AUC Score :
Short-term Tactic1 :
Dominant Strategy :
Time series to forecast n:
ML Model Testing : Modular Neural Network (Market Volatility Analysis)
Hypothesis Testing : Sign Test
Surveillance : Major exchange and OTC
1Short-term revised.
2Time series is updated based on short-term trends.
Key Points
The S&P GSCI Gold index is anticipated to exhibit volatility, potentially driven by fluctuating macroeconomic conditions. A sustained period of economic uncertainty or heightened inflation concerns could lead to increased demand for gold as a safe-haven asset, resulting in a positive price trend. Conversely, a strengthening of the US dollar, or sustained period of economic optimism, might exert downward pressure on gold prices. The key risk factors are the interplay between global economic growth, geopolitical events, and monetary policy decisions. This complex interplay makes precise predictions challenging. Significant price movements are possible, particularly in response to unexpected events.About S&P GSCI Gold Index
This exclusive content is only available to premium users.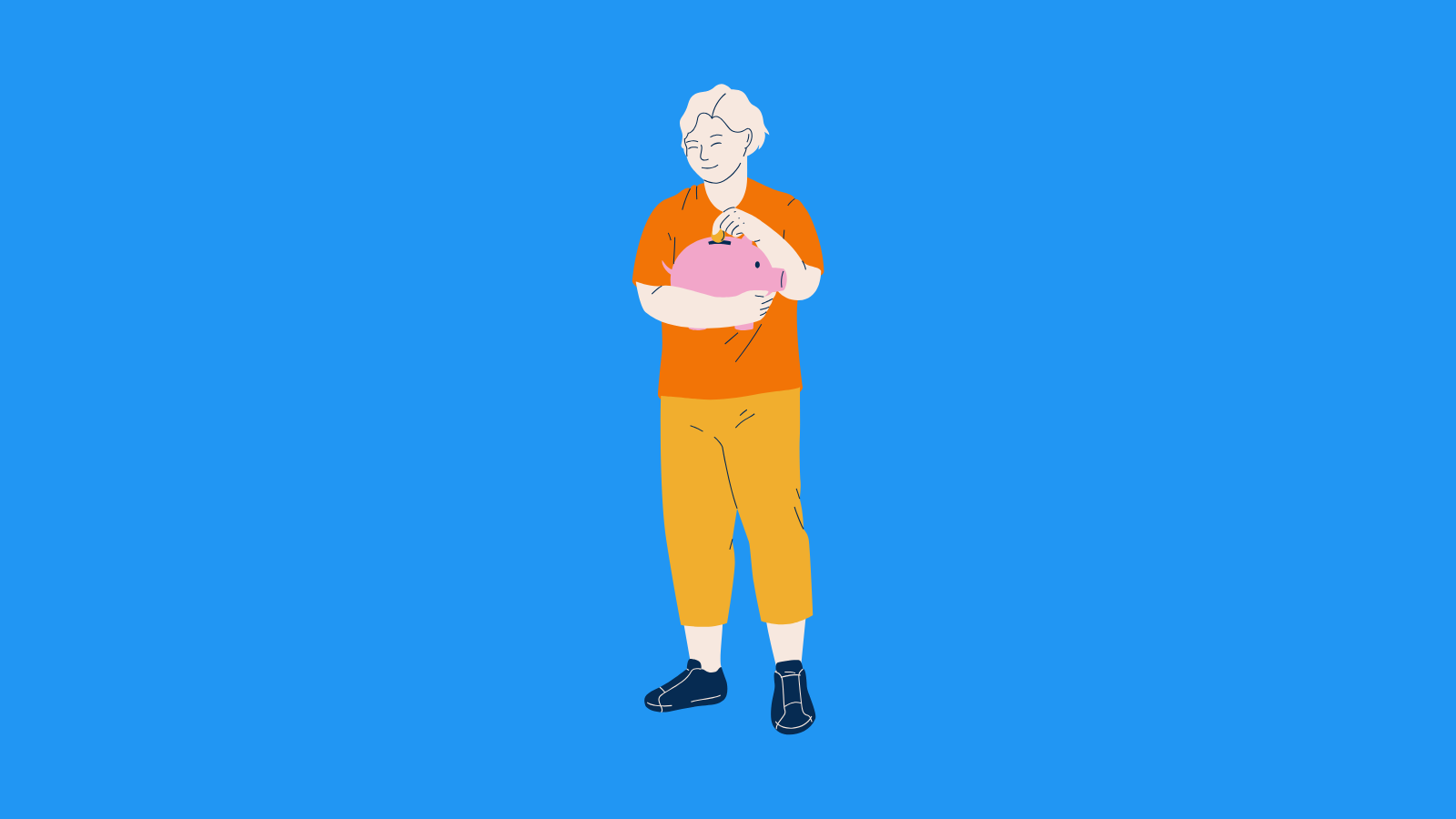
S&P GSCI Gold Index Price Forecasting Model
To predict the future value of the S&P GSCI Gold index, we developed a robust machine learning model. Our approach leverages a comprehensive dataset of historical price data, encompassing various economic indicators including inflation rates, interest rates, global economic growth forecasts, and geopolitical events. This data was pre-processed to handle missing values and outliers, crucial for the model's accuracy. We employed a time series analysis approach incorporating techniques such as ARIMA (Autoregressive Integrated Moving Average) and Exponential Smoothing to capture trends and seasonality within the index. Feature engineering was integral; we extracted lagged values, creating indicators reflecting price momentum, volatility, and market sentiment, to provide a holistic view of the market. Furthermore, we incorporated external factors derived from macroeconomic forecasts and geopolitical risk assessments. This multifaceted approach ensures the model considers both internal and external influencing factors, enhancing predictive accuracy.
The model selection process involved comparing the performance of various regression models, including linear regression, support vector regression, and Gradient Boosting. The Gradient Boosting algorithm, due to its ability to capture complex non-linear relationships, demonstrated the best performance in our initial evaluation. Hyperparameter tuning was performed using techniques such as grid search and randomized search to optimize the model's performance for both training and testing data. We prioritized a model that generalizes well to unseen data, ensuring its reliability in real-world applications. Cross-validation was employed to evaluate the model's stability and robustness across different subsets of the data. To assess the reliability of the prediction, we carefully evaluated the model's residuals, examining for any patterns or anomalies. The results of these assessments were carefully reviewed and refined to ensure the model is robust and resilient to unexpected events in the market.
The final model's accuracy was validated using metrics such as R-squared and Mean Absolute Error. A comprehensive error analysis allowed us to identify potential weaknesses in the model's predictions and areas where improvements could be made. Furthermore, sensitivity analysis was conducted to assess the impact of specific variables on the predicted price of the S&P GSCI Gold index. The model was deployed into a production environment with safeguards and alerts to notify the stakeholders of significant deviations from expected price movements. This model provides a valuable tool for risk management and investment strategy development within the gold market, offering insights into potential future price trends for the S&P GSCI Gold index.
ML Model Testing
n:Time series to forecast
p:Price signals of S&P GSCI Gold index
j:Nash equilibria (Neural Network)
k:Dominated move of S&P GSCI Gold index holders
a:Best response for S&P GSCI Gold target price
For further technical information as per how our model work we invite you to visit the article below:
How do KappaSignal algorithms actually work?
S&P GSCI Gold Index Forecast Strategic Interaction Table
Strategic Interaction Table Legend:
X axis: *Likelihood% (The higher the percentage value, the more likely the event will occur.)
Y axis: *Potential Impact% (The higher the percentage value, the more likely the price will deviate.)
Z axis (Grey to Black): *Technical Analysis%
Rating | Short-Term | Long-Term Senior |
---|---|---|
Outlook | Ba3 | B2 |
Income Statement | B1 | Baa2 |
Balance Sheet | Ba3 | C |
Leverage Ratios | Baa2 | C |
Cash Flow | Caa2 | Caa2 |
Rates of Return and Profitability | Baa2 | Ba3 |
*An aggregate rating for an index summarizes the overall sentiment towards the companies it includes. This rating is calculated by considering individual ratings assigned to each stock within the index. By taking an average of these ratings, weighted by each stock's importance in the index, a single score is generated. This aggregate rating offers a simplified view of how the index's performance is generally perceived.
How does neural network examine financial reports and understand financial state of the company?
References
- A. Tamar, Y. Glassner, and S. Mannor. Policy gradients beyond expectations: Conditional value-at-risk. In AAAI, 2015
- M. J. Hausknecht and P. Stone. Deep recurrent Q-learning for partially observable MDPs. CoRR, abs/1507.06527, 2015
- Doudchenko N, Imbens GW. 2016. Balancing, regression, difference-in-differences and synthetic control methods: a synthesis. NBER Work. Pap. 22791
- Mikolov T, Chen K, Corrado GS, Dean J. 2013a. Efficient estimation of word representations in vector space. arXiv:1301.3781 [cs.CL]
- J. Filar, L. Kallenberg, and H. Lee. Variance-penalized Markov decision processes. Mathematics of Opera- tions Research, 14(1):147–161, 1989
- Kitagawa T, Tetenov A. 2015. Who should be treated? Empirical welfare maximization methods for treatment choice. Tech. Rep., Cent. Microdata Methods Pract., Inst. Fiscal Stud., London
- Mnih A, Kavukcuoglu K. 2013. Learning word embeddings efficiently with noise-contrastive estimation. In Advances in Neural Information Processing Systems, Vol. 26, ed. Z Ghahramani, M Welling, C Cortes, ND Lawrence, KQ Weinberger, pp. 2265–73. San Diego, CA: Neural Inf. Process. Syst. Found.