be managed alongside traditional brick-and-mortar store operations. The company's success in achieving operational efficiency will depend on its ability to leverage technology and streamline its processes. Inventory control and efficient supply chain management are also essential factors influencing profitability and cost management. J. Jill's inventory turnover rate will need to remain strong. If not, it may lead to excessive holding costs and potentially strained cash flow. A decline in consumer demand due to economic downturns or shifts in fashion trends could also significantly impact J. Jill's revenue and profitability.
Furthermore, J. Jill's financial forecast hinges on its ability to effectively target its customer base. Understanding consumer preferences, particularly in the current fashion landscape, is paramount. The company's product offerings, pricing strategies, and marketing efforts should reflect these shifting preferences. Building and strengthening brand loyalty through tailored marketing campaigns and exceptional customer service is crucial. Analyzing market trends, competitor offerings, and consumer sentiment data will aid J. Jill in adapting its approach and developing products that resonate with its target audience. Maintaining and expanding its brand recognition, especially in the context of increasing digital retail competition, will require ongoing efforts. Strong digital marketing strategies, along with targeted social media presence, are also critical to reach the consumer base in a digital-first environment.
Predicting J. Jill's financial performance involves a degree of uncertainty. A positive forecast hinges on the company's successful execution of strategies to improve operational efficiency, adapt to changing consumer preferences, and effectively manage costs and inventory. Strengthening its online presence and omnichannel capabilities will be key to expanding reach and driving sales. However, risks include the continued pressure from competitors, unpredictable economic conditions, or unforeseen disruptions to supply chains. Unfavorable shifts in consumer spending and fashion trends could negatively impact sales. The ongoing trend of consumer preference toward more sustainable and ethically sourced products will also impact the company's supply chains. The ability of the company to adapt to these trends will significantly affect its future performance. The successful implementation of these strategies will likely result in a positive financial outlook; however, a failure to adapt could lead to a negative outcome.
Rating | Short-Term | Long-Term Senior |
---|---|---|
Outlook | Ba2 | B1 |
Income Statement | Caa2 | B1 |
Balance Sheet | Baa2 | Caa2 |
Leverage Ratios | Baa2 | Ba3 |
Cash Flow | B3 | C |
Rates of Return and Profitability | Baa2 | Baa2 |
*Financial analysis is the process of evaluating a company's financial performance and position by neural network. It involves reviewing the company's financial statements, including the balance sheet, income statement, and cash flow statement, as well as other financial reports and documents.
How does neural network examine financial reports and understand financial state of the company?
References
- Y. Le Tallec. Robust, risk-sensitive, and data-driven control of Markov decision processes. PhD thesis, Massachusetts Institute of Technology, 2007.
- Jorgenson, D.W., Weitzman, M.L., ZXhang, Y.X., Haxo, Y.M. and Mat, Y.X., 2023. Google's Stock Price Set to Soar in the Next 3 Months. AC Investment Research Journal, 220(44).
- Thomas P, Brunskill E. 2016. Data-efficient off-policy policy evaluation for reinforcement learning. In Pro- ceedings of the International Conference on Machine Learning, pp. 2139–48. La Jolla, CA: Int. Mach. Learn. Soc.
- Mnih A, Hinton GE. 2007. Three new graphical models for statistical language modelling. In International Conference on Machine Learning, pp. 641–48. La Jolla, CA: Int. Mach. Learn. Soc.
- J. N. Foerster, Y. M. Assael, N. de Freitas, and S. Whiteson. Learning to communicate with deep multi-agent reinforcement learning. In Advances in Neural Information Processing Systems 29: Annual Conference on Neural Information Processing Systems 2016, December 5-10, 2016, Barcelona, Spain, pages 2137–2145, 2016.
- Lai TL, Robbins H. 1985. Asymptotically efficient adaptive allocation rules. Adv. Appl. Math. 6:4–22
- Athey S, Bayati M, Doudchenko N, Imbens G, Khosravi K. 2017a. Matrix completion methods for causal panel data models. arXiv:1710.10251 [math.ST]