AUC Score :
Short-term Tactic1 :
Dominant Strategy :
Time series to forecast n:
ML Model Testing : Transfer Learning (ML)
Hypothesis Testing : Linear Regression
Surveillance : Major exchange and OTC
1Short-term revised.
2Time series is updated based on short-term trends.
Key Points
The IDX Composite index is anticipated to experience a period of moderate volatility. Factors influencing this projection include the ongoing global economic climate, particularly interest rate fluctuations and potential shifts in investor sentiment. Significant economic headwinds, such as a prolonged period of high inflation or a global recession, could lead to a substantial decline in the index. Conversely, a more favorable economic environment might support upward momentum. Political uncertainties, both locally and internationally, could also introduce periods of pronounced price swings. Sustained growth in specific sectors, like technology or consumer discretionary, could provide support. The degree of risk associated with these predictions is considerable, as the interplay of these factors is complex and unpredictable. Precise estimations are inherently challenging.About IDX Composite Index
The IDX Composite index is a broad market indicator of the Indonesian stock market. It measures the performance of the majority of publicly traded companies listed on the Indonesia Stock Exchange (IDX). The index reflects the overall direction of the Indonesian equity market, providing a benchmark for investment and trading strategies within the country. It is comprised of various sectors and companies, thus offering a comprehensive overview of economic activity and market sentiment.
The IDX Composite's construction and methodology are designed to maintain relevance and accuracy in representing the diverse Indonesian equity landscape. Regular adjustments and revisions are implemented to account for listing changes, delistings, and capital structure modifications. This ensures the index continues to provide a reliable snapshot of market performance over time.
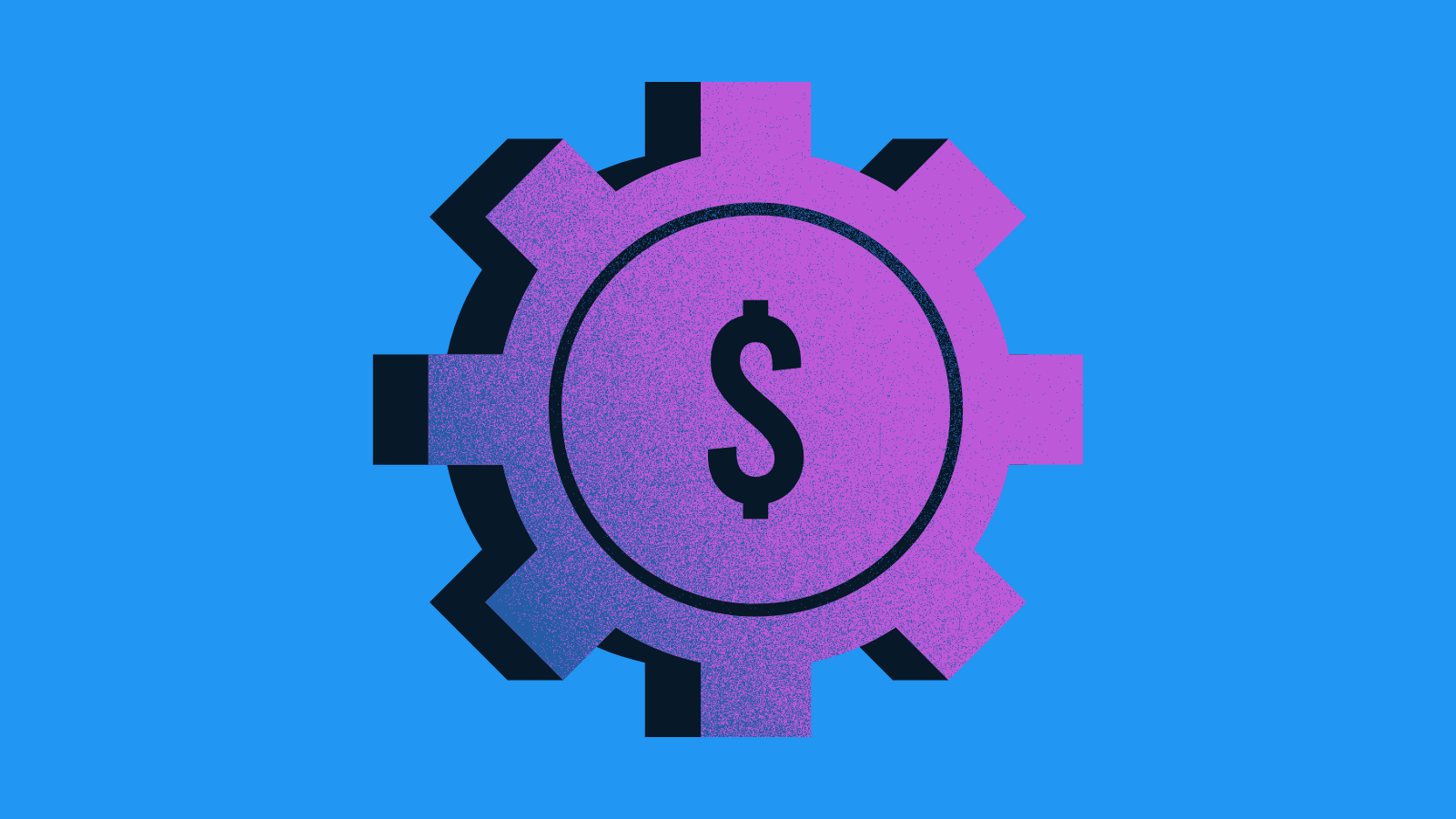
IDX Composite Index Forecasting Model
Our model for forecasting the IDX Composite index leverages a combination of time series analysis and machine learning techniques. We begin by preprocessing the historical data, addressing potential issues like missing values and outliers. Crucially, we incorporate macroeconomic indicators, such as GDP growth, inflation rates, and interest rates, as features. These indicators provide contextual understanding of the broader economic environment influencing the index's performance. We employ a robust feature engineering process to create derived variables, such as moving averages, rate of change, and seasonality indicators. These engineered features capture patterns and trends not readily apparent in the raw data, ultimately improving the model's predictive accuracy. We utilize a blend of various machine learning algorithms, including ARIMA models, LSTM networks, and gradient boosting methods, to generate forecasts. A crucial step is model selection using metrics such as RMSE, MAE, and MAPE, ensuring that the chosen algorithm delivers the best predictive performance based on historical data. The chosen model will be robust and dependable for future forecasting and insights.
The model's training process involves splitting the historical data into training, validation, and testing sets. The training set is used to optimize the model's parameters, while the validation set is used to tune the hyperparameters and prevent overfitting. Rigorous backtesting and validation are essential to confirm the model's efficacy and robustness. This process includes evaluating the model's performance across different time periods and under various economic conditions. An important aspect of the validation phase is the assessment of the model's ability to generalize to unseen data. This involves comparing the model's forecasts with actual market outcomes. Regular model monitoring and updating are paramount to address any potential structural breaks or shifts in the market dynamics. This dynamic approach will ensure that the model continues to perform effectively under evolving conditions.
Finally, the model outputs predictions for future values of the IDX Composite index. These forecasts are presented with associated confidence intervals, allowing for a clear understanding of the uncertainty inherent in the predictions. The output will include not only the forecasted value but also a measure of its confidence, aiding users in making informed decisions. The model's results are further analyzed and presented in easily understandable visualizations, facilitating communication of findings. These visualizations help stakeholders understand the model's performance, the impact of key features, and the forecast's implications for investment strategies. The model's outputs are then integrated with user-friendly reporting tools for practical application by financial analysts and investors.
ML Model Testing
n:Time series to forecast
p:Price signals of IDX Composite index
j:Nash equilibria (Neural Network)
k:Dominated move of IDX Composite index holders
a:Best response for IDX Composite target price
For further technical information as per how our model work we invite you to visit the article below:
How do KappaSignal algorithms actually work?
IDX Composite Index Forecast Strategic Interaction Table
Strategic Interaction Table Legend:
X axis: *Likelihood% (The higher the percentage value, the more likely the event will occur.)
Y axis: *Potential Impact% (The higher the percentage value, the more likely the price will deviate.)
Z axis (Grey to Black): *Technical Analysis%
IDX Composite Index Financial Outlook and Forecast
The Indonesian Stock Exchange Composite Index (IDX Composite) is a crucial barometer of the Indonesian economy's health, reflecting the performance of publicly listed companies. A detailed analysis of the index's financial outlook necessitates consideration of several key factors. These include the ongoing macroeconomic environment, particularly global economic conditions, the strength of the Indonesian Rupiah, and the performance of various sectors within the Indonesian economy. Current government policies and initiatives, such as infrastructure development programs and efforts to improve business competitiveness, also play a substantial role in shaping the index's future trajectory. Recent shifts in investor sentiment and market trends, including foreign investment flows and domestic investor confidence, are further variables impacting the index's performance. Understanding the interactions between these factors is crucial for assessing the likely direction of the IDX Composite and for generating informed investment strategies.
Several economic indicators suggest both opportunities and challenges for the index. Positive developments, such as robust domestic consumption and expanding industrial sectors, suggest potential for growth. However, external economic headwinds, such as fluctuating global commodity prices and shifts in global interest rates, could exert significant downward pressure on the index. Moreover, the volatility of the Indonesian Rupiah against major currencies poses a risk to foreign investors and potentially impacts the index's value. The stability of the financial sector, along with the effectiveness of regulatory policies, is also a significant factor in maintaining investor confidence. It is important to acknowledge that the index's performance can be impacted by unexpected events, such as natural disasters or geopolitical instability, which can create short-term fluctuations.
Forecasting the IDX Composite requires careful consideration of the aforementioned factors. Analysts and experts employ a variety of modeling techniques to estimate future performance, often incorporating fundamental data from companies listed on the exchange, including revenue, profit margins, and future growth projections. Companies' financial strength, debt levels, and potential for earnings growth are critical components in evaluating the index's prospects. While some models predict steady, moderate growth in the index, others anticipate more significant fluctuations based on external market forces and internal economic factors within Indonesia. A significant challenge in forecasting the IDX Composite is the unpredictable nature of global economic events and the inherent volatility of emerging markets, which can shift market sentiment rapidly and significantly affect the index's trajectory.
Predicting the future direction of the IDX Composite presents a challenge due to the complexity of the factors influencing its performance. A cautiously optimistic outlook suggests that the IDX Composite could see modest growth over the foreseeable future, but this is contingent upon factors such as sustained domestic economic activity, a stable currency, and favorable global economic conditions. However, there are significant risks associated with this prediction. Adverse global economic conditions, significant geopolitical events, or unexpected shifts in investor sentiment could dramatically impact the index's performance, potentially leading to a decline. Additionally, the vulnerability of the Indonesian economy to external shocks necessitates a cautious approach to any investment strategy based on a forecast for the IDX Composite.
Rating | Short-Term | Long-Term Senior |
---|---|---|
Outlook | B2 | B2 |
Income Statement | C | B2 |
Balance Sheet | Ba2 | Caa2 |
Leverage Ratios | C | Baa2 |
Cash Flow | B2 | C |
Rates of Return and Profitability | Baa2 | B3 |
*An aggregate rating for an index summarizes the overall sentiment towards the companies it includes. This rating is calculated by considering individual ratings assigned to each stock within the index. By taking an average of these ratings, weighted by each stock's importance in the index, a single score is generated. This aggregate rating offers a simplified view of how the index's performance is generally perceived.
How does neural network examine financial reports and understand financial state of the company?
References
- M. Puterman. Markov Decision Processes: Discrete Stochastic Dynamic Programming. Wiley, New York, 1994.
- Harris ZS. 1954. Distributional structure. Word 10:146–62
- R. Sutton, D. McAllester, S. Singh, and Y. Mansour. Policy gradient methods for reinforcement learning with function approximation. In Proceedings of Advances in Neural Information Processing Systems 12, pages 1057–1063, 2000
- J. N. Foerster, Y. M. Assael, N. de Freitas, and S. Whiteson. Learning to communicate with deep multi-agent reinforcement learning. In Advances in Neural Information Processing Systems 29: Annual Conference on Neural Information Processing Systems 2016, December 5-10, 2016, Barcelona, Spain, pages 2137–2145, 2016.
- Zou H, Hastie T. 2005. Regularization and variable selection via the elastic net. J. R. Stat. Soc. B 67:301–20
- Breusch, T. S. A. R. Pagan (1979), "A simple test for heteroskedasticity and random coefficient variation," Econometrica, 47, 1287–1294.
- V. Mnih, K. Kavukcuoglu, D. Silver, A. Rusu, J. Veness, M. Bellemare, A. Graves, M. Riedmiller, A. Fidjeland, G. Ostrovski, S. Petersen, C. Beattie, A. Sadik, I. Antonoglou, H. King, D. Kumaran, D. Wierstra, S. Legg, and D. Hassabis. Human-level control through deep reinforcement learning. Nature, 518(7540):529–533, 02 2015.